Bottleneck Low-rank Transformers for Low-resource Spoken Language Understanding
Conference of the International Speech Communication Association (INTERSPEECH)(2022)
摘要
End-to-end spoken language understanding (SLU) systems benefit from pretraining on large corpora, followed by fine-tuning on application-specific data. The resulting models are too large for on-edge applications. For instance, BERT-based systems contain over 110M parameters. Observing the model is overparameterized, we propose lean transformer structure where the dimension of the attention mechanism is automatically reduced using group sparsity. We propose a variant where the learned attention subspace is transferred to an attention bottleneck layer. In a low-resource setting and without pre-training, the resulting compact SLU model achieves accuracies competitive with pre-trained large models.
更多查看译文
关键词
low-rank transformer, bottleneck attention, lowresource spoken language understanding
AI 理解论文
溯源树
样例
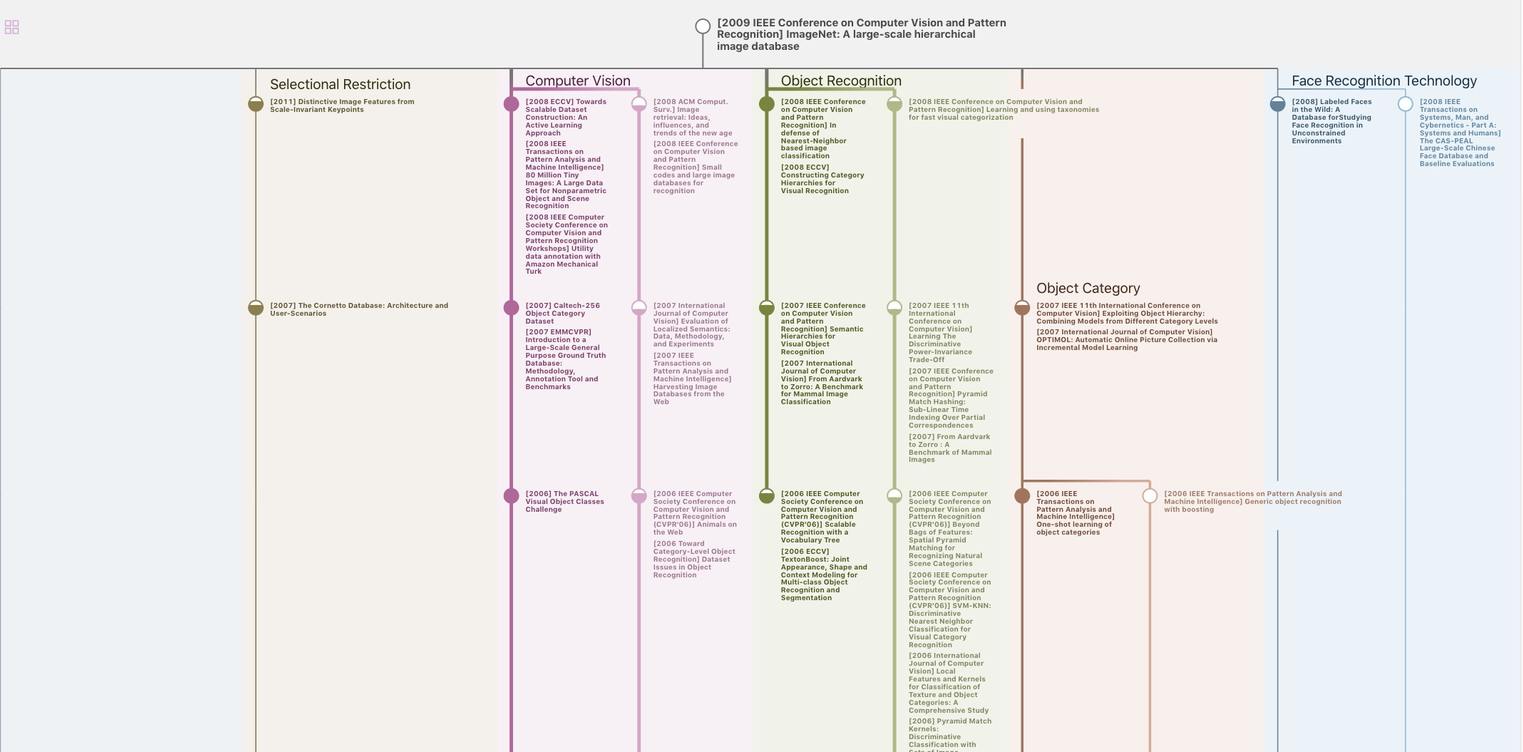
生成溯源树,研究论文发展脉络
Chat Paper
正在生成论文摘要