Efficient Entity Candidate Generation for Low-Resource Languages.
International Conference on Language Resources and Evaluation (LREC)(2022)
摘要
Candidate generation is a crucial module in entity linking. It also plays a key role in multiple NLP tasks that have been proven to beneficially leverage knowledge bases. Nevertheless, it has often been overlooked in the monolingual English entity linking literature, as naive approaches obtain very good performance. Unfortunately, the existing approaches for English cannot be successfully transferred to poorly resourced languages. This paper constitutes an in-depth analysis of the candidate generation problem in the context of cross-lingual entity linking with a focus on low-resource languages. Among other contributions, we point out limitations in the evaluation conducted in previous works. We introduce a characterization of queries into types based on their difficulty, which improves the interpretability of the performance of different methods. We also propose a light-weight and simple solution based on the construction of indexes whose design is motivated by more complex transfer learning based neural approaches. A thorough empirical analysis on 9 real-world datasets under 2 evaluation settings shows that our simple solution outperforms the state-of-the-art approach in terms of both quality and efficiency for almost all datasets and query types.
更多查看译文
关键词
Candidate generation, low-resource languages, evaluation pitfalls, efficiency, light-weight models
AI 理解论文
溯源树
样例
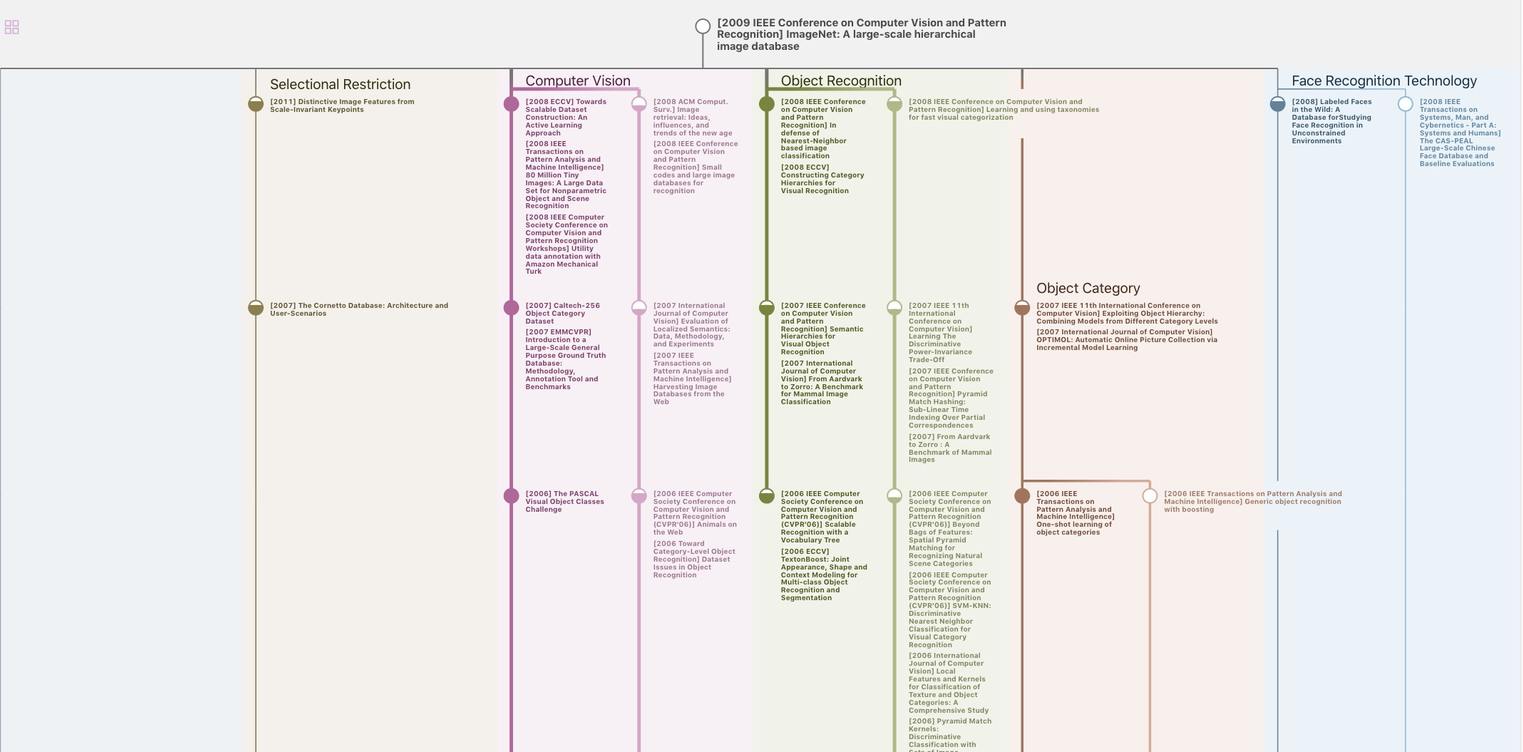
生成溯源树,研究论文发展脉络
Chat Paper
正在生成论文摘要