Patient Aware Active Learning for Fine-Grained OCT Classification
arxiv(2022)
摘要
This paper considers making active learning more sensible from a medical perspective. In practice, a disease manifests itself in different forms across patient cohorts. Existing frameworks have primarily used mathematical constructs to engineer uncertainty or diversity-based methods for selecting the most informative samples. However, such algorithms do not present themselves naturally as usable by the medical community and healthcare providers. Thus, their deployment in clinical settings is very limited, if any. For this purpose, we propose a framework that incorporates clinical insights into the sample selection process of active learning that can be incorporated with existing algorithms. Our medically interpretable active learning framework captures diverse disease manifestations from patients to improve generalization performance of OCT classification. After comprehensive experiments, we report that incorporating patient insights within the active learning framework yields performance that matches or surpasses five commonly used paradigms on two architectures with a dataset having imbalanced patient distributions. Also, the framework integrates within existing medical practices and thus can be used by healthcare providers.
更多查看译文
关键词
classification,learning,patient,fine-grained
AI 理解论文
溯源树
样例
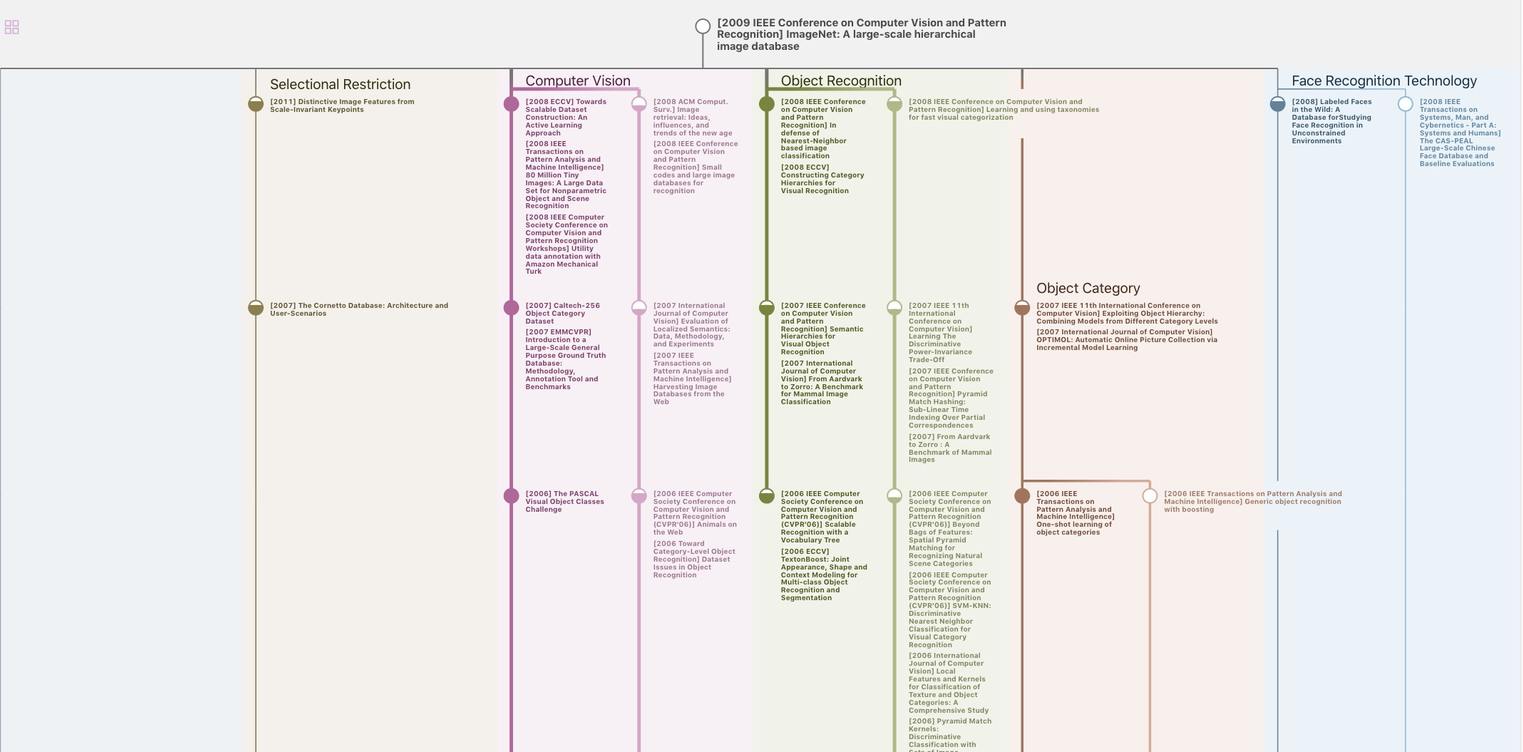
生成溯源树,研究论文发展脉络
Chat Paper
正在生成论文摘要