Unified BERT for Few-shot Natural Language Understanding
arxiv(2022)
摘要
Even as pre-trained language models share a semantic encoder, natural language understanding suffers from a diversity of output schemas. In this paper, we propose UBERT, a unified bidirectional language understanding model based on BERT framework, which can universally model the training objects of different NLU tasks through a biaffine network. Specifically, UBERT encodes prior knowledge from various aspects, uniformly constructing learning representations across multiple NLU tasks, which is conducive to enhancing the ability to capture common semantic understanding. By using the biaffine to model scores pair of the start and end position of the original text, various classification and extraction structures can be converted into a universal, span-decoding approach. Experiments show that UBERT wins the first price in the 2022 AIWIN - World Artificial Intelligence Innovation Competition, Chinese insurance few-shot multi-task track, and realizes the unification of extensive information extraction and linguistic reasoning tasks.
更多查看译文
关键词
unified bert,natural language understanding,few-shot
AI 理解论文
溯源树
样例
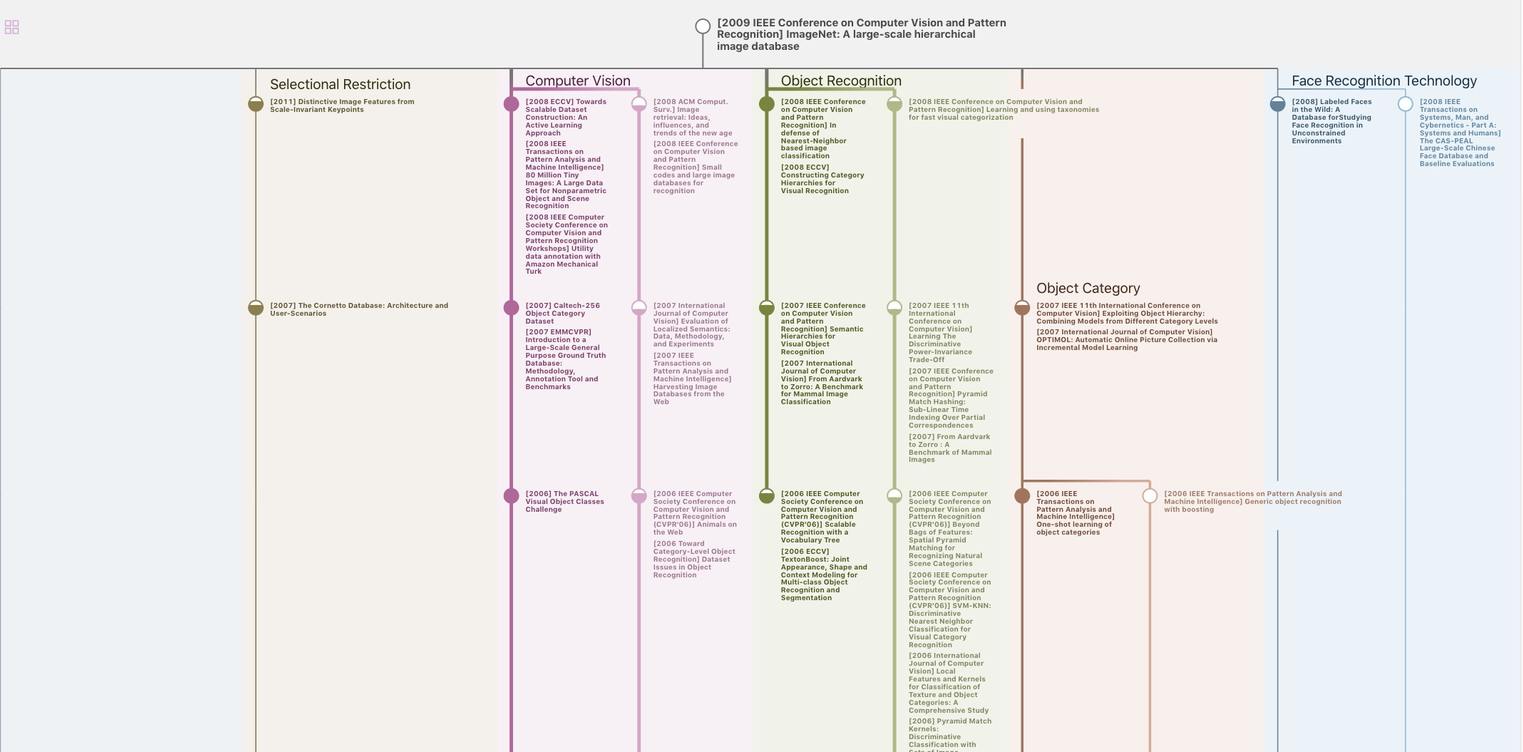
生成溯源树,研究论文发展脉络
Chat Paper
正在生成论文摘要