SRCN3D: Sparse R-CNN 3D Surround-View Camera Object Detection and Tracking for Autonomous Driving
CoRR(2022)
Abstract
Detection and tracking of moving objects (DATMO) is an essential component in environmental perception for autonomous driving. In the flourishing field of multi-view 3D camera-based detectors, different transformer-based pipelines are designed to learn queries in 3D space from 2D feature maps of perspective views, but the dominant dense cross-attention mechanism between queries to values is computationally inefficient. This paper proposes Sparse R-CNN 3D (SRCN3D), a novel two-stage fully-sparse detector with sparse queries, sparse attention and sparse prediction for surround-view camera detection and tracking. SRCN3D adopts a cascade structure with twin-track update of both fixed number of proposal boxes and latent proposal features. Compared to prior arts, our novel sparse feature sampling module only utilizes local 2D region of interest (RoI) features calculated by projection of 3D proposal boxes for further box refinement, leading to an effective, fast and lightweight pipeline. For multi-object tracking, motion features, proposal features and RoI features are comprehensively utilized in multi-hypotheses data association. Extensive experiments on nuScenes dataset demonstrate that SRCN3D achieves competitive performance in object detection and surpasses previous best arts before 2022.08.09 in camera-only multi-object tracking by more than 10 points in terms of AMOTA metric. Code is available at https://github.com/synsin0/SRCN3D.
MoreTranslated text
AI Read Science
Must-Reading Tree
Example
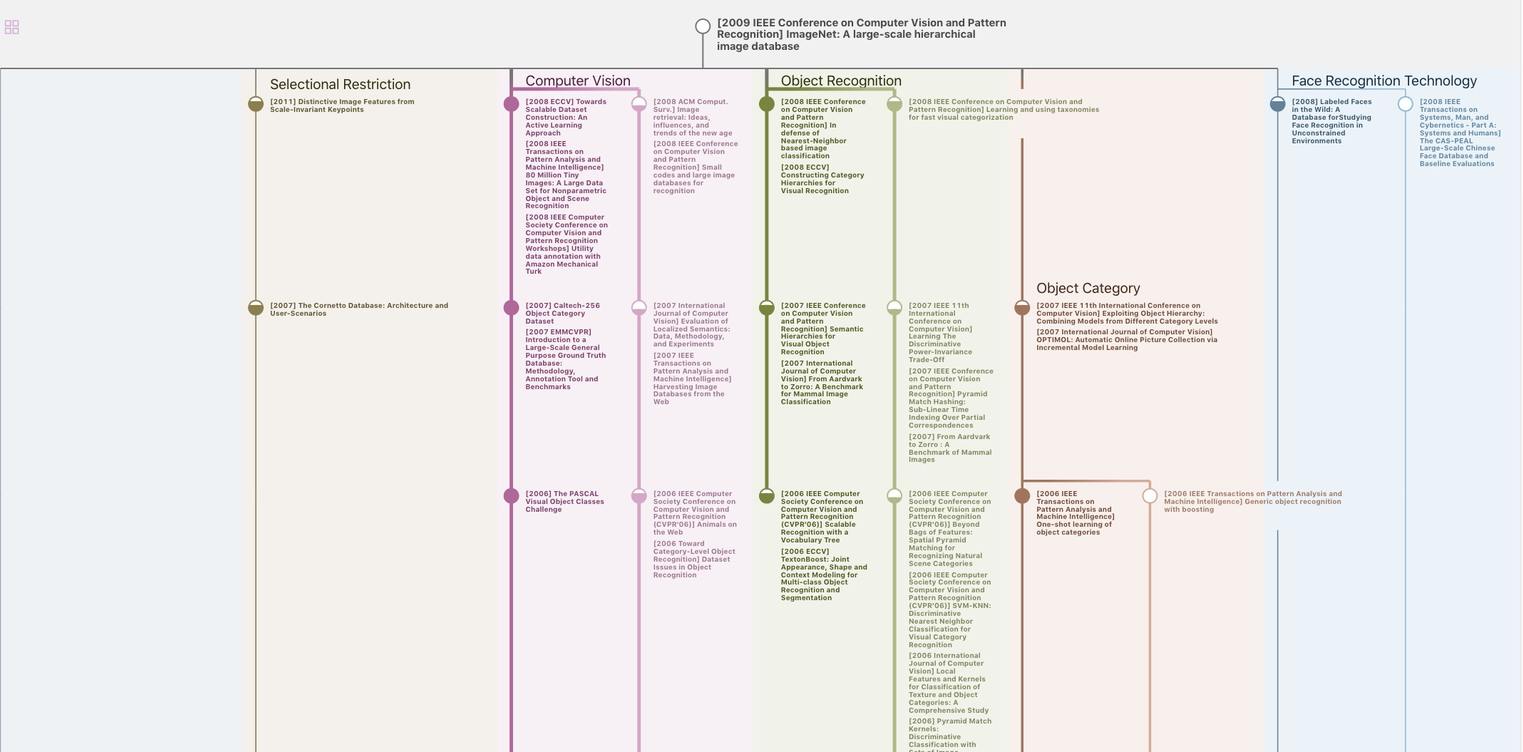
Generate MRT to find the research sequence of this paper
Chat Paper
Summary is being generated by the instructions you defined