Pragmatic distributionally robust optimization for simple integer recourse models
arXiv (Cornell University)(2022)
摘要
Inspired by its success for their continuous counterparts, the standard approach to deal with mixed-integer recourse (MIR) models under distributional uncertainty is to use distributionally robust optimization (DRO). We argue, however, that this modeling choice is not always justified, since DRO techniques are generally computationally extremely challenging when integer decision variables are involved. That is why we propose a fundamentally different approach for MIR models under distributional uncertainty aimed at obtaining models with improved computational tractability. For the special case of simple integer recourse (SIR) models, we show that tractable models can be obtained by pragmatically selecting the uncertainty set. Here, we consider uncertainty sets based on the Wasserstein distance and also on generalized moment conditions. We compare our approach with standard DRO and discuss potential generalizations of our ideas to more general MIR models. An important side-result of our analysis is the derivation of performance guarantees for convex approximations of SIR models. In contrast with the literature, these error bounds are not only valid for continuous distribution, but hold for any distribution.
更多查看译文
AI 理解论文
溯源树
样例
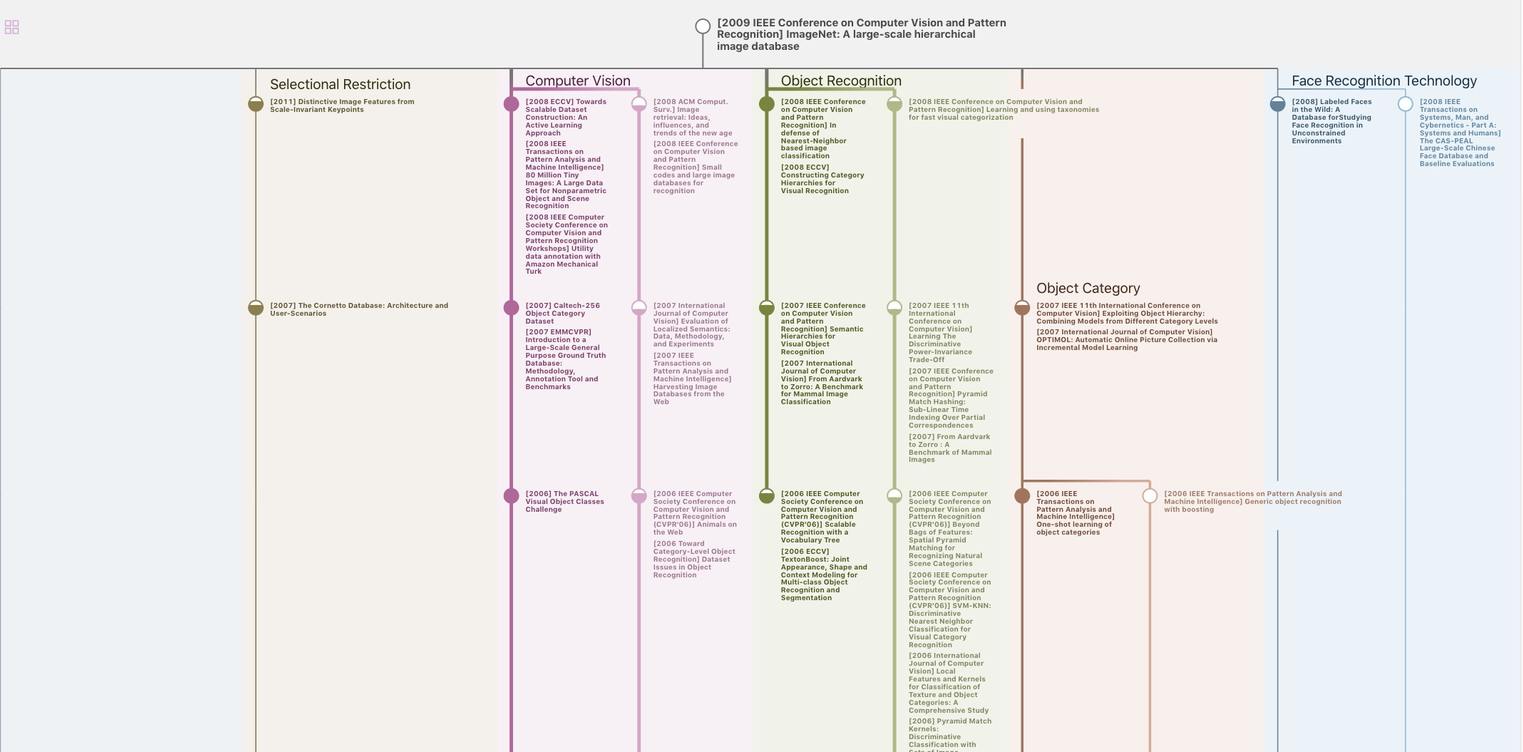
生成溯源树,研究论文发展脉络
Chat Paper
正在生成论文摘要