Assessing model accuracy using random data split: a simulation study.
Journal of biopharmaceutical statistics(2023)
摘要
Randomization is considered a safeguard against bias and a gold standard in clinical studies. To assess the generalizability of the accuracy of a model, a common approach is to randomly split a master data set into two parts: one for training and the other for testing. In this paper, we demonstrated the limitations of random split in assessing the generalizability of the accuracy of models through simulation studies. We generated three simulation data for binary or continuous endpoints, each with large sample size ( = 10,000). In each simulation scenario, we randomly split the data into two, one for training and one for testing, and then compare the performance of the model between training and testing data. All simulations were repeated 1,000 times. When random split was used, the model performance based on training and testing data behaved similarly in terms of the true positive fraction and false positive fraction for binary data and mean-squared errors for continuous data. However, when there is a time drift effect in the data, random split will result in large differences between training and testing data. As the training and testing data are similar through a random split, assessing the generalizability of the model on similar data will generate similar results. Generalizability of the accuracy of models is thus best achieved if testing is done in a distinct and independent study.
更多查看译文
关键词
Random split,false positive fraction,mean-squared error,true positive fraction
AI 理解论文
溯源树
样例
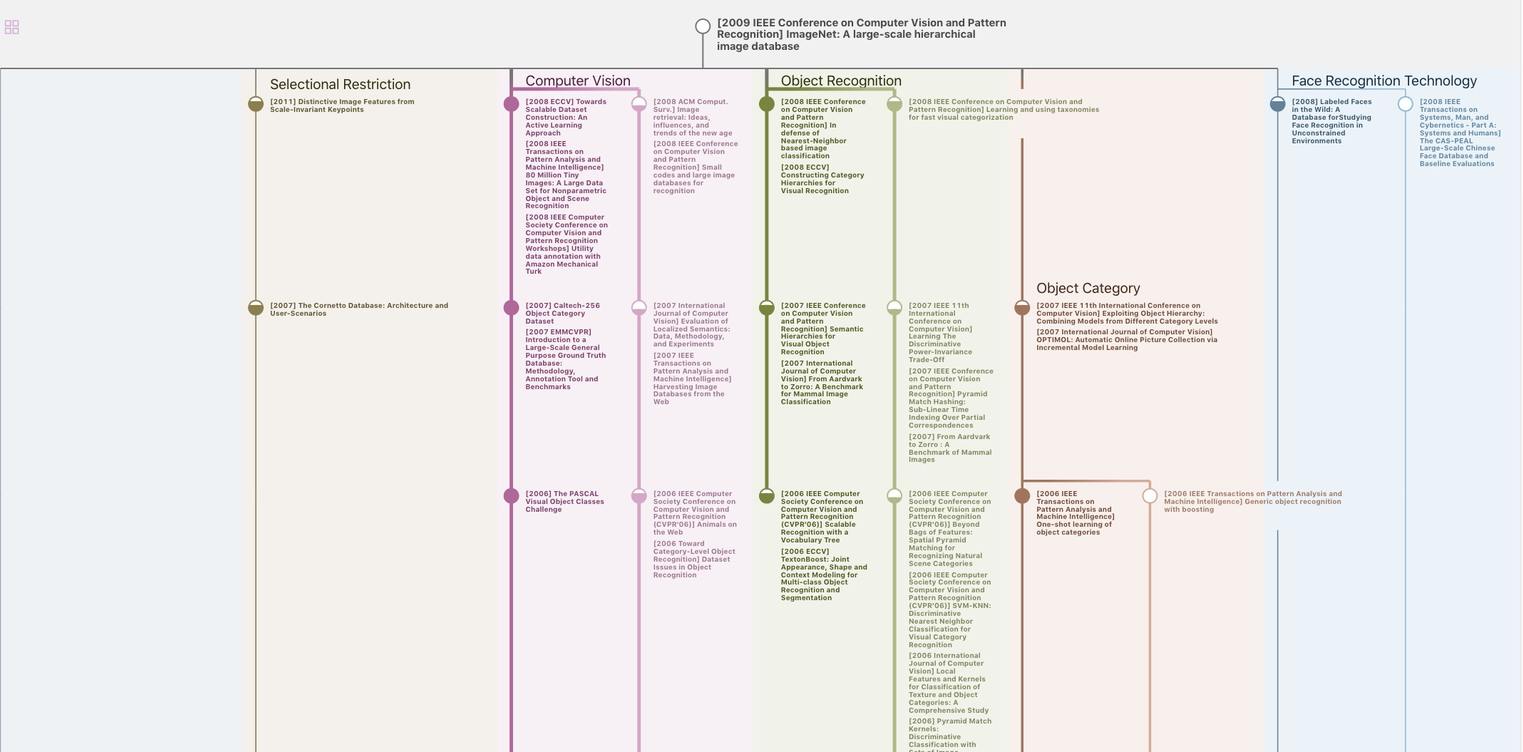
生成溯源树,研究论文发展脉络
Chat Paper
正在生成论文摘要