Three-dimensional prostate CT segmentation through fine-tuning of a pre-trained neural network using no reference labeling
MEDICAL IMAGING 2021: IMAGE-GUIDED PROCEDURES, ROBOTIC INTERVENTIONS, AND MODELING(2021)
摘要
Accurate segmentation of the prostate on computed tomography (CT) has many diagnostic and therapeutic applications. However, manual segmentation is time-consuming and suffers from high inter- and intra-observer variability. Computer-assisted approaches are useful to speed up the process and increase the reproducibility of the segmentation. Deep learning-based segmentation methods have shown potential for quick and accurate segmentation of the prostate on CT images. However, difficulties in obtaining manual, expert segmentations on a large quantity of images limit further progress. Thus, we proposed an approach to train a base model on a small, manually-labeled dataset and fine-tuned the model using unannotated images from a large dataset without any manual segmentation. The datasets used for pre-training and fine-tuning the base model have been acquired in different centers with different CT scanners and imaging parameters. Our fine-tuning method increased the validation and testing Dice scores. A paired, two-tailed t-test shows a significant change in test score (p = 0.017) demonstrating that unannotated images can be used to increase the performance of automated segmentation models.
更多查看译文
关键词
Image segmentation,deep learning,pre-trained neural networks,fine tuning,prostate,computed tomography (CT)
AI 理解论文
溯源树
样例
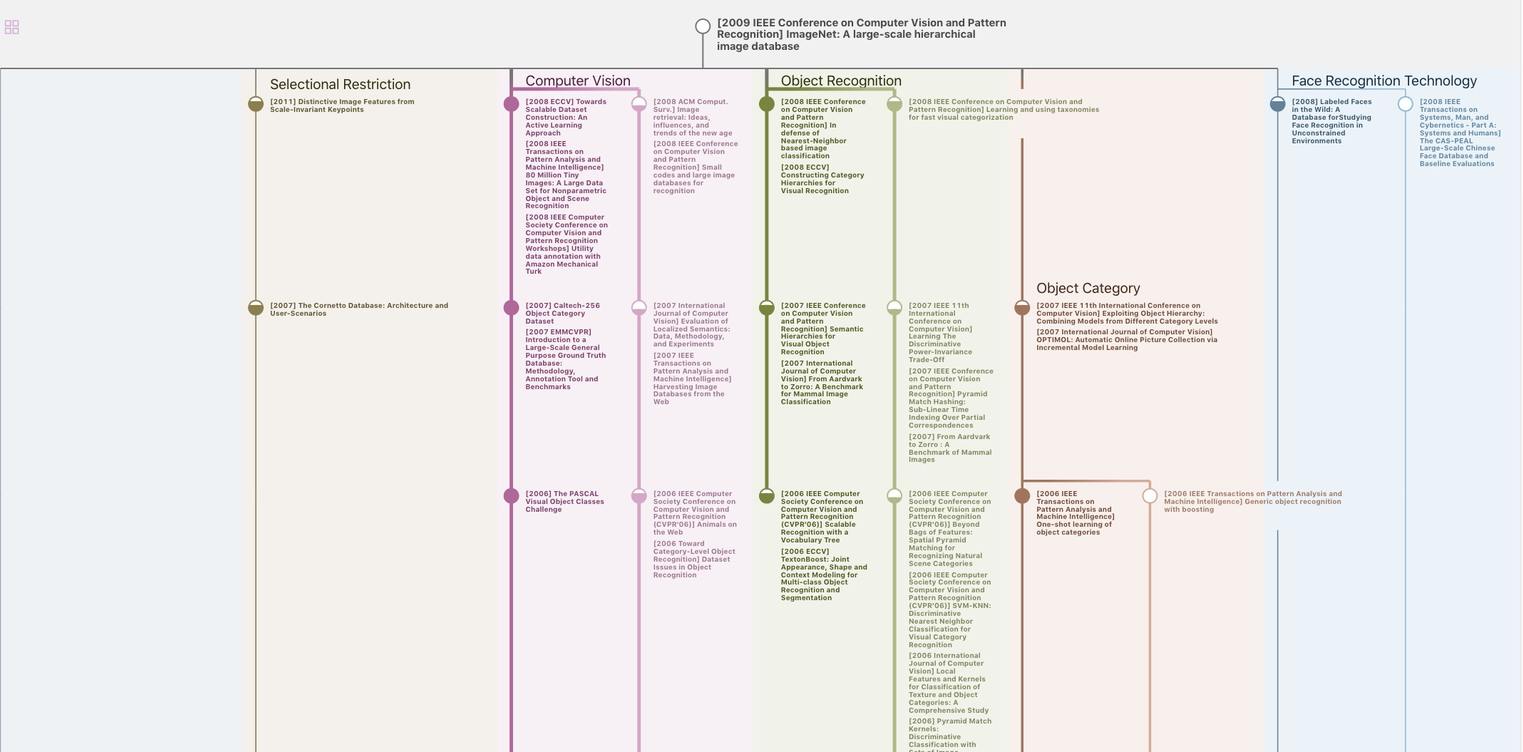
生成溯源树,研究论文发展脉络
Chat Paper
正在生成论文摘要