Classifying high-dimensional Gaussian mixtures: Where kernel methods fail and neural networks succeed
INTERNATIONAL CONFERENCE ON MACHINE LEARNING, VOL 139(2021)
Abstract
A recent series of theoretical works showed that the dynamics of neural networks with a certain initialisation are well-captured by kernel methods. Concurrent empirical work demonstrated that kernel methods can come close to the performance of neural networks on some image classification tasks. These results raise the question of whether neural networks only learn successfully if kernels also learn successfully, despite neural nets being more expressive. Here, we show theoretically that two-layer neural networks (2LNN) with only a few neurons can beat the performance of kernel learning on a simple Gaussian mixture classification task. We study the high-dimensional limit, i.e. when the number of samples is linearly proportional to the dimension, and show that while small 2LNN achieve near-optimal performance on this task, lazy training approaches such as random features and kernel methods do not. Our analysis is based on the derivation of a closed set of equations that track the learning dynamics of the 2LNN and thus allow to extract the asymptotic performance of the network as a function of signal-to-noise ratio and other hyperparameters. We finally illustrate how over-parametrising the neural network leads to faster convergence, but does not improve its final performance.
MoreTranslated text
Key words
kernel methods,neural networks,mixtures,high-dimensional
AI Read Science
Must-Reading Tree
Example
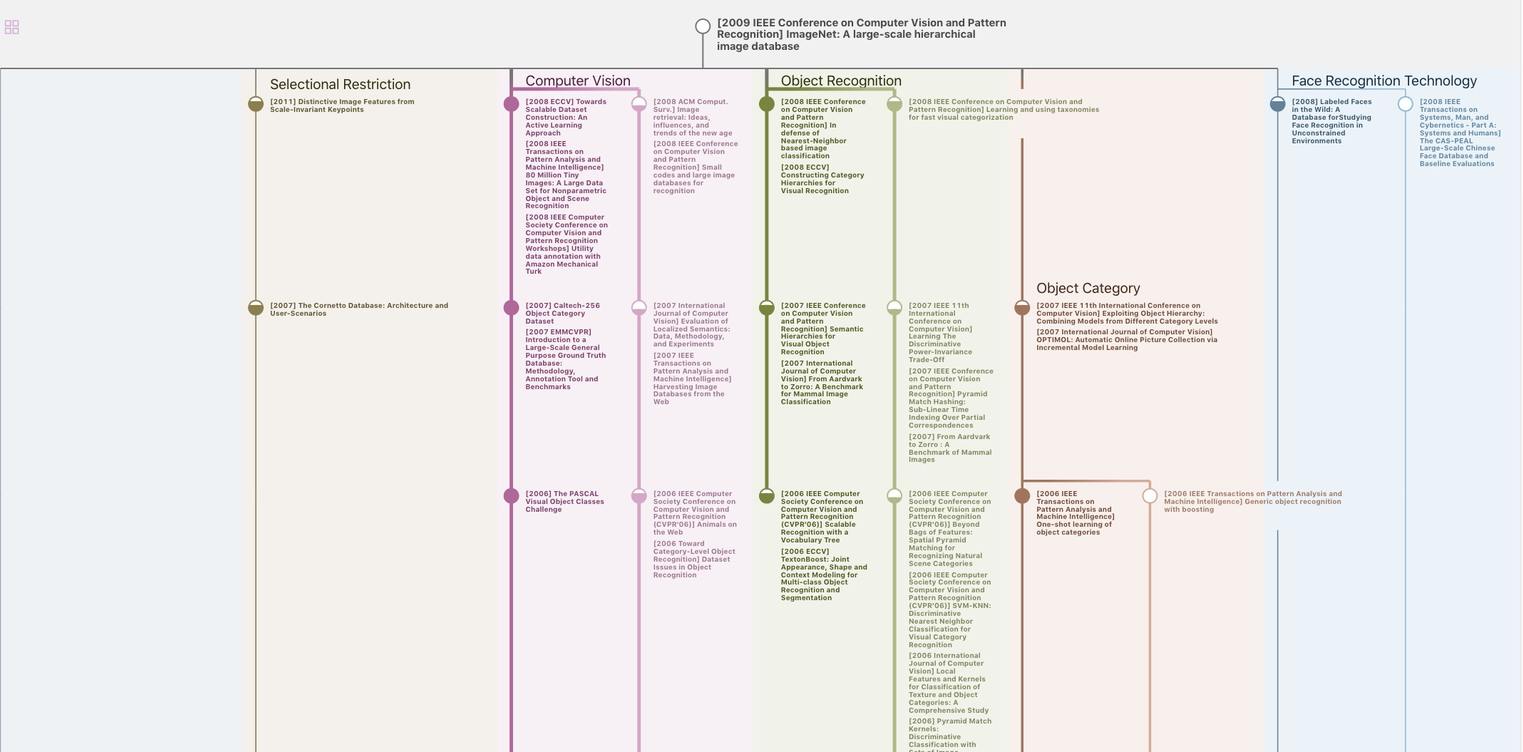
Generate MRT to find the research sequence of this paper
Chat Paper
Summary is being generated by the instructions you defined