Information Entropy Initialized Concrete Autoencoder for Optimal Sensor Placement and Reconstruction of Geophysical Fields
arxiv(2022)
摘要
We propose a new approach to the optimal placement of sensors for the problem of reconstructing geophysical fields from sparse measurements. Our method consists of two stages. In the first stage, we estimate the variability of the physical field as a function of spatial coordinates by approximating its information entropy through the Conditional PixelCNN network. To calculate the entropy, a new ordering of a two-dimensional data array (spiral ordering) is proposed, which makes it possible to obtain the entropy of a physical field simultaneously for several spatial scales. In the second stage, the entropy of the physical field is used to initialize the distribution of optimal sensor locations. This distribution is further optimized with the Concrete Autoencoder architecture with the straight-through gradient estimator and adversarial loss to simultaneously minimize the number of sensors and maximize reconstruction accuracy. Our method scales linearly with data size, unlike commonly used Principal Component Analysis. We demonstrate our method on the two examples: (a) temperature and (b) salinity fields around the Barents Sea and the Svalbard group of islands. For these examples, we compute the reconstruction error of our method and a few baselines. We test our approach against two baselines (1) PCA with QR factorization and (2) climatology. We find out that the obtained optimal sensor locations have clear physical interpretation and correspond to the boundaries between sea currents.
更多查看译文
关键词
concrete autoencoder,optimal sensor placement,entropy
AI 理解论文
溯源树
样例
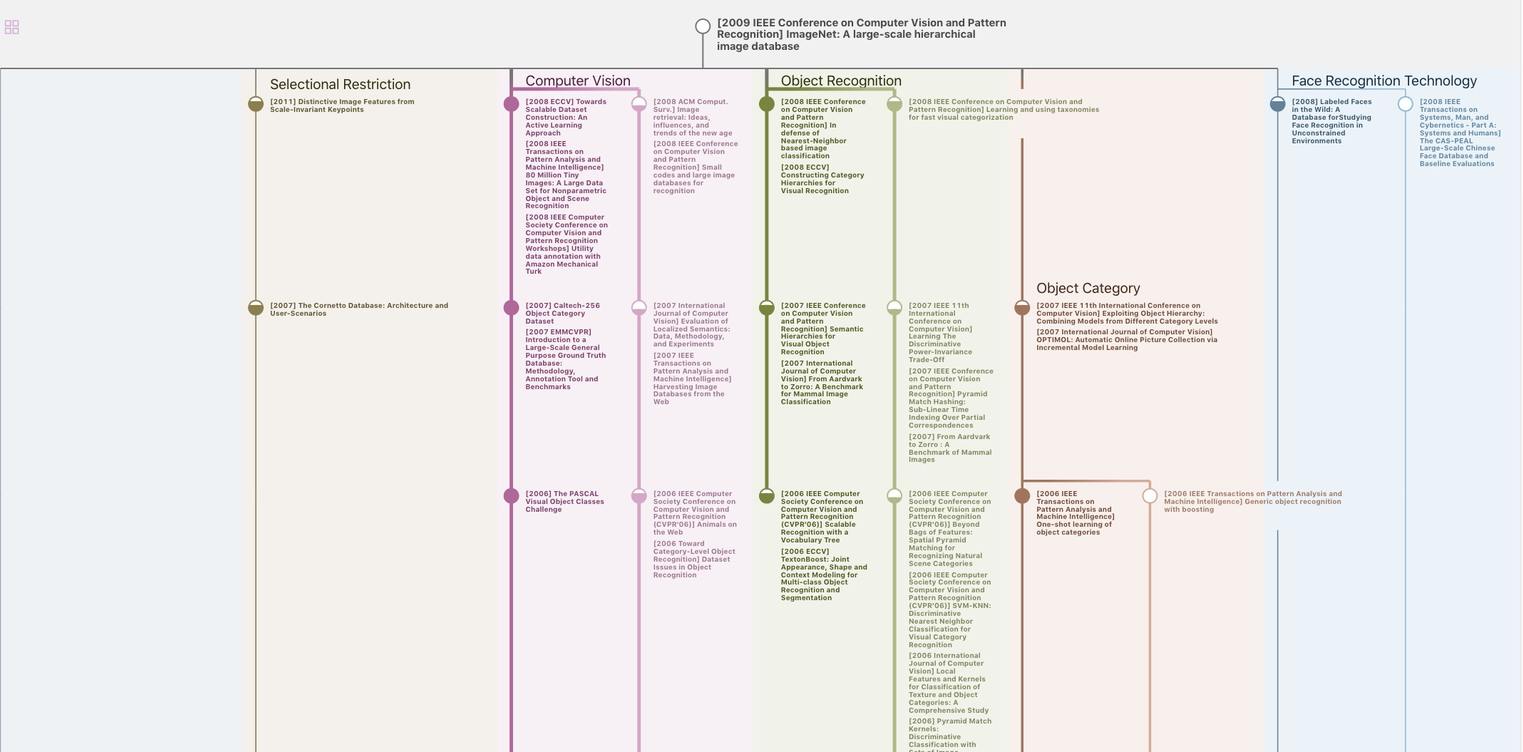
生成溯源树,研究论文发展脉络
Chat Paper
正在生成论文摘要