R-MelNet: Reduced Mel-Spectral Modeling for Neural TTS
arxiv(2022)
摘要
This paper introduces R-MelNet, a two-part autoregressive architecture with a frontend based on the first tier of MelNet and a backend WaveRNN-style audio decoder for neural text-to-speech synthesis. Taking as input a mixed sequence of characters and phonemes, with an optional audio priming sequence, this model produces low-resolution mel-spectral features which are interpolated and used by a WaveRNN decoder to produce an audio waveform. Coupled with half precision training, R-MelNet uses under 11 gigabytes of GPU memory on a single commodity GPU (NVIDIA 2080Ti). We detail a number of critical implementation details for stable half precision training, including an approximate, numerically stable mixture of logistics attention. Using a stochastic, multi-sample per step inference scheme, the resulting model generates highly varied audio, while enabling text and audio based controls to modify output waveforms. Qualitative and quantitative evaluations of an R-MelNet system trained on a single speaker TTS dataset demonstrate the effectiveness of our approach.
更多查看译文
关键词
neural tts,r-melnet,mel-spectral
AI 理解论文
溯源树
样例
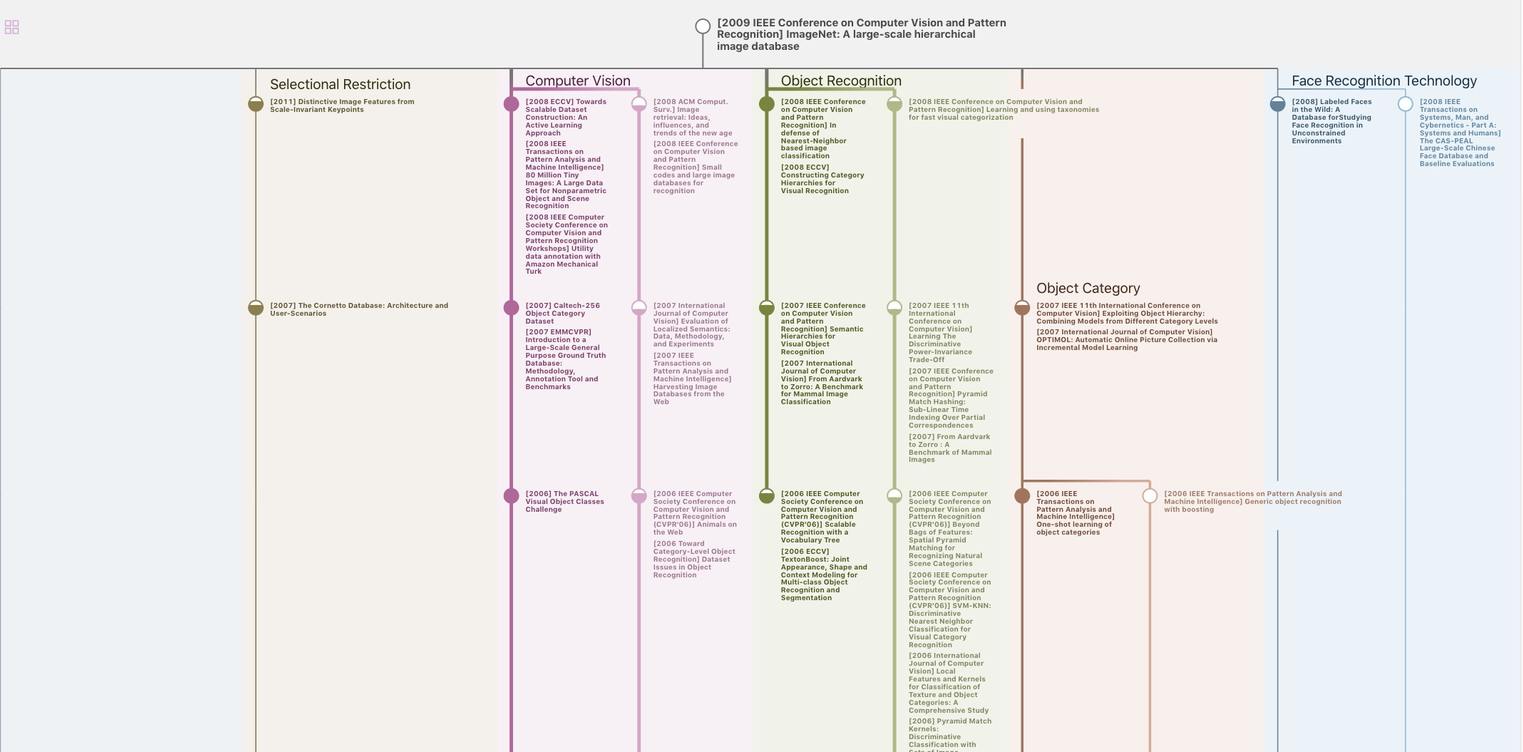
生成溯源树,研究论文发展脉络
Chat Paper
正在生成论文摘要