EMVLight: A multi-agent reinforcement learning framework for an emergency vehicle decentralized routing and traffic signal control system
Transportation Research Part C: Emerging Technologies(2023)
摘要
Emergency vehicles (EMVs) play a crucial role in responding to time-critical calls such as medical emergencies and fire outbreaks in urban areas. Existing methods for EMV dispatch typically optimize routes based on historical traffic-flow data and design traffic signal pre-emption accordingly; however, we still lack a systematic methodology to address the coupling between EMV routing and traffic signal control. In this paper, we propose EMVLight, a decentralized reinforcement learning (RL) framework for joint dynamic EMV routing and traffic signal pre-emption. We adopt the multi-agent advantage actor–critic method with policy sharing and spatial discounted factor. This framework addresses the coupling between EMV navigation and traffic signal control via an innovative design of multi-class RL agents and a novel pressure-based reward function. The proposed methodology enables EMVLight to learn network-level cooperative traffic signal phasing strategies that not only reduce EMV travel time but also shortens the travel time of non-EMVs. Simulation-based experiments indicate that EMVLight enables up to a 42.6% reduction in EMV travel time as well as an 23.5% shorter average travel time compared with existing approaches.
更多查看译文
关键词
Emergency vehicle management,Traffic signal control,Deep reinforcement learning,Multi-agent system
AI 理解论文
溯源树
样例
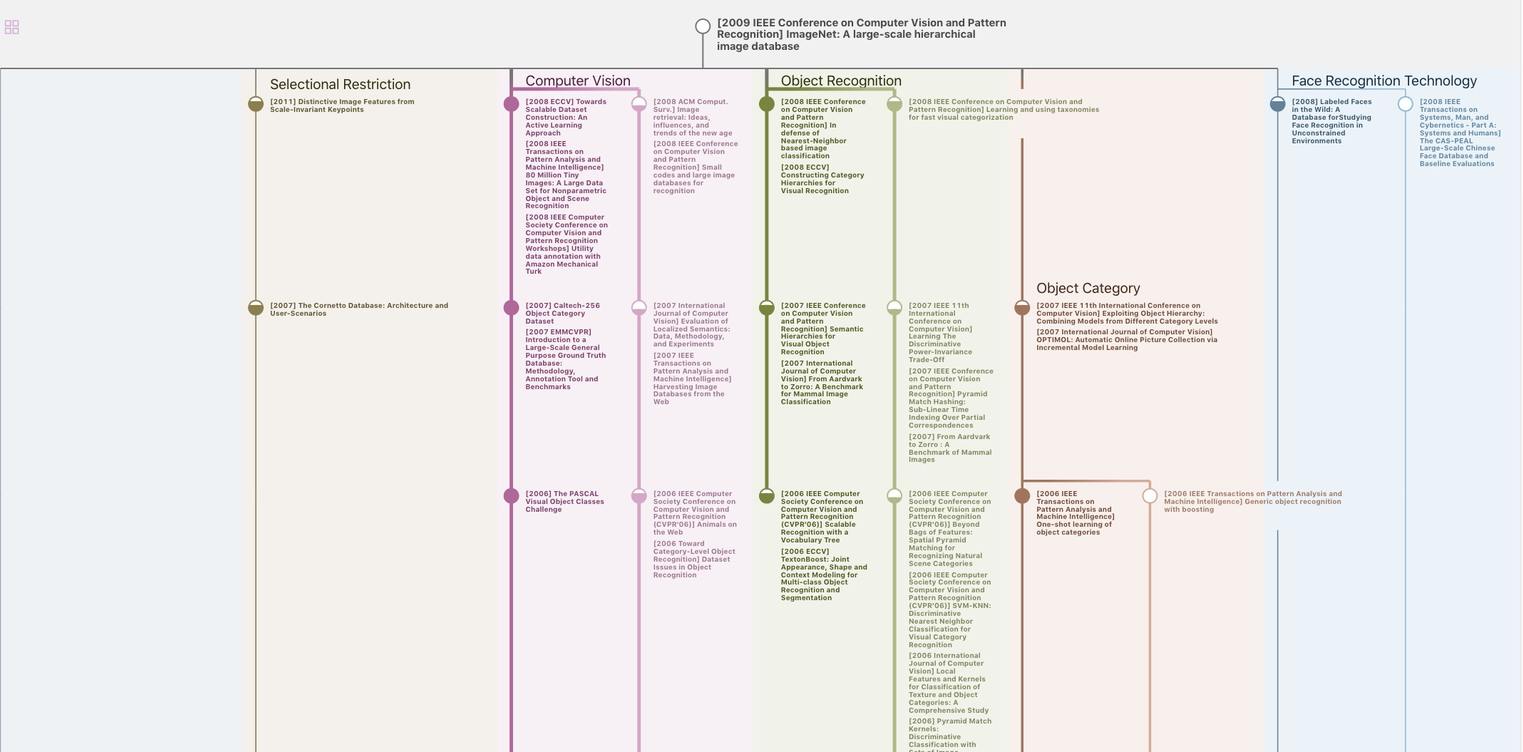
生成溯源树,研究论文发展脉络
Chat Paper
正在生成论文摘要