Real-Scenes Face Super-Resolution using a Lightweight Generative Adversarial Network
2021 3rd International Conference on Machine Learning, Big Data and Business Intelligence (MLBDBI)(2021)
摘要
Although deep learning-based methods have achieved great success in the field of image super-resolution (SR), the SR algorithms still have some shortcomings, such as unsatisfactory results in reconstructing face images collected in real scenes, the model has a large amount of calculation and serious delays when running in a real-time scene. To solve the above problems, this paper proposes a SR algorithm for face images based on a lightweight Generative Adversarial Network (GAN). The algorithm in this paper constructs a real-scenes face datasets for training. Based on the ESRGAN, this study completes the lightweight reconstruction of the basic network inspired by MobileNetV3. Experimental results show that the algorithm is better than the existing mainstream SR algorithms in terms of image reconstruction time, model volume, and parameters amount. When the test image is a face image with an unknown degradation method collected in a real scene, the algorithm in this paper can also achieve the ideal reconstruction effect.
更多查看译文
关键词
Generative Adversarial Network,real scenes,super-resolution,lightweight,deep separable convolution
AI 理解论文
溯源树
样例
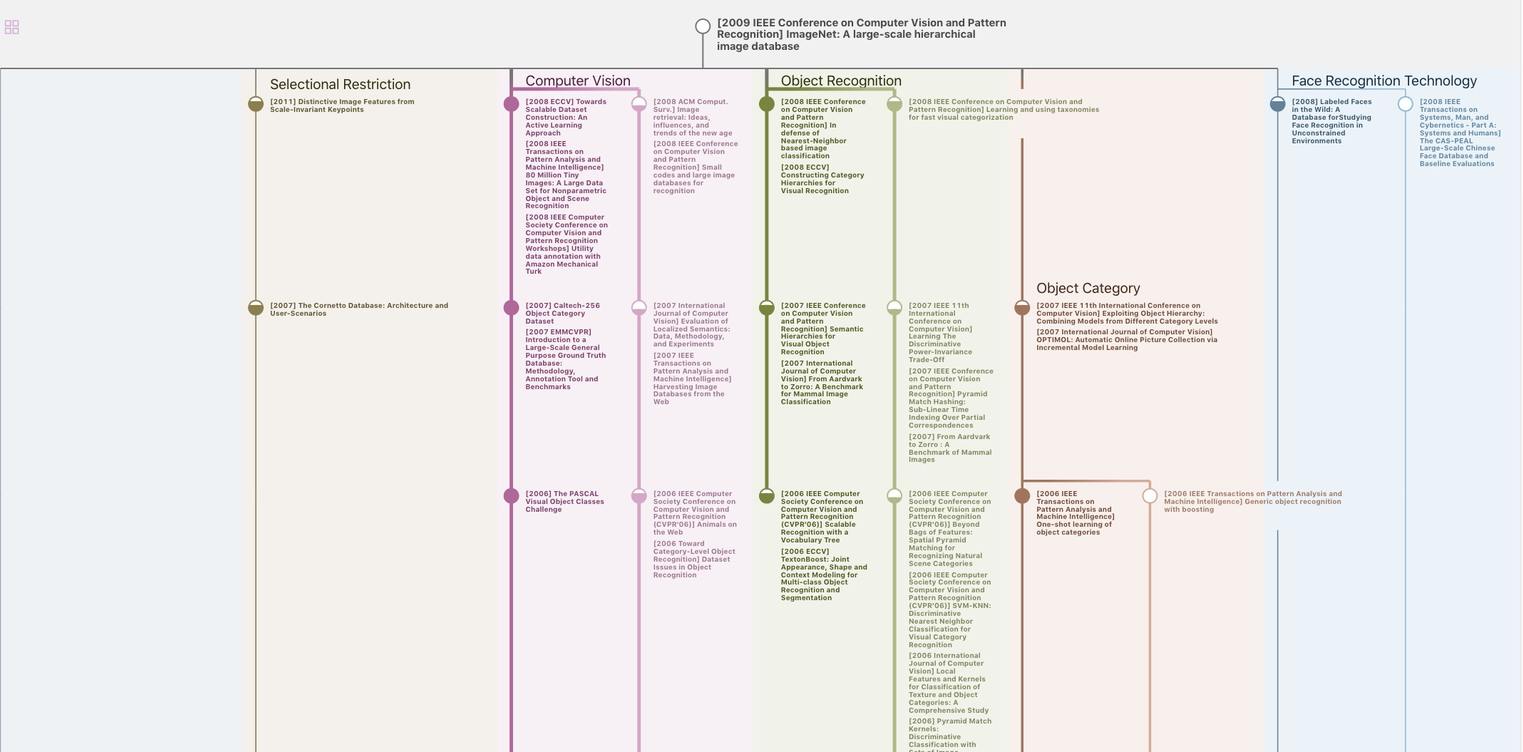
生成溯源树,研究论文发展脉络
Chat Paper
正在生成论文摘要