Uncovering variability in human driving behavior through automatic extraction of similar traffic scenes from large naturalistic datasets
arxiv(2023)
摘要
Recently, multiple naturalistic traffic datasets of human-driven trajectories have been published (e.g., highD, NGSim, and pNEUMA). These datasets have been used in studies that investigate variability in human driving behavior, for example for scenario-based validation of autonomous vehicle (AV) behavior, modeling driver behavior, or validating driver models. Thus far, these studies focused on the variability on an operational level (e.g., velocity profiles during a lane change), not on a tactical level (i.e., to change lanes or not). Investigating the variability on both levels is necessary to develop driver models and AVs that include multiple tactical behaviors. To expose multi-level variability, the human responses to the same traffic scene could be investigated. However, no method exists to automatically extract similar scenes from datasets. Here, we present a four-step extraction method that uses the Hausdorff distance, a mathematical distance metric for sets. We performed a case study on the highD dataset that showed that the method is practically applicable. The human responses to the selected scenes exposed the variability on both the tactical and operational levels. With this new method, the variability in operational and tactical human behavior can be investigated, without the need for costly and time-consuming driving-simulator experiments.
更多查看译文
关键词
Human Behavior,Behavioral Variables,Nature Of The Dataset,Driver Behavior,Humoral Response,Operational Level,Autonomous Vehicles,Lane Change,Hausdorff Distance,Tactical Level,Vehicle Behavior,Driver Model,Tactical Behavior,Response Variables,Positive Difference,Set Point,Lateral Position,Post-processing Step,Operational Variables,Dynamic Elements,Longitudinal Position,Vehicle Identification,Scene Dataset,Dataset ID,Traffic Scenarios,Lane Center,Left Lane
AI 理解论文
溯源树
样例
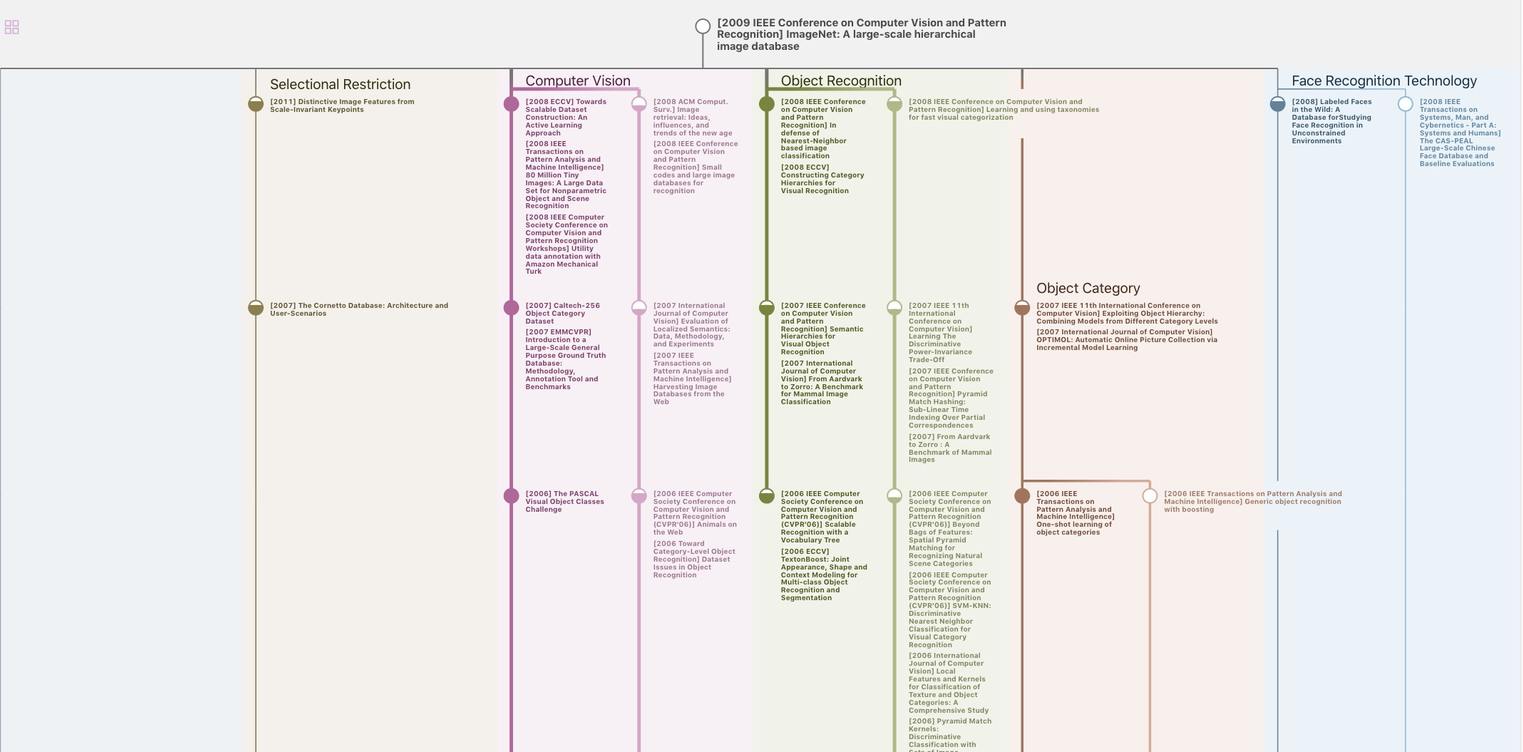
生成溯源树,研究论文发展脉络
Chat Paper
正在生成论文摘要