Research on the membrane fouling diagnosis of MBR membrane module based on ECA-CNN
Journal of Environmental Chemical Engineering(2022)
Abstract
In order to make it easier to extract fault features, reduce model complexity and improve the accuracy of membrane fouling diagnosis on membrane modules, a membrane module fault diagnosis method based on attention mechanism and convolutional neural network (ECA-CNN) is proposed in this study. First, the convolution kernel is used to extract the image features of the input layer. At the same time, a rectified linear unit (ReLU) is connected after each convolution layer, and a batch normalization layer (BN) is added to solve the problem of internal co variate shift, so as improving the expression ability of nonlinear models. Secondly, in batches after the first layer, add the attention mechanism module (ECA), extract the important features and connect to the pooling layer, reduce the network calculation complexity, and improve the accuracy and efficiency of the network. Finally, the membrane module operating data is used as the research object to conduct fault diagnosis experiments as verification. This method can improve the diagnosis accuracy to a large extent, in which the highly difficult fault classification and localization can be accomplished. Besides, the effluent quality from the membrane water treatment system may be improved with this method with less energy consumption, paving the theoretical foundation for actual production.
MoreTranslated text
Key words
Membrane fouling,Convolutional neural network,Attention mechanism,Feature extraction,Fault diagnosis
AI Read Science
Must-Reading Tree
Example
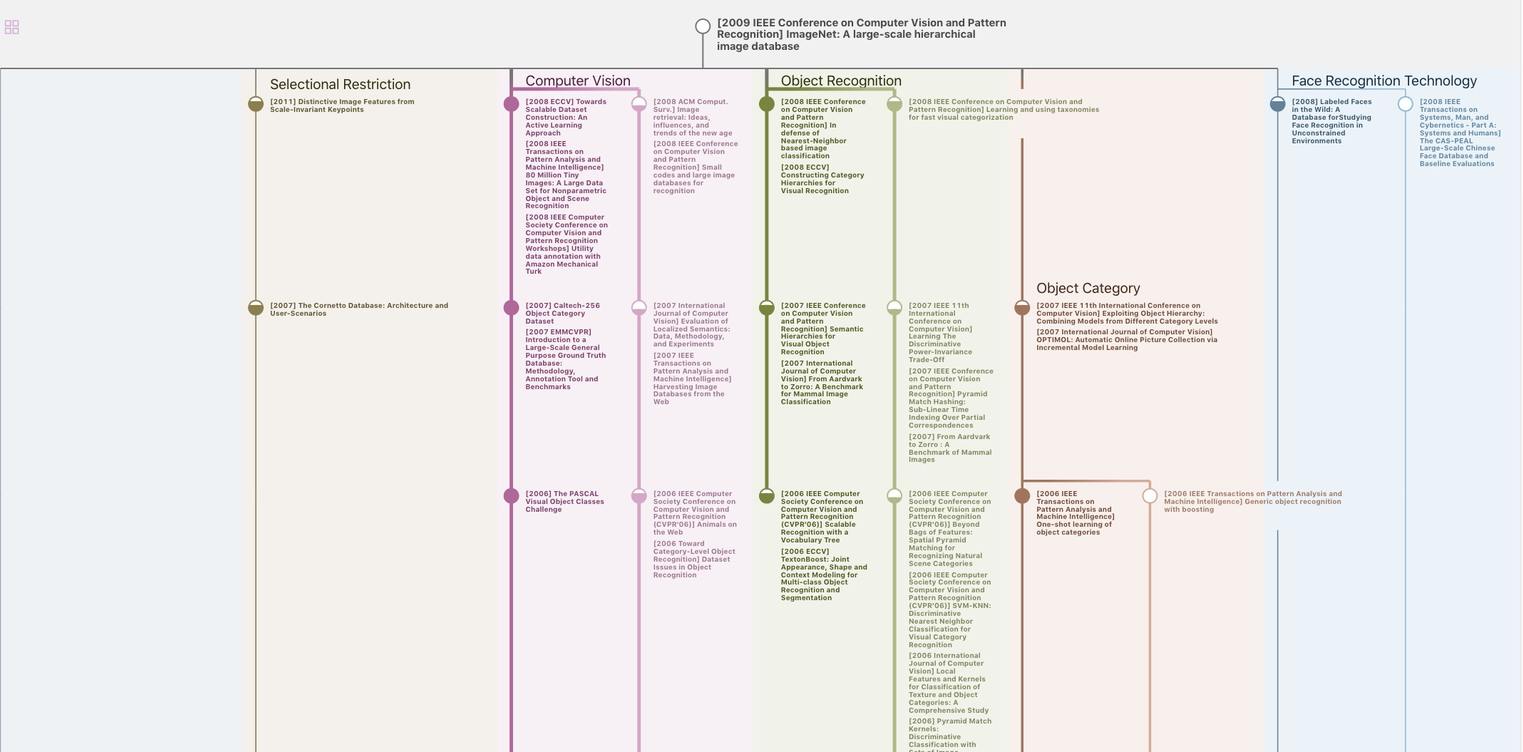
Generate MRT to find the research sequence of this paper
Chat Paper
Summary is being generated by the instructions you defined