GPR denoising via shearlet transformation and a data-driven tight frame
Near Surface Geophysics(2022)
摘要
Ground-penetrating radar (GPR) is commonly used to detect buried and near-surface geophysical structures. GPR denoising is necessary because some level of interference, such as from clutter, random noise and/or the column artefact, are inevitable and can cause false geological interpretations. Existing sparse representation methods, including wavelet transformation, curvelet transformation and dictionary learning, are critical in GPR denoising. However, they perform poorly in some cases because GPR data cannot be represented efficiently under severe interference. Thus, this study proposes an approach that combines shearlet transformation (ST) and a data-driven tight frame (DDTF) to improve data sparsity. The ST can provide the prior information of GPR data to the DDTF, while the DDTF can self-adaptively represent GPR data. First, we separate significant reflections and interferences using ST. Second, we apply the DDTF to further suppress the interferences by setting different thresholds in different ST scales and directions. Finally, we adopt inverse transformations to recover the GPR data. In the experiments, ST is used to show the differences between the significant reflections and interferences of the synthetic GPR data. We also sequentially remove each interference of the synthetic GPR data to clearly highlight the performance of the method. To ensure the effectiveness of the ST-DDTF approach, we test the method using synthetic GPR data from different models, along with some example field GPR data. The ST-DDTF method, which is aimed at improving data sparsity, shows state-of-the-art results relative to more standard GPR denoising methods. Although our approach is time consuming, it is useful in processing small GPR data and obtaining accurate denoising results.
更多查看译文
关键词
Data processing, Filtering, Frequency, Ground-penetrating radar
AI 理解论文
溯源树
样例
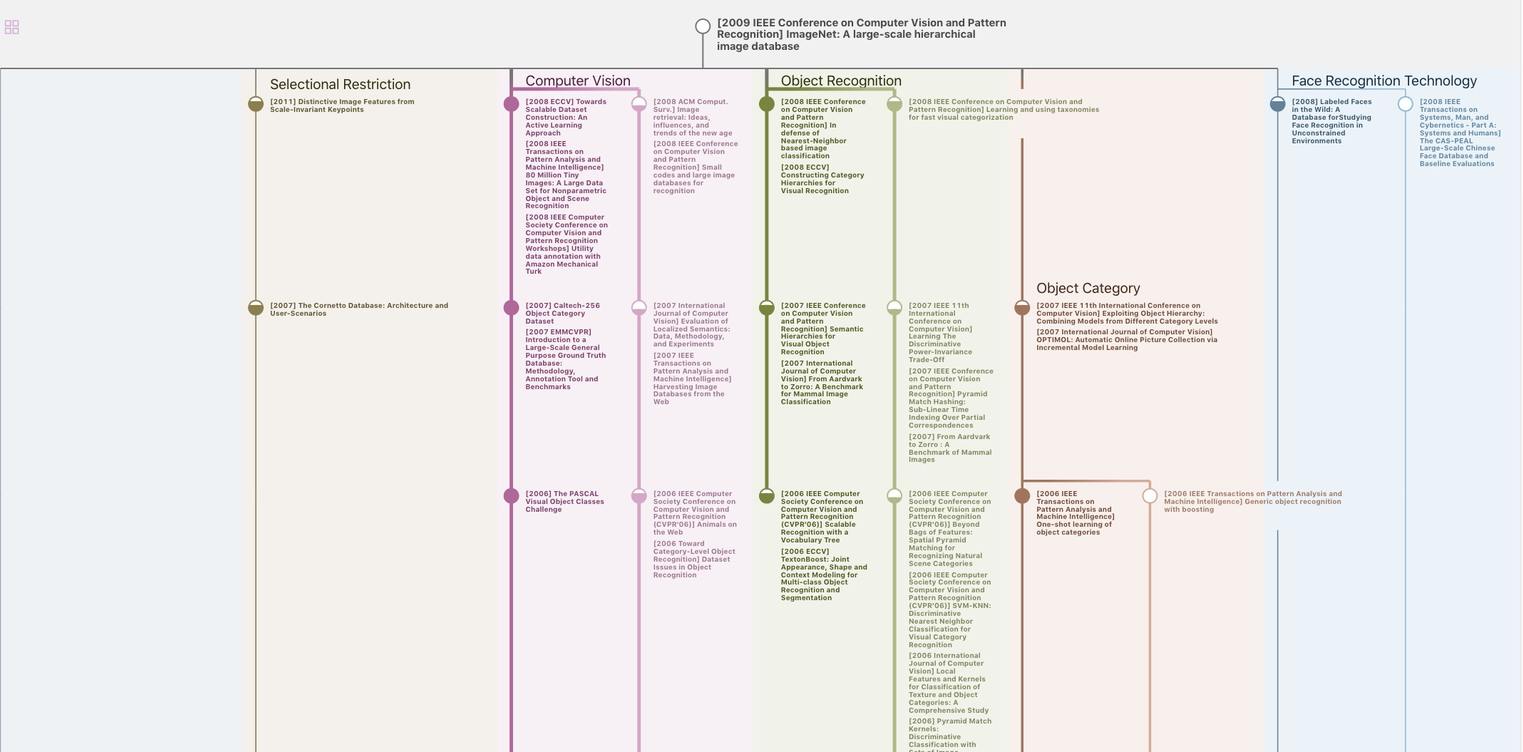
生成溯源树,研究论文发展脉络
Chat Paper
正在生成论文摘要