Dummy Prototypical Networks for Few-Shot Open-Set Keyword Spotting
Conference of the International Speech Communication Association (INTERSPEECH)(2022)
Abstract
Keyword spotting is the task of detecting a keyword in streaming audio. Conventional keyword spotting targets predefined keywords classification, but there is growing attention in few-shot (query-by-example) keyword spotting, e.g., N-way classification given M-shot support samples. Moreover, in real-world scenarios, there can be utterances from unexpected categories (open-set) which need to be rejected rather than classified as one of the N classes. Combining the two needs, we tackle few-shot open-set keyword spotting with a new benchmark setting, named splitGSC. We propose episode-known dummy prototypes based on metric learning to detect an open-set better and introduce a simple and powerful approach, Dummy Prototypical Networks (D-ProtoNets). Our D-ProtoNets shows clear margins compared to recent few-shot open-set recognition (FSOSR) approaches in the suggested splitGSC. We also verify our method on a standard benchmark, miniImageNet, and D-ProtoNets shows the state-of-the-art open-set detection rate in FSOSR.
MoreTranslated text
Key words
networks,few-shot,open-set
AI Read Science
Must-Reading Tree
Example
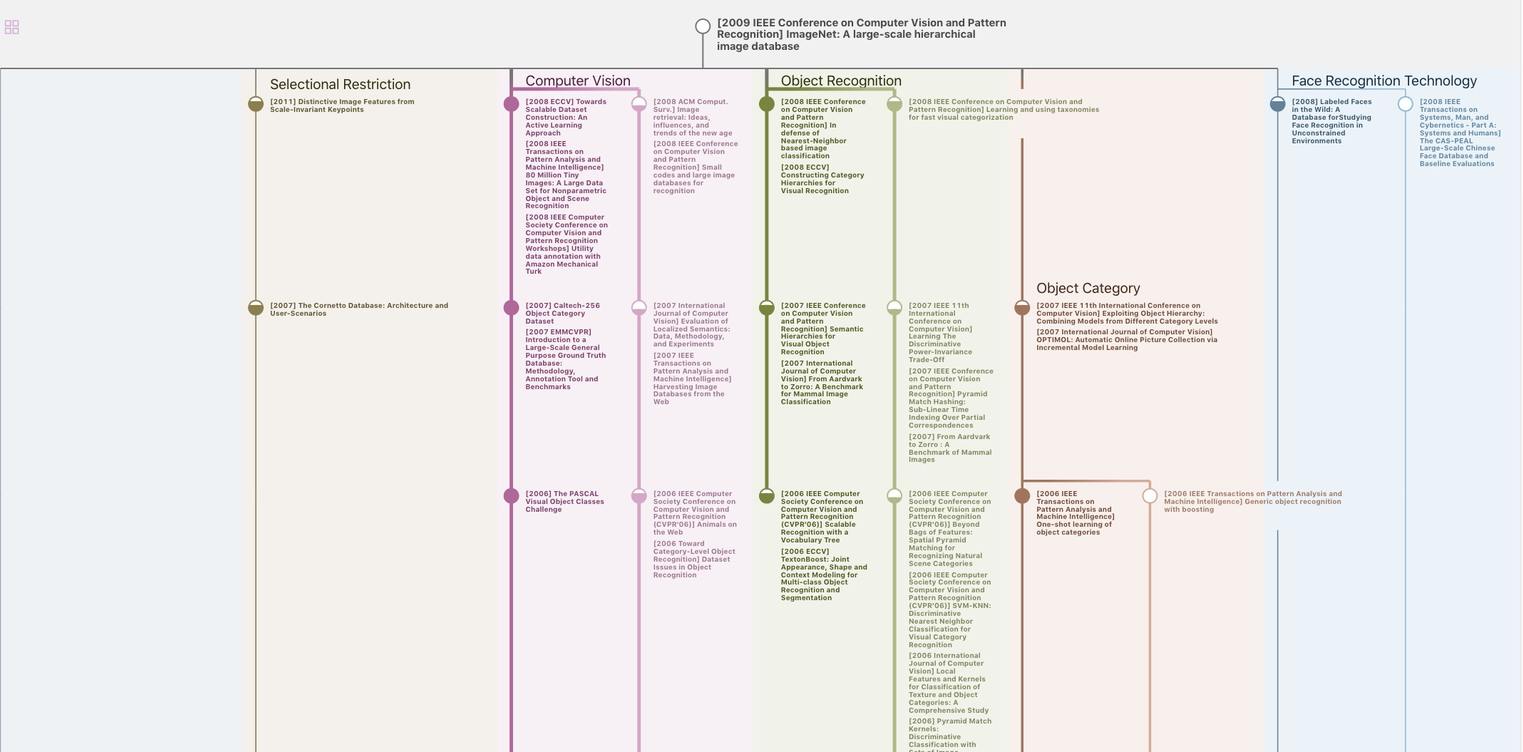
Generate MRT to find the research sequence of this paper
Chat Paper
Summary is being generated by the instructions you defined