Uncertainty Calibration for Deep Audio Classifiers.
Conference of the International Speech Communication Association (INTERSPEECH)(2022)
摘要
Although deep Neural Networks (DNNs) have achieved tremendous success in audio classification tasks, their uncertainty calibration are still under-explored. A well-calibrated model should be accurate when it is certain about its prediction and indicate high uncertainty when it is likely to be inaccurate. In this work, we investigate the uncertainty calibration for deep audio classifiers. In particular, we empirically study the performance of popular calibration methods: (i) Monte Carlo Dropout, (ii) ensemble, (iii) focal loss, and (iv) spectral-normalized Gaussian process (SNGP), on audio classification datasets. To this end, we evaluate (i-iv) for the tasks of environment sound and music genre classification. Results indicate that uncalibrated deep audio classifiers may be over-confident, and SNGP performs the best and is very efficient on the two datasets of this paper.
更多查看译文
关键词
uncertainty
AI 理解论文
溯源树
样例
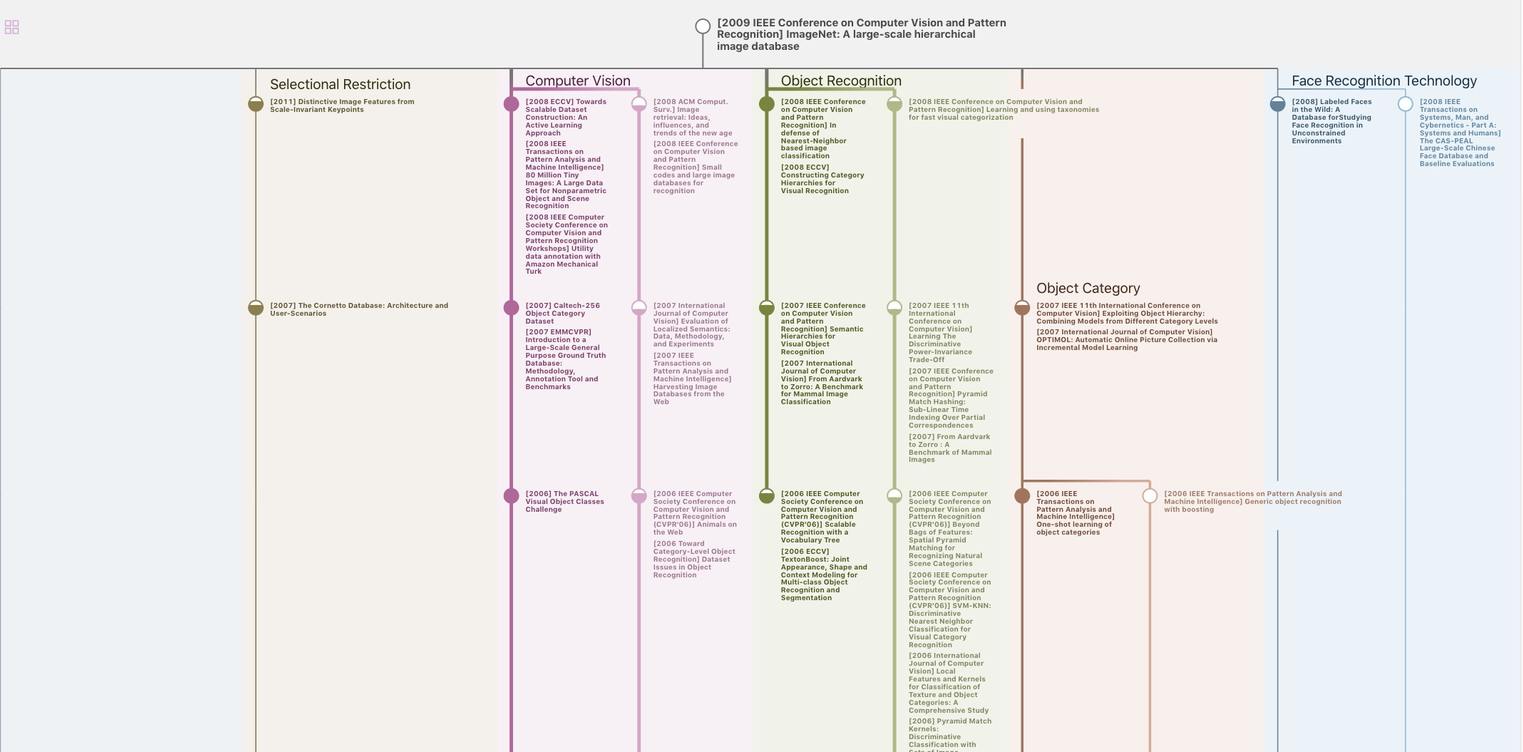
生成溯源树,研究论文发展脉络
Chat Paper
正在生成论文摘要