Classification models and SAR analysis on HDAC1 inhibitors using machine learning methods
Molecular diversity(2022)
摘要
Histone deacetylase (HDAC) 1, a member of the histone deacetylases family, plays a pivotal role in various tumors. In this study, we collected 7313 human HDAC1 inhibitors with bioactivities to form a dataset. Then, the dataset was divided into a training set and a test set using two splitting methods: (1) Kohonen’s self-organizing map and (2) random splitting. The molecular structures were represented by MACCS fingerprints, RDKit fingerprints, topological torsions fingerprints and ECFP4 fingerprints. A total of 80 classification models were built by using five machine learning methods, including decision tree (DT), random forest, support vector machine, eXtreme Gradient Boosting and deep neural network. Model 15A_2 built by the XGBoost algorithm based on ECFP4 fingerprints showed the best performance, with an accuracy of 88.08% and an MCC value of 0.76 on the test set. Finally, we clustered the 7313 HDAC1 inhibitors into 31 subsets, and the substructural features in each subset were investigated. Moreover, using DT algorithm we analyzed the structure–activity relationship of HDAC1 inhibitors. It may conclude that some substructures have a significant effect on high activity, such as N -(2-amino-phenyl)-benzamide, benzimidazole, AR-42 analogues, hydroxamic acid with a middle chain alkyl and 4-aryl imidazole with a midchain of alkyl whose α carbon is chiral. Graphical abstract
更多查看译文
关键词
Classification models,Histone deacetylase (HDAC) 1 inhibitor,Machine learning method,Structure clustering,Structure–activity relationship (SAR)
AI 理解论文
溯源树
样例
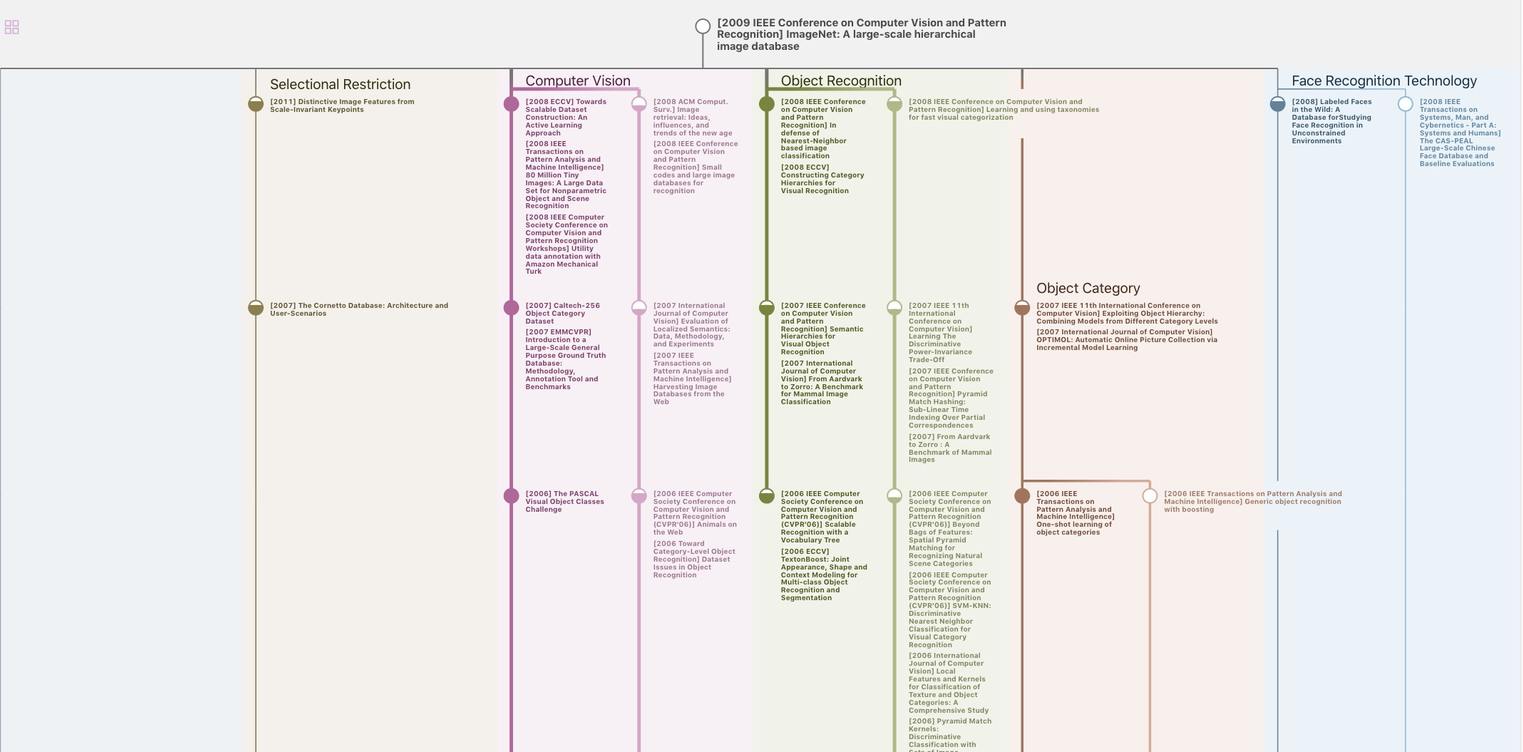
生成溯源树,研究论文发展脉络
Chat Paper
正在生成论文摘要