Multitrend Conditional Value at Risk for Portfolio Optimization
IEEE TRANSACTIONS ON NEURAL NETWORKS AND LEARNING SYSTEMS(2024)
Abstract
Trend representation has been attracting more and more attention recently in portfolio optimization (PO) via machine learning methods. It adopts concepts and phenomena from the field of empirical and behavioral finance when little prior knowledge is obtained or strict statistical assumptions cannot be guaranteed. It is used mostly in estimating the expected asset returns, but hardly in measuring risk. To fill this gap, we propose a novel multitrend conditional value at risk (MT-CVaR), which embeds multiple trends and their influences in CVaR. Besides, we propose a novel PO model with this MT-CVaR as the risk metric and then design a solving algorithm based on the interior point method to compute the portfolio. Extensive experiments on six benchmark datasets from diverse financial markets with different frequencies show that MT-CVaR achieves the state-of-the-art investing performance and risk management.
MoreTranslated text
Key words
Market research,Portfolios,Optimization,Reactive power,Estimation,Task analysis,Information science,Conditional value at risk (CVaR),interior point method,multiple trends,portfolio optimization (PO)
AI Read Science
Must-Reading Tree
Example
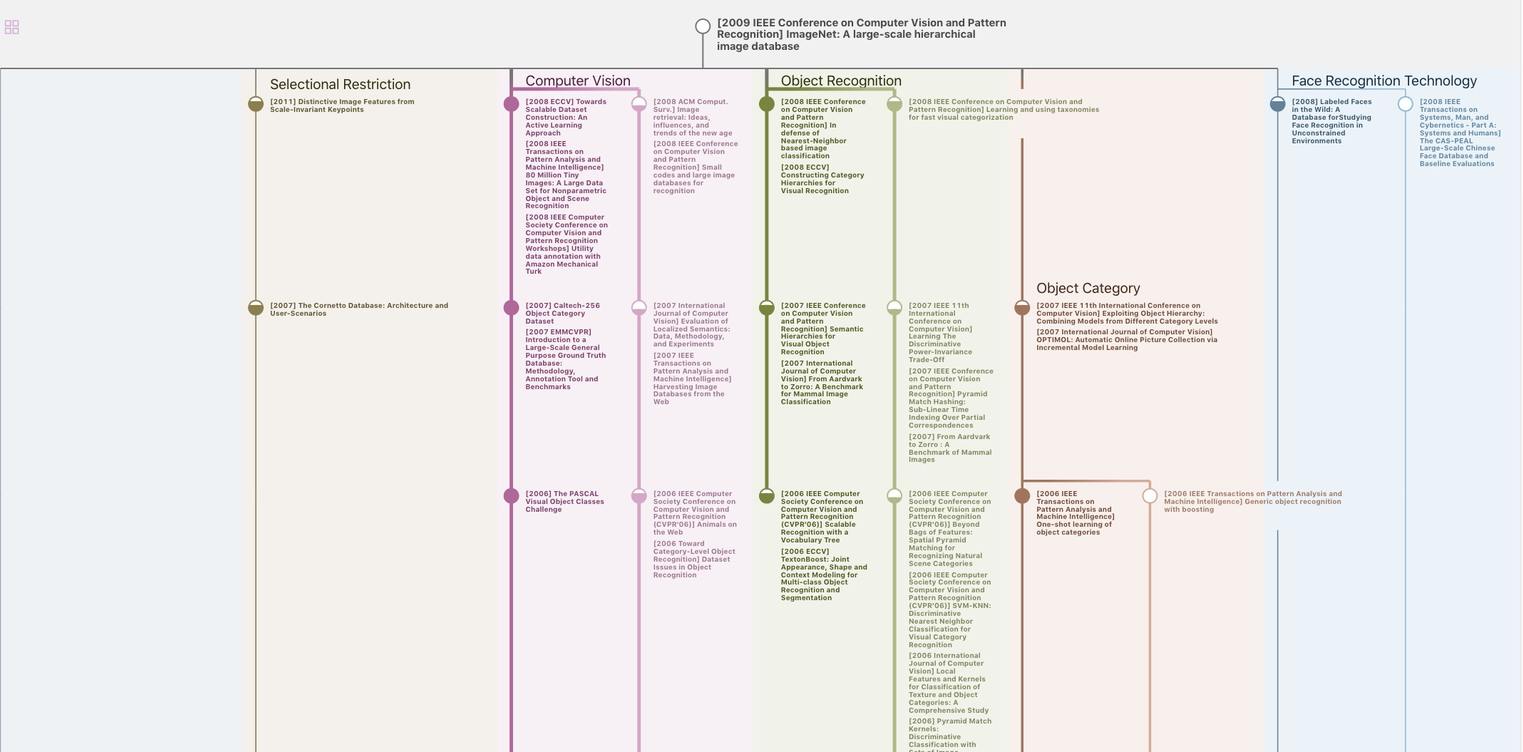
Generate MRT to find the research sequence of this paper
Chat Paper
Summary is being generated by the instructions you defined