Attention-guided neural network for early dementia detection using MRS data.
Computerized medical imaging and graphics : the official journal of the Computerized Medical Imaging Society(2022)
摘要
Imaging bio-markers have been widely used for Computer-Aided Diagnosis (CAD) of Alzheimer's Disease (AD) with Deep Learning (DL). However, the structural brain atrophy is not detectable at an early stage of the disease (namely for Mild Cognitive Impairment (MCI) and Mild Alzheimer's Disease (MAD)). Indeed, potential biological bio-markers have been proved their ability to early detect brain abnormalities related to AD before brain structural damage and clinical manifestation. Proton Magnetic Resonance Spectroscopy (1H-MRS) provides a promising solution for biological brain changes detection in a no invasive manner. In this paper, we propose an attention-guided supervised DL framework for early AD detection using 1H-MRS data. In the early stages of AD, features may be closely related and often complex to delineate between subjects. Hence, we develop a 1D attention mechanism that explicitly guides the classifier to focus on diagnostically relevant metabolites for classes discrimination. Synthetic data are used to tackle the lack of data problem and to help in learning the feature space. Data used in this paper are collected in the University Hospital of Poitiers, which contained 111 1H-MRS samples extracted from the Posterior Cingulate Cortex (PCC) brain region. The data contain 33 Normal Control (NC), 49 MCI due to AD, and 29 MAD subjects. The proposed model achieves an average classification accuracy of 95.23%. Our framework outperforms state of the art imaging-based approaches, proving the robustness of learning metabolites features against traditional imaging bio-markers for early AD detection.
更多查看译文
AI 理解论文
溯源树
样例
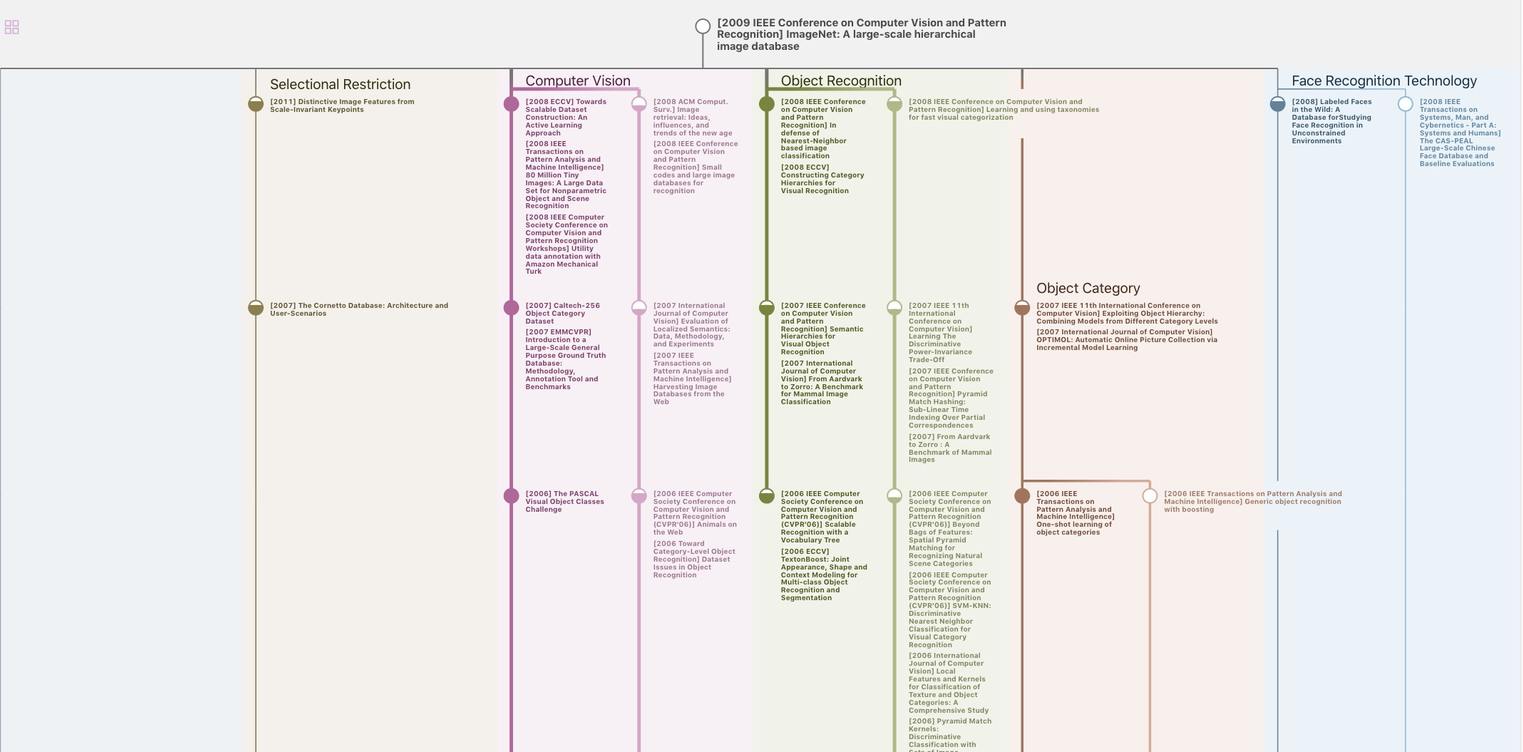
生成溯源树,研究论文发展脉络
Chat Paper
正在生成论文摘要