Dynamic-group-aware networks for multi-agent trajectory prediction with relational reasoning
NEURAL NETWORKS(2024)
摘要
Demystifying the interactions among multiple agents from their past trajectories is fundamental to precise and interpretable trajectory prediction. However, previous works mainly consider static, pairwise interactions with limited relational reasoning. To more comprehensively model interactions and reason relations, we propose DynGroupNet, a dynamic-group-aware network, which (i) models time-varying interactions in highly dynamic scenes; (ii) captures both pairwise and group-wise interactions; and (iii) reasons both interaction strength and category without direct supervision. Based on DynGroupNet, we further design a prediction system to forecast socially plausible trajectories with dynamic relational reasoning. The proposed prediction system leverages the Gaussian mixture model, multiple sampling and prediction refinement to promote prediction diversity for multiple future possibilities capturing, training stability for efficient model learning and trajectory smoothness for more realistic predictions, respectively. The proposed complex interaction modeling of DynGroupNet, future diversity capturing, efficient model training and trajectory smoothing of prediction system together to promote more accurate and plausible future predictions. Extensive experiments show that: (1) DynGroupNet can capture time-varying group behaviors, infer time-varying interaction category and interaction strength during prediction; (2) DynGroupNet significantly outperforms the state-of-the-art trajectory prediction methods by 28.0%, 34.9%, 13.0% in FDE on the NBA, NFL and SDD datasets.
更多查看译文
关键词
Multi-agent trajectory prediction,Relational reasoning,Interaction modeling,Dynamic multiscale hypergraph
AI 理解论文
溯源树
样例
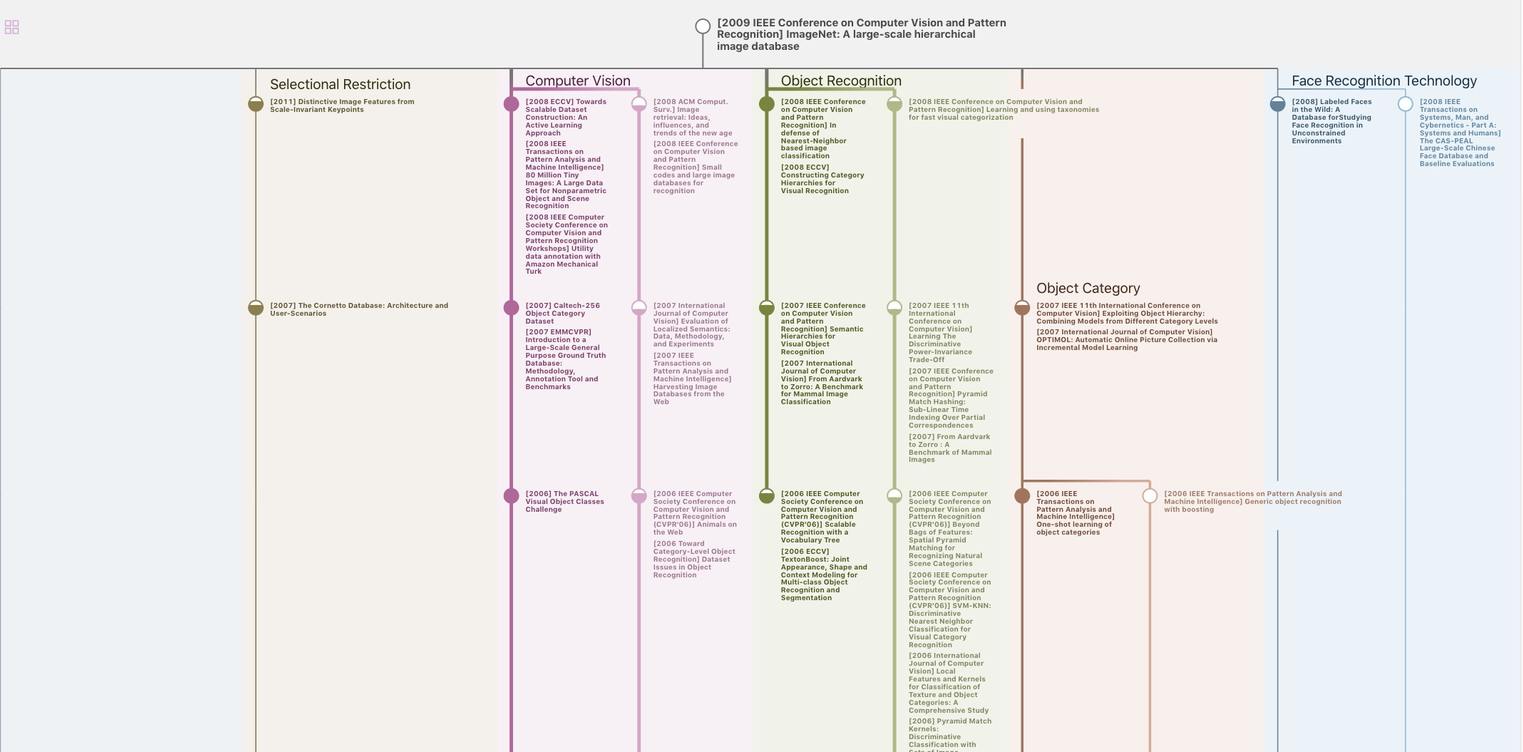
生成溯源树,研究论文发展脉络
Chat Paper
正在生成论文摘要