A Neuroevolutionary Approach for Opponent Modeling and Exploitation in No-limit Texas Hold’em Poker
2021 China Automation Congress (CAC)(2021)
Abstract
In recent years, the research of solving incomplete information games such as Texas Hold’em Poker game promotes the development of Artificial Intelligence(AI), which includes a variety of advanced theories and approaches such as game theory, reinforcement learning, neural network, tree search and so on. As a landmark area in AI, interest in Texas Hold’em Poker game has never dropped off, since the breakthroughs in this area can be potentially applied to many real-world problems varying from financial negotiations to military confrontations. In this paper, we proposed a new framework with population-based neuroevolution for the problem of opponent modeling and exploitation in Texas Hold’em Poker. The framework composes of two core connected components: opponent network and game network. The opponent network is used to capture the opponents’ significant features that can be exploited and the pre-trained game network can thus make decisions accordingly. The game network is an end-to-end recurrent neural network and is evolved with a genetic algorithm in the training process. The feasibility of this scheme is verified through preliminary experiments in specific game scenarios.
MoreTranslated text
Key words
incomplete information game,opponent modeling,population-based neuroevolution
AI Read Science
Must-Reading Tree
Example
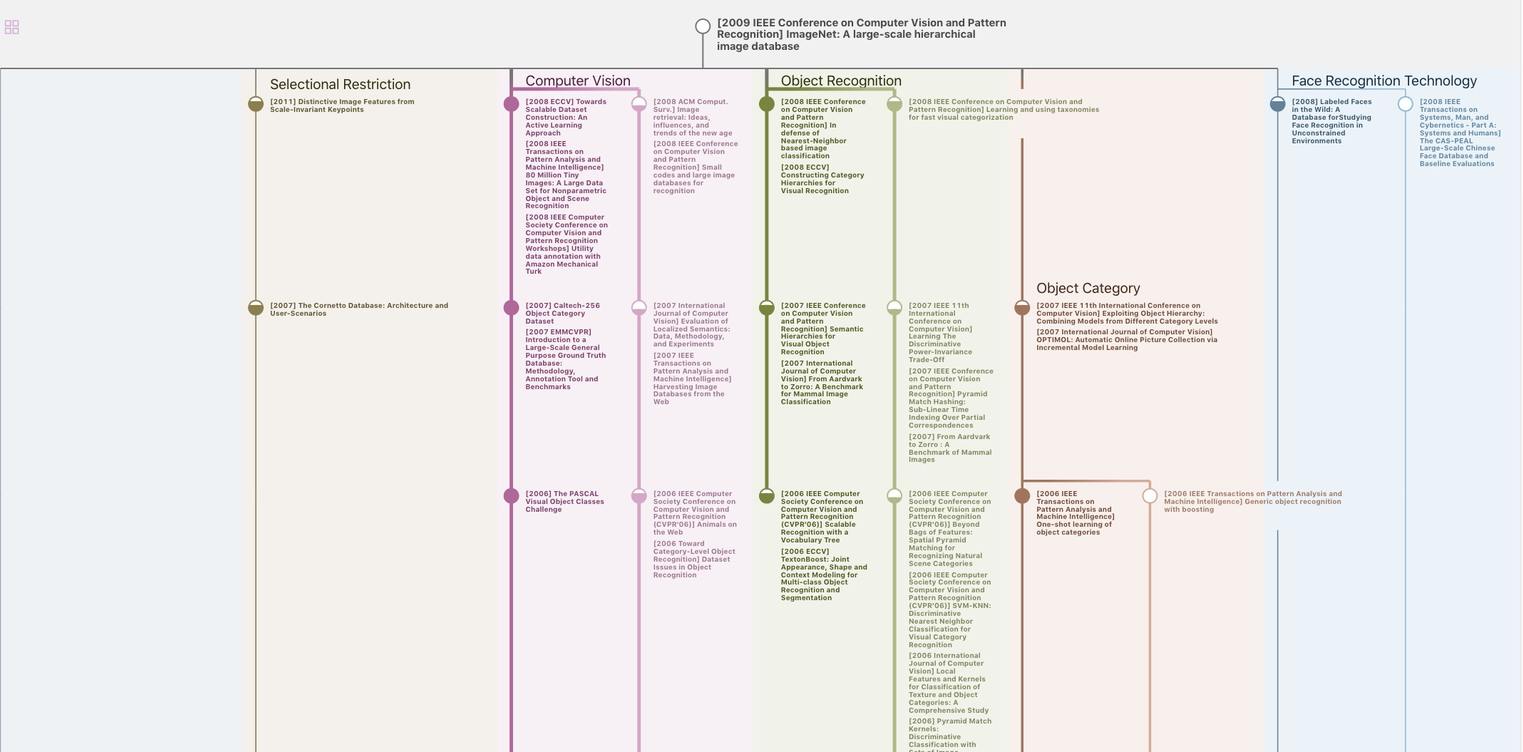
Generate MRT to find the research sequence of this paper
Chat Paper
Summary is being generated by the instructions you defined