A Stack-Propagation Framework With Slot Filling for Multi-Domain Dialogue State Tracking
IEEE TRANSACTIONS ON NEURAL NETWORKS AND LEARNING SYSTEMS(2024)
摘要
Dialogue state tracking (DST) is a core component of task-oriented dialogue systems. Recent works focus mainly on end-to-end DST models that omit the spoken language understanding (SLU) module to directly obtain the dialogue state based on a user's dialogue. However, the slot information detected by slot filling in SLU is closely tied to the slot-value pair that needs to be updated in DST. Efficient use of the key slot semantic knowledge obtained by slot filling contributes to improving the performance of DST. Based on this idea, we introduce slot filling as a subtask and build an end-to-end joint model to explicitly integrate the slot information detected by slot filling, which further guides DST. In this article, a novel stack-propagation framework with slot filling for multidomain DST is proposed. The stack-propagation framework is introduced to jointly model slot filling and DST. The framework directly feeds the key slot semantic knowledge detected by slot filling into the DST module. In addition, a slot-masked attention mechanism is designed to enable DST to focus on the key slot information obtained by slot filling. When the slot value is updated, a slot-value softcopy mechanism is designed to enhance the influence of the words marked by key slots. Experiments show that our approach outperforms previous methods and performs outstandingly on two benchmark datasets.
更多查看译文
关键词
Task analysis,History,Context modeling,Semantics,Detectors,Computational modeling,Filling,Deep learning,dialogue state tracking (DST),dialogue system,slot filling
AI 理解论文
溯源树
样例
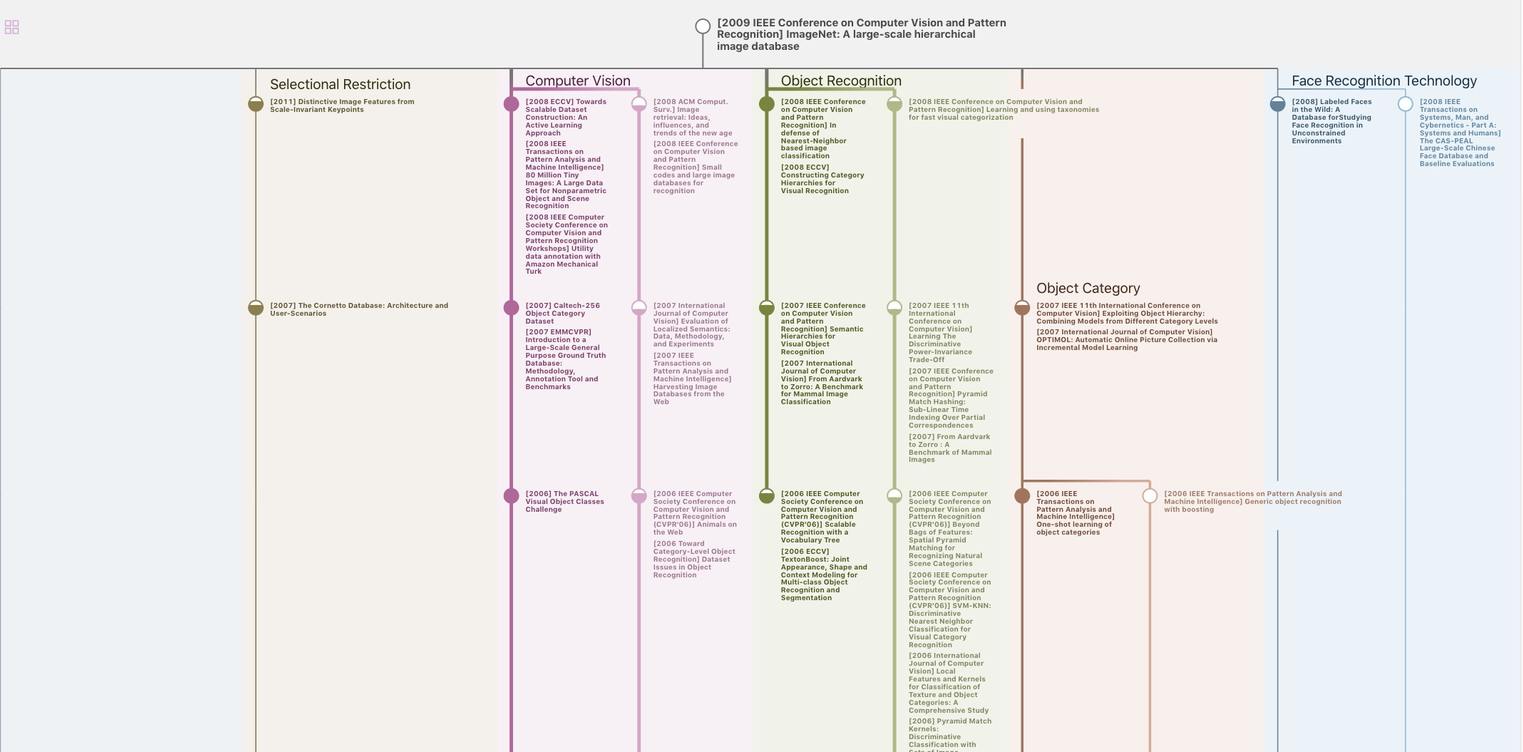
生成溯源树,研究论文发展脉络
Chat Paper
正在生成论文摘要