Learning to Detect 3D Symmetry From Single-View RGB-D Images With Weak Supervision
IEEE Transactions on Pattern Analysis and Machine Intelligence(2023)
摘要
3D symmetry detection is a fundamental problem in computer vision and graphics. Most prior works detect symmetry when the object model is fully known, few studies symmetry detection on objects with partial observation, such as single RGB-D images. Recent work addresses the problem of detecting symmetries from incomplete data with a deep neural network by leveraging the dense and accurate symmetry annotations. However, due to the tedious labeling process, full symmetry annotations are not always practically available. In this work, we present a 3D symmetry detection approach to detect symmetry from single-view RGB-D images without using symmetry supervision. The key idea is to train the network in a weakly-supervised learning manner to complete the shape based on the predicted symmetry such that the completed shape be similar to existing plausible shapes. To achieve this, we first propose a discriminative variational autoencoder to learn the shape prior in order to determine whether a 3D shape is plausible or not. Based on the learned shape prior, a symmetry detection network is present to predict symmetries that produce shapes with high shape plausibility when completed based on those symmetries. Moreover, to facilitate end-to-end network training and multiple symmetry detection, we introduce a new symmetry parametrization for the learning-based symmetry estimation of both reflectional and rotational symmetry. The proposed approach, coupled symmetry detection with shape completion, essentially learns the symmetry-aware shape prior, facilitating more accurate and robust symmetry detection. Experiments demonstrate that the proposed method is capable of detecting reflectional and rotational symmetries accurately, and shows good generality in challenging scenarios, such as objects with heavy occlusion and scanning noise. Moreover, it achieves state-of-the-art performance, improving the F1-score over the existing supervised learning method by 2%-11% on the ShapeNet and ScanNet datasets.
更多查看译文
关键词
Symmetry detection,weakly-supervised learning,RGB-D images,deep neural networks
AI 理解论文
溯源树
样例
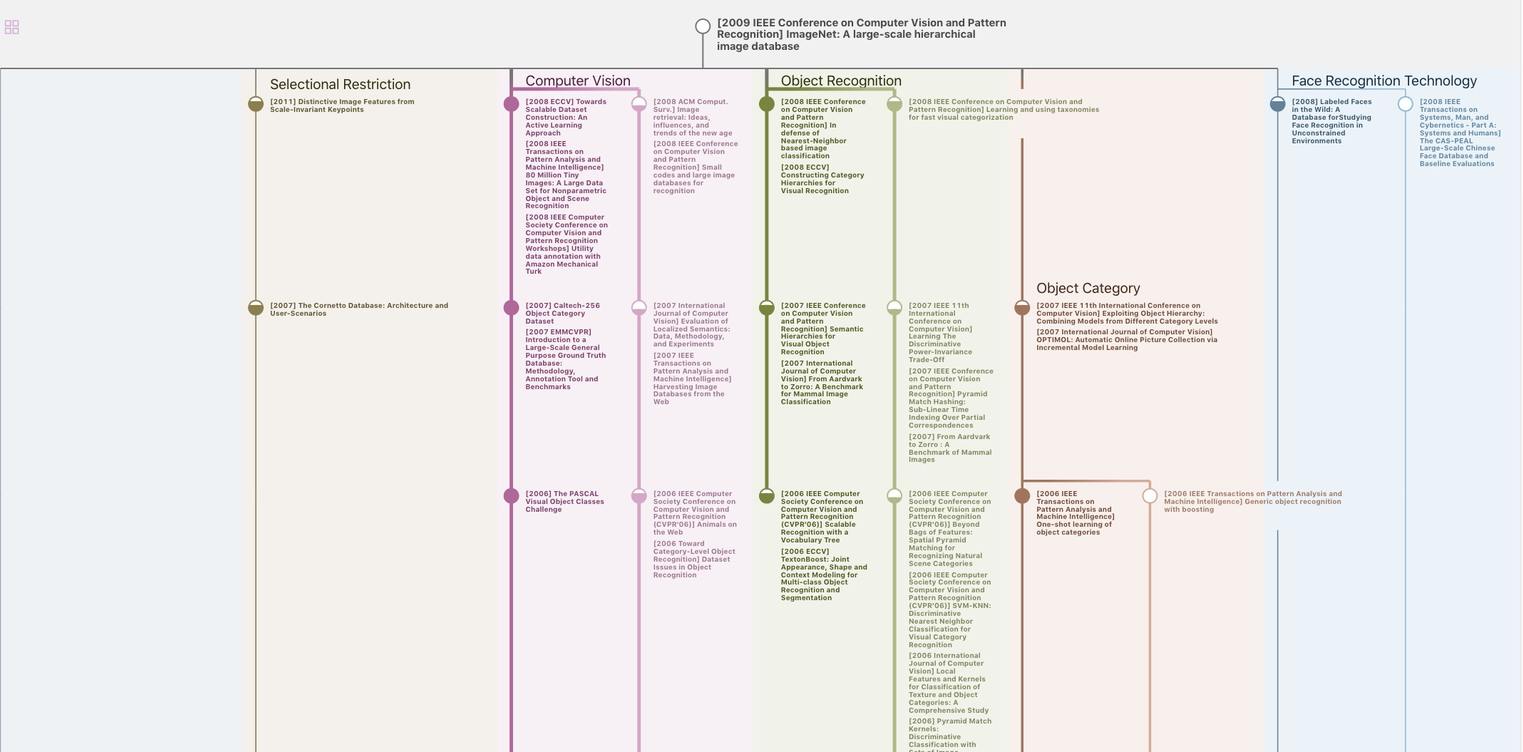
生成溯源树,研究论文发展脉络
Chat Paper
正在生成论文摘要