Anomaly Detection for Internet of Things Time Series Data Using Generative Adversarial Networks With Attention Mechanism in Smart Agriculture.
FRONTIERS IN PLANT SCIENCE(2022)
摘要
More recently, smart agriculture has received widespread attention, which is a deep combination of modern agriculture and the Internet of Things (IoT) technology. To achieve the aim of scientific cultivation and precise control, the agricultural environments are monitored in real time by using various types of sensors. As a result, smart agricultural IoT generated a large amount of multidimensional time series data. However, due to the limitation of applied scenarios, smart agricultural IoT often suffers from data loss and misrepresentation. Moreover, some intelligent decision-makings for agricultural management also require the detailed analysis of data. To address the above problems, this article proposes a new anomaly detection model based on generative adversarial networks (GAN), which can process the multidimensional time series data generated by smart agricultural IoT. GAN is a deep learning model to learn the distribution patterns of normal data and capture the temporal dependence of time series and the potential correlations between features through learning. For the problem of generator inversion, an encoder-decoder structure incorporating the attention mechanism is designed to improve the performance of the model in learning normal data. In addition, we also present a new reconstruction error calculation method that measures the error in terms of both point-wise difference and curve similarity to improve the detection effect. Finally, based on three smart agriculture-related datasets, experimental results show that our proposed model can accurately achieve anomaly detection. The experimental precision, recall, and F1 score exceeded the counterpart models by reaching 0.9351, 0.9625, and 0.9482, respectively.
更多查看译文
关键词
anomaly detection, smart agriculture, time series data, deep learning, generative adversarial network, attention mechanism
AI 理解论文
溯源树
样例
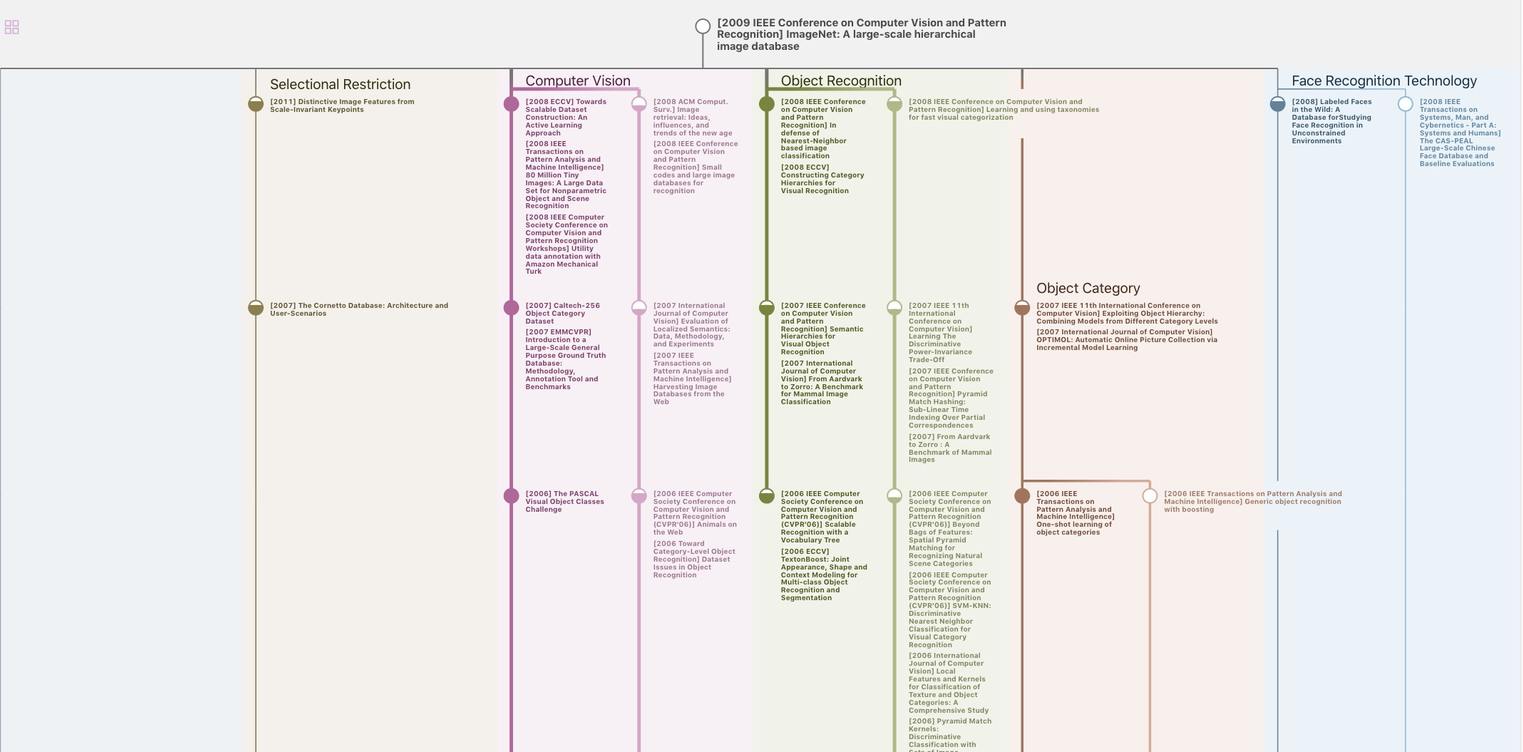
生成溯源树,研究论文发展脉络
Chat Paper
正在生成论文摘要