Heterogeneous hybrid extreme learning machine for temperature sensor accuracy improvement
EXPERT SYSTEMS WITH APPLICATIONS(2022)
摘要
High-accuracy temperature sensors usually come at a high cost, making them cost-ineffective solutions for mass-produced commercial products. The overall product cost can be one of the decisive factors for its success (e.g., commercial freezers). On the other hand, low-cost temperature sensors are viable options, but they suffer from accuracy issues. This paper presents a system aimed at improving the accuracy of a low-cost temperature sensor using a hybrid machine learning algorithm. The system utilizes a low-cost single-board computer connected to a series of sensors to collect data. These data are then used as an input vector to the proposed linear regression heterogeneous hybrid extreme learning machine (LR-HE-HyELM), a supervised learning algorithm responsible for increasing the sensors' accuracy. The proposed linear regression heterogeneous extreme learning machine (LR-HE-HyELM) combines linear regression with the self-adaptive heterogeneous hybrid extreme learning machine (SA-He-HyELM) algorithm. SA-He-HyELM is an extreme learning machine (ELM) based algorithm that utilizes a genetic algorithm (GA) for the creation of an optimized single-layer neural network (SLNN) having different combinations of custom-created neurons at its hidden layer. The proposed training algorithm has all the advantages of SA-He-HyELM. These advantages include utilizing low-order and higher-order neurons in the same network while retaining ELM's simplicity by making all GA parameters self adaptive. The utilization of low-order and higher-order unit types in the same network resulted in optimized neural networks with better generalization ability than those with the same neuron types in their hidden layer. The creation of SA-He-HyELM was nature-inspired since the human brain contains different neuron types. The proposed system is tested with eight existing methods, with most of them having different neuron types in the hidden layer. The experimental results involved using a custom-created regression dataset having measurements taken from a commercial fridge. The proposed method managed to get the lowest mean square error (MSE) compared to the other eight methods.
更多查看译文
关键词
Artificial neural network,Custom neuron,Genetic algorithm,Hybrid extreme learning machine,Linear regression,Regression problem
AI 理解论文
溯源树
样例
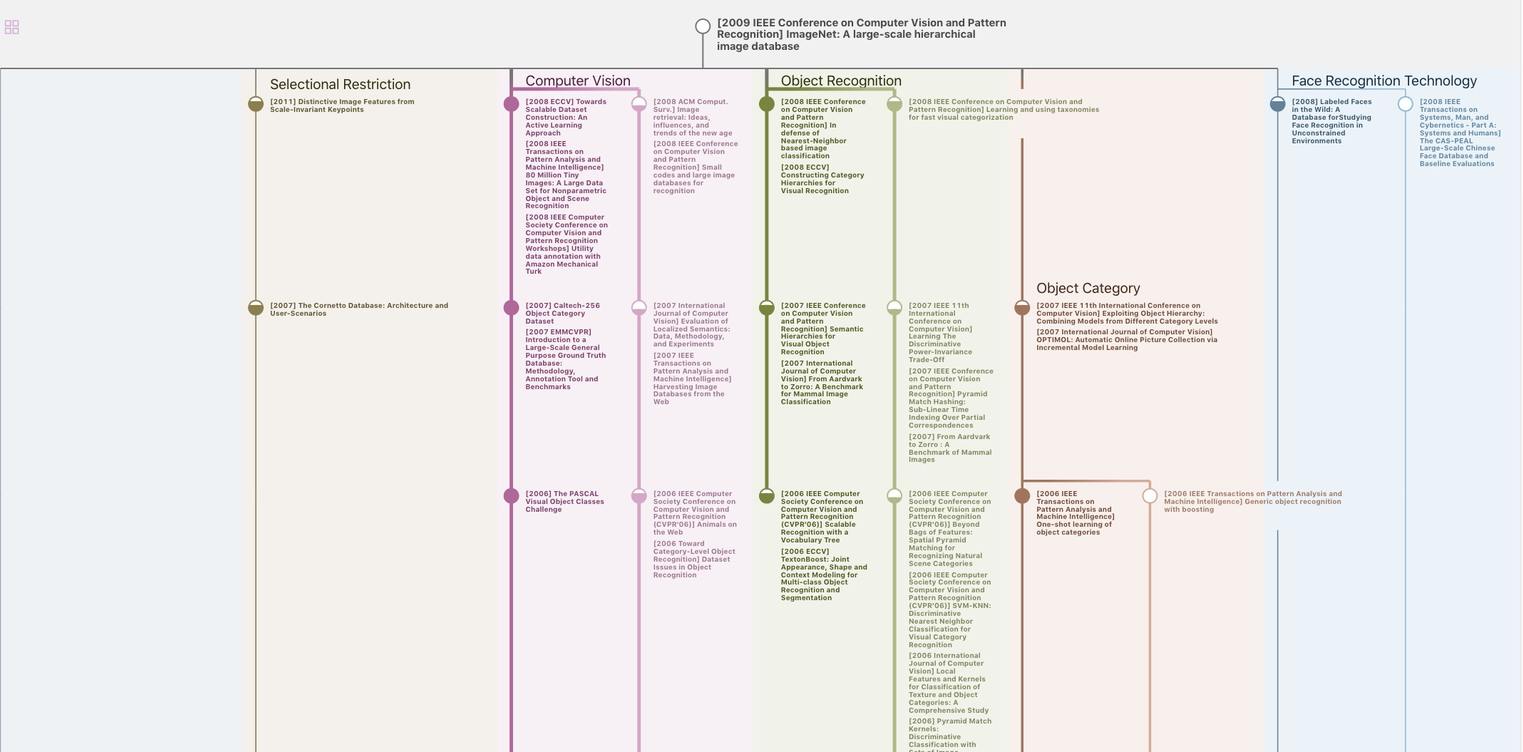
生成溯源树,研究论文发展脉络
Chat Paper
正在生成论文摘要