"You Can't Fix What You Can't Measure": Privately Measuring Demographic Performance Disparities in Federated Learning
AFCP(2023)
摘要
As in traditional machine learning models, models trained with federated learning may exhibit disparate performance across demographic groups. Model holders must identify these disparities to mitigate undue harm to the groups. However, measuring a model's performance in a group requires access to information about group membership which, for privacy reasons, often has limited availability. We propose novel locally differentially private mechanisms to measure differences in performance across groups while protecting the privacy of group membership. To analyze the effectiveness of the mechanisms, we bound their error in estimating a disparity when optimized for a given privacy budget. Our results show that the error rapidly decreases for realistic numbers of participating clients, demonstrating that, contrary to what prior work suggested, protecting privacy is not necessarily in conflict with identifying performance disparities of federated models.
更多查看译文
关键词
federated learning,performance disparities,demographic
AI 理解论文
溯源树
样例
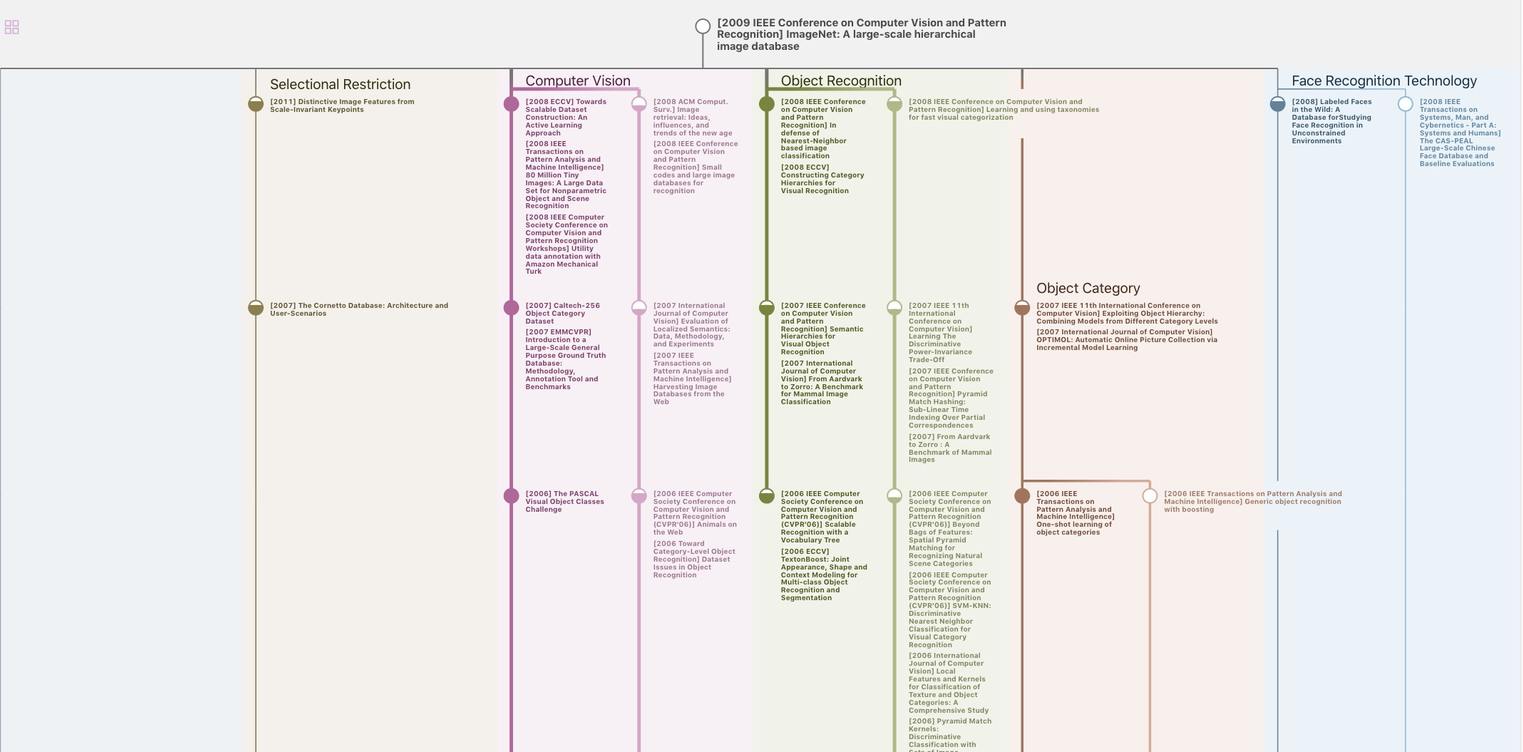
生成溯源树,研究论文发展脉络
Chat Paper
正在生成论文摘要