Supervised Learning with General Risk Functionals.
International Conference on Machine Learning(2022)
摘要
Standard uniform convergence results bound the generalization gap of the expected loss over a hypothesis class. The emergence of risk-sensitive learning requires generalization guarantees for functionals of the loss distribution beyond the expectation. While prior works specialize in uniform convergence of particular functionals, our work provides uniform convergence for a general class of Hölder risk functionals for which the closeness in the Cumulative Distribution Function (CDF) entails closeness in risk. We establish the first uniform convergence results for estimating the CDF of the loss distribution, which yield uniform convergence guarantees that hold simultaneously both over a class of Hölder risk functionals and over a hypothesis class. Thus licensed to perform empirical risk minimization, we develop practical gradient-based methods for minimizing distortion risks (widely studied subset of Hölder risks that subsumes the spectral risks, including the mean, conditional value at risk, cumulative prospect theory risks, and others) and provide convergence guarantees. In experiments, we demonstrate the efficacy of our learning procedure, both in settings where uniform convergence results hold and in high-dimensional settings with deep networks.
更多查看译文
关键词
general risk functionals,learning
AI 理解论文
溯源树
样例
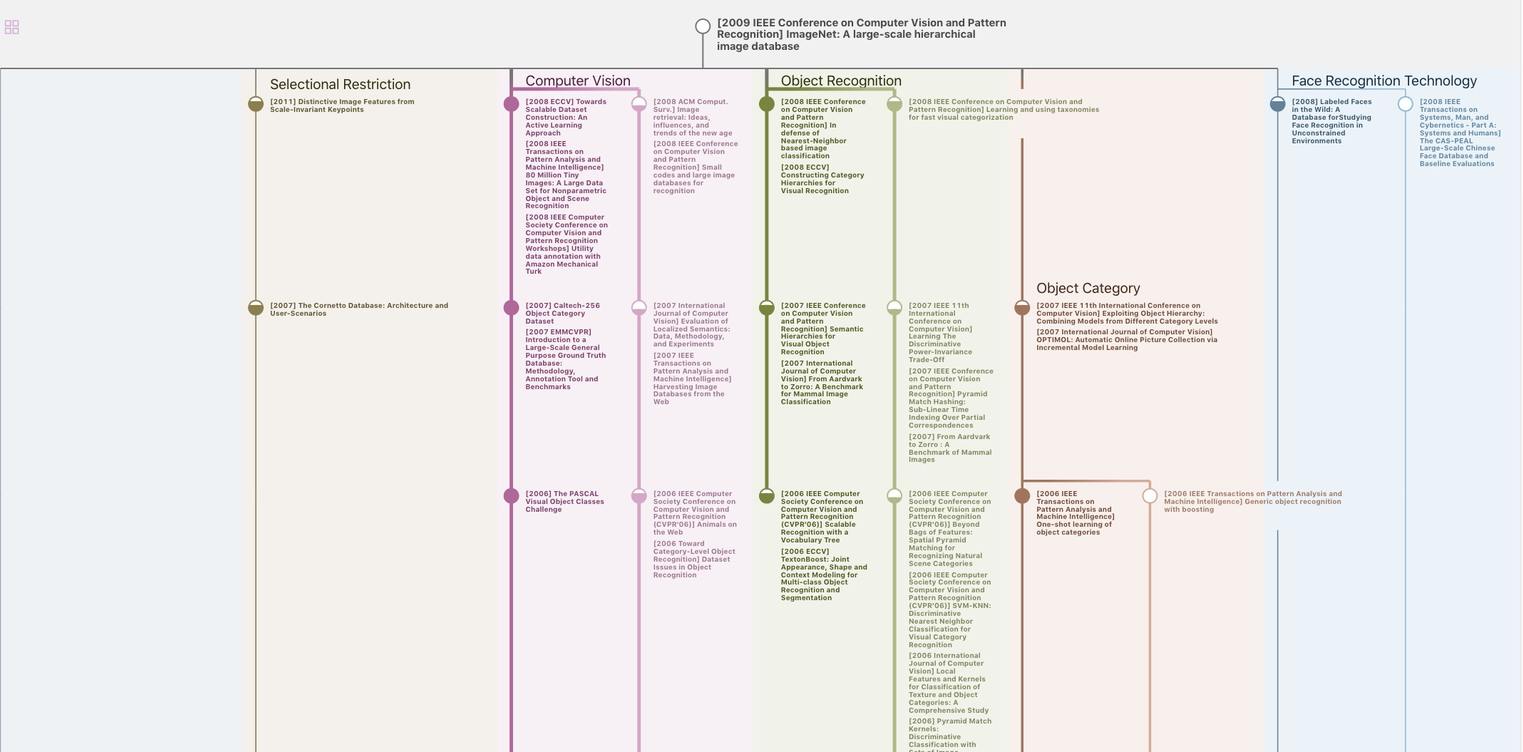
生成溯源树,研究论文发展脉络
Chat Paper
正在生成论文摘要