Position Paper
Proceedings of the 1st on Workshop on Robust Malware Analysis(2022)
摘要
Along the evolution of malware detection systems, adversaries develop sophisticated evasion techniques that render malicious samples undetectable. Especially for ML-based detection systems, an effective approach is to craft adversarial malware to evade detection. In this position paper, we conduct a critical review of existing adversarial attacks against malware detection, and conclude that current research focuses mainly on evasion techniques against static analysis; generating adversarial Windows samples to evade dynamic analysis remains largely unexplored. In the context of black-box attack scenarios, we investigate an adversary's potential to carry out practical transformations in order to influence behavioral features observed by ML systems and security products. Moreover, we investigate the range of dynamic behavior transformations and identify critical properties and associated challenges that relate to feasibility, automation, technical costs and detection risks. Through this discussion, we propose solutions to important challenges and present promising paths for future research on evasive malware under dynamic analysis.
更多查看译文
关键词
position paper
AI 理解论文
溯源树
样例
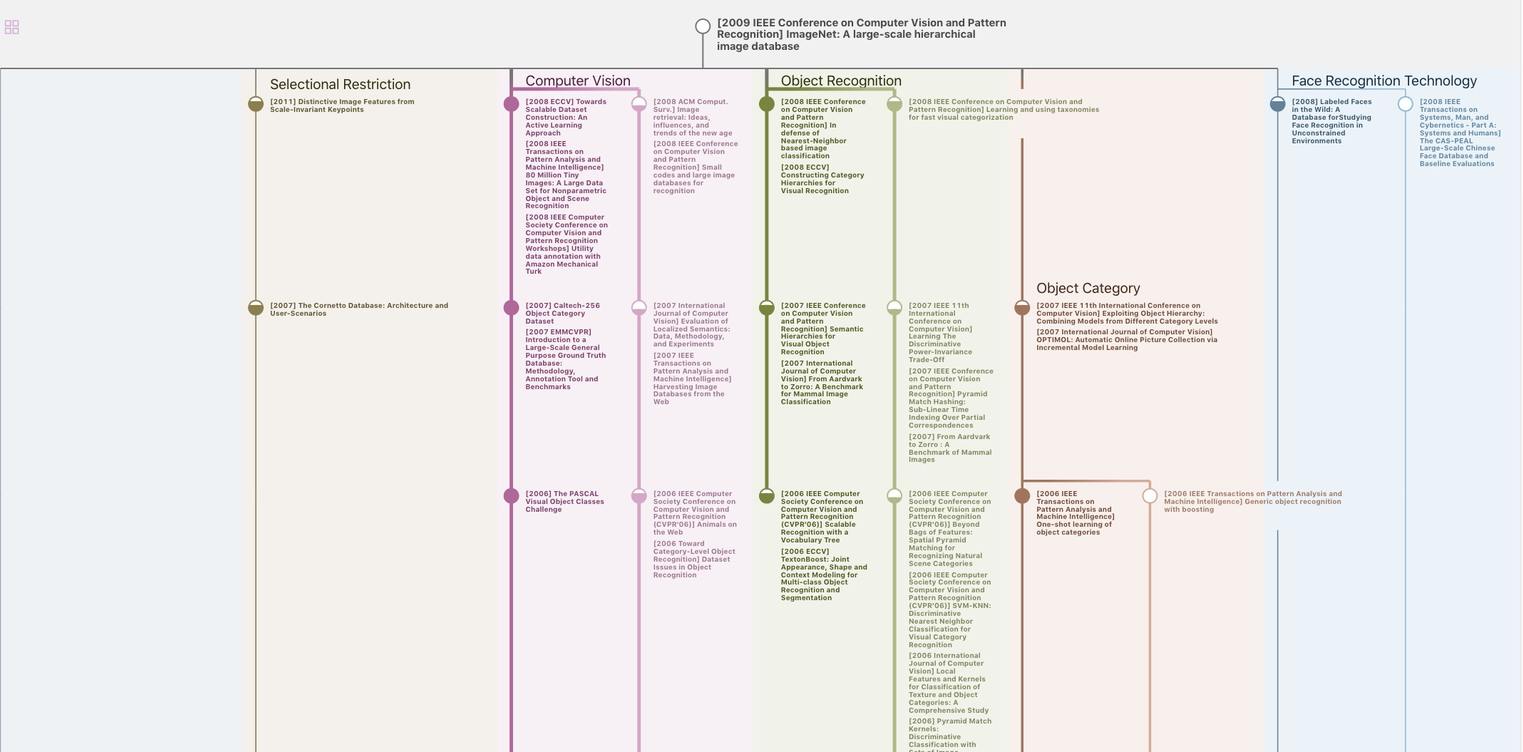
生成溯源树,研究论文发展脉络
Chat Paper
正在生成论文摘要