Learning nonparametric ordinary differential equations from noisy data
CoRR(2023)
摘要
Learning nonparametric systems of Ordinary Differential Equations (ODEs) dot x = f(t,x) from noisy data is an emerging machine learning topic. We use the well-developed theory of Reproducing Kernel Hilbert Spaces (RKHS) to define candidates for f for which the solution of the ODE exists and is unique. Learning f consists of solving a constrained optimization problem in an RKHS. We propose a penalty method that iteratively uses the Representer theorem and Euler approximations to provide a numerical solution. We prove a generalization bound for the L2 distance between x and its estimator and provide experimental comparisons with the state-of-the-art.
更多查看译文
关键词
nonparametric ordinary differential equations,noisy data,learning
AI 理解论文
溯源树
样例
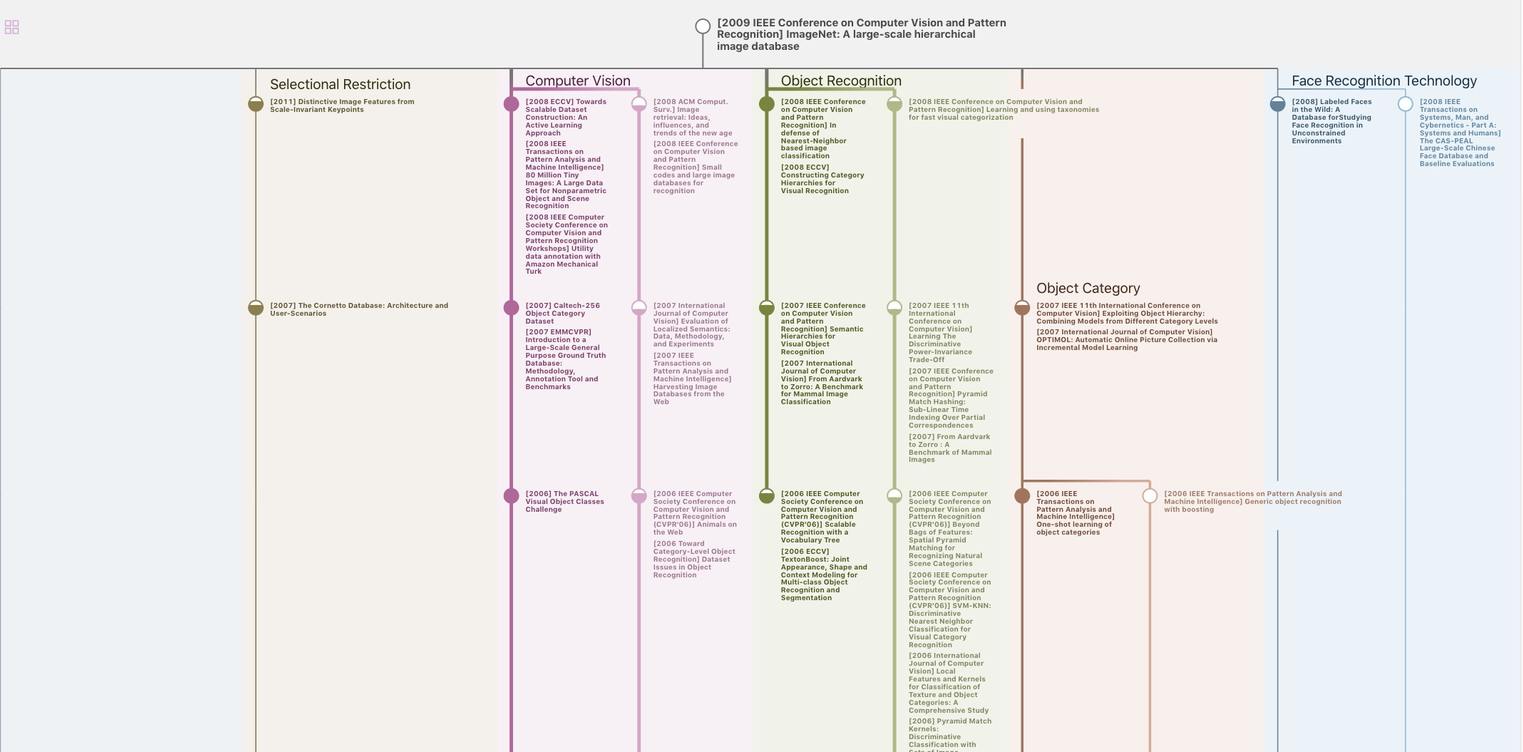
生成溯源树,研究论文发展脉络
Chat Paper
正在生成论文摘要