Out-of-Distribution Detection for Long-Tailed and Fine-Grained Skin Lesion Images
MEDICAL IMAGE COMPUTING AND COMPUTER ASSISTED INTERVENTION, MICCAI 2022, PT I(2022)
摘要
Recent years have witnessed a rapid development of automated methods for skin lesion diagnosis and classification. Due to an increasing deployment of such systems in clinics, it has become important to develop a more robust system towards various Out-of-Distribution (OOD) samples (unknown skin lesions and conditions). However, the current deep learning models trained for skin lesion classification tend to classify these OOD samples incorrectly into one of their learned skin lesion categories. To address this issue, we propose a simple yet strategic approach that improves the OOD detection performance while maintaining the multi-class classification accuracy for the known categories of skin lesion. To specify, this approach is built upon a realistic scenario of a long-tailed and fine-grained OOD detection task for skin lesion images. Through this approach, 1) First, we target the mixup amongst middle and tail classes to address the long-tail problem. 2) Later, we combine the above mixup strategy with prototype learning to address the fine-grained nature of the dataset. The unique contribution of this paper is two-fold, justified by extensive experiments. First, we present a realistic problem setting of OOD task for skin lesion. Second, we propose an approach to target the long-tailed and fine-grained aspects of the problem setting simultaneously to increase the OOD performance.
更多查看译文
关键词
Skin lesion, Out-of-distribution, Openset, Mixup, Prototype
AI 理解论文
溯源树
样例
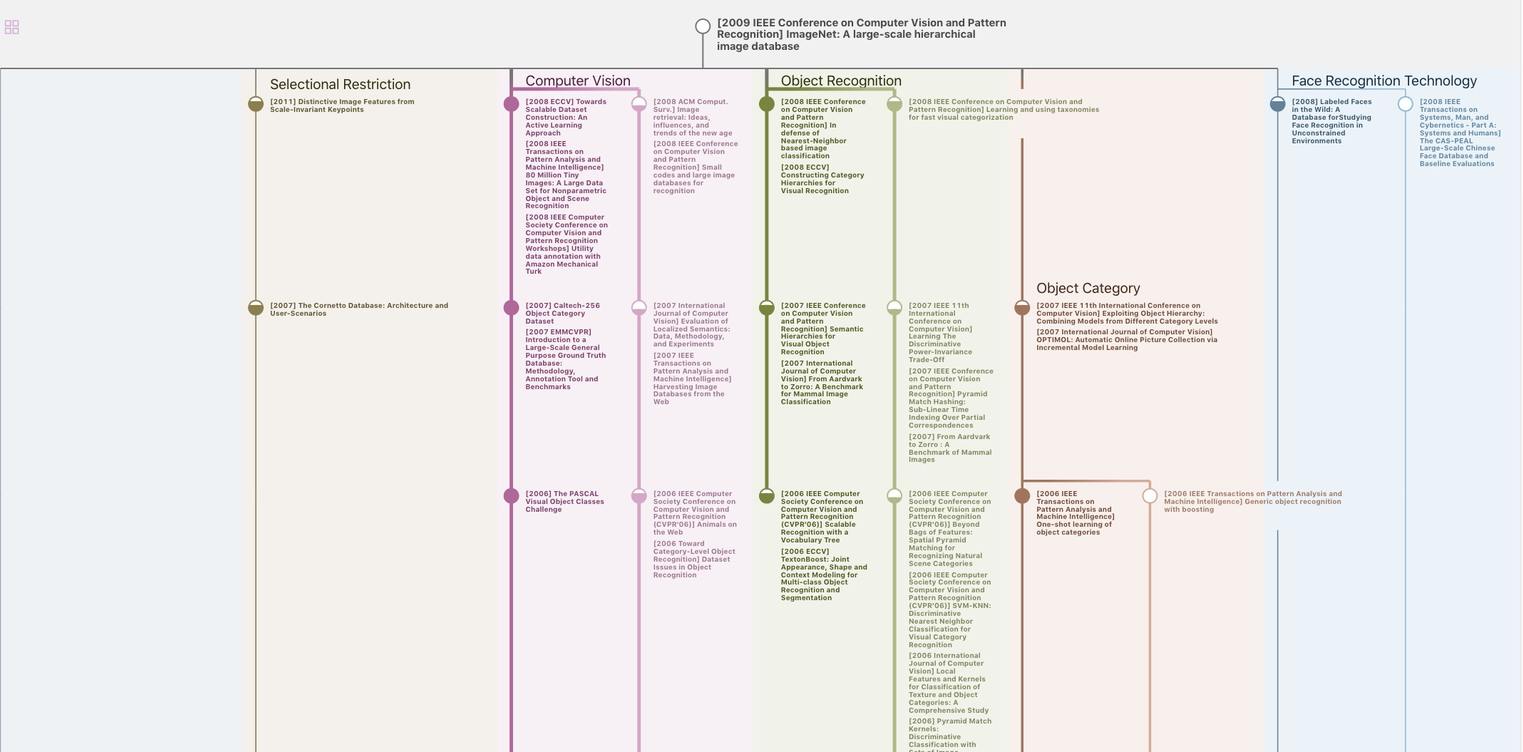
生成溯源树,研究论文发展脉络
Chat Paper
正在生成论文摘要