Predicting quorum sensing peptides using stacked generalization ensemble with gradient boosting based feature selection
Journal of Microbiology(2022)
摘要
Bacteria exist in natural environments for most of their life as complex, heterogeneous, and multicellular aggregates. Under these circumstances, critical cell functions are controlled by several signaling molecules known as quorum sensing (QS) molecules. In Gram-positive bacteria, peptides are deployed as QS molecules. The development of antibodies against such QS molecules has been identified as a promising therapeutic intervention for bacterial control. Hence, the identification of QS peptides has received considerable attention. Availability of a fast and reliable predictive model to effectively identify QS peptides can help the existing high throughput experiments. In this study, a stacked generalization ensemble model with Gradient Boosting Machine (GBM)-based feature selection, namely EnsembleQS was developed to predict QS peptides with high accuracy. On selected GBM features (791D), the EnsembleQS outperformed finely tuned baseline classifiers and demonstrated robust performance, indicating the superiority of the model. The accuracy of EnsembleQS is 4% higher than those resulting from ensemble model on hybrid dataset. When evaluating an independent data set of 40 QS peptides, the EnsembleQS model showed an accuracy of 93.4% with Matthew’s Correlation Coefficient (MCC) and area under the ROC curve (AUC) values of 0.91 and 0.951, respectively. These results suggest that EnsembleQS will be a useful computational framework for predicting QS peptides and will efficiently support proteomics research. The source code and all datasets used in this study are publicly available at https://github.com/proteinexplorers/EnsembleQS .
更多查看译文
关键词
Gram-positive bacterium, quorum sensing peptides, machine learning, stacked generalization ensemble, feature selection, gradient boosting machines
AI 理解论文
溯源树
样例
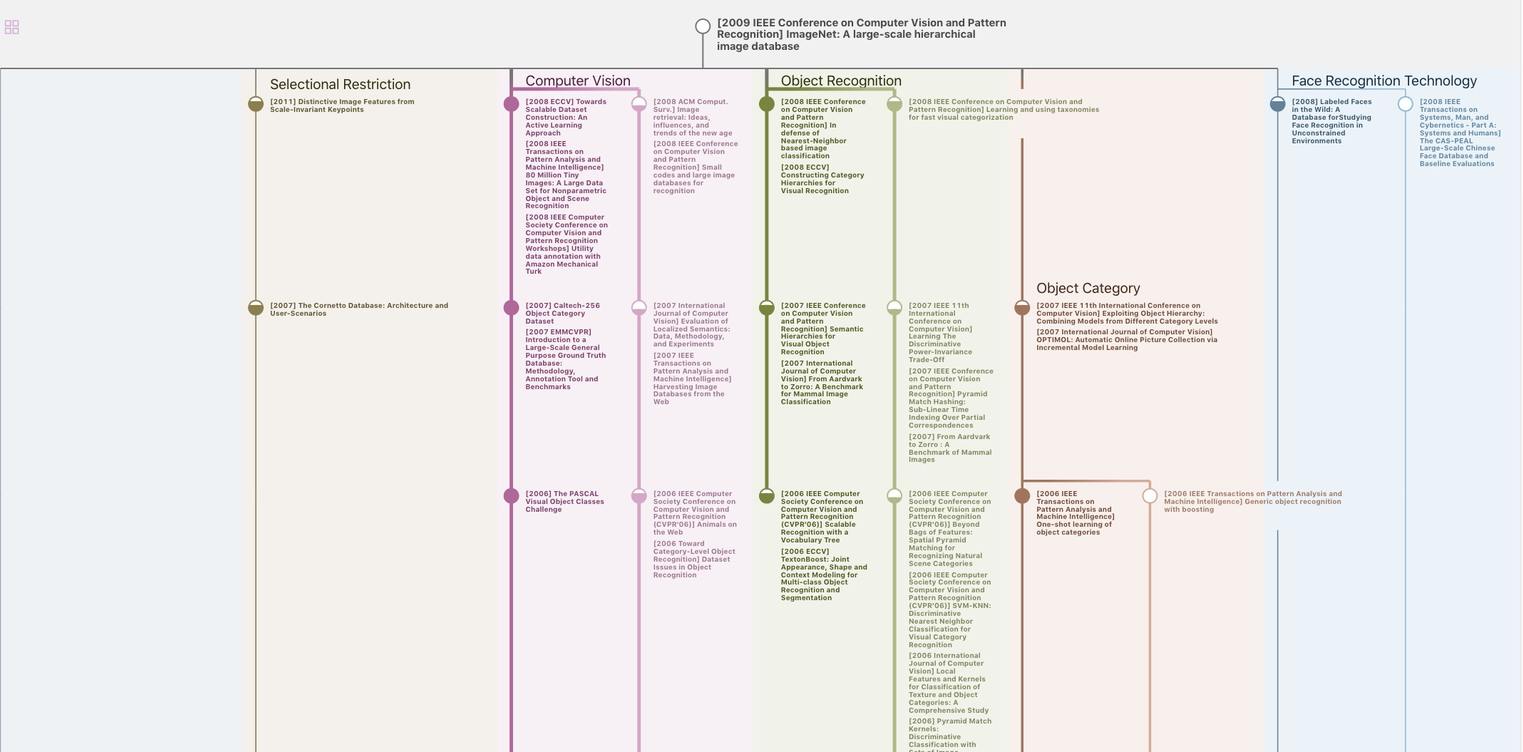
生成溯源树,研究论文发展脉络
Chat Paper
正在生成论文摘要