Learning Rhetorical Structure Theory-based descriptions of observed behaviour
CoRR(2022)
摘要
In a previous paper, we have proposed a set of concepts, axiom schemata and algorithms that can be used by agents to learn to describe their behaviour, goals, capabilities, and environment. The current paper proposes a new set of concepts, axiom schemata and algorithms that allow the agent to learn new descriptions of an observed behaviour (e.g., perplexing actions), of its actor (e.g., undesired propositions or actions), and of its environment (e.g., incompatible propositions). Each learned description (e.g., a certain action prevents another action from being performed in the future) is represented by a relationship between entities (either propositions or actions) and is learned by the agent, just by observation, using domain-independent axiom schemata and or learning algorithms. The relations used by agents to represent the descriptions they learn were inspired on the Theory of Rhetorical Structure (RST). The main contribution of the paper is the relation family Although, inspired on the RST relation Concession. The accurate definition of the relations of the family Although involves a set of deontic concepts whose definition and corresponding algorithms are presented. The relations of the family Although, once extracted from the agent's observations, express surprise at the observed behaviour and, in certain circumstances, present a justification for it. The paper shows results of the presented proposals in a demonstration scenario, using implemented software.
更多查看译文
关键词
behaviour,structure,learning,descriptions,theory-based
AI 理解论文
溯源树
样例
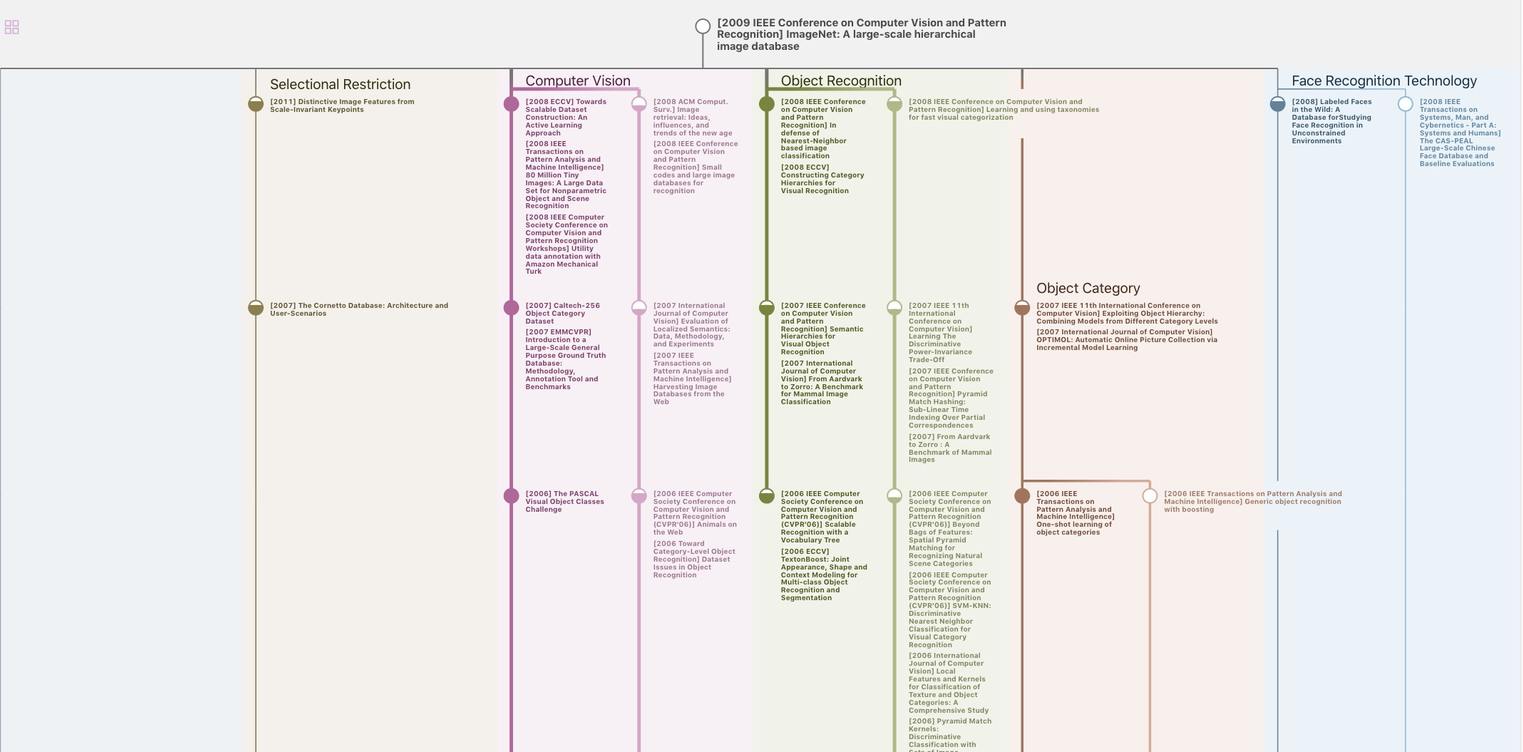
生成溯源树,研究论文发展脉络
Chat Paper
正在生成论文摘要