Curating Computer Science Educational Content with Machine Learning: Analyzing Learner Ratings within an Algorithmic Recommender System
TMS Proceedings 2021(2022)
摘要
Machine learning (ML) can in theory be used to personalize educational content by identifying online activities aligned with learnersâ interests. Yet, are learnersâ self-reported ratings of activities associated with a machine learning generated recommender score? In the current study we sought to address this question using learnerâs ratings of activity units (i.e., âbadgesâ) based on a conventional 7-item Likert scale administered immediately afterwards within an online app designed to teach computer programming skills. The sample included 78 learners (MeanAge = 13.2 years, SDAge = .84 years, %female = 37.2%) enrolled in schools and after-school programs in the United States. Even after controlling for other factors, such as the position of the recommendation on a list and the number of previous selections the learner had made, as well as certain learner demographics, there was a significant positive association between overall badge approval ratings provided by learners and the recommender score. These findings provide validity evidence in support of the ML- generated recommender score by suggesting badges that the learner is likely to approve of, even when considering other relevant factors that could affect their selection of badges, such as the position of the recommendation on the list and the number of selections made by the learner. Further work should seek to establish whether the likelihood of a learner selecting a highly ranked activity is associated with improvement in more domain general attitudes towards computer programming and whether this association is moderated or robust to certain demographic factors.
更多查看译文
AI 理解论文
溯源树
样例
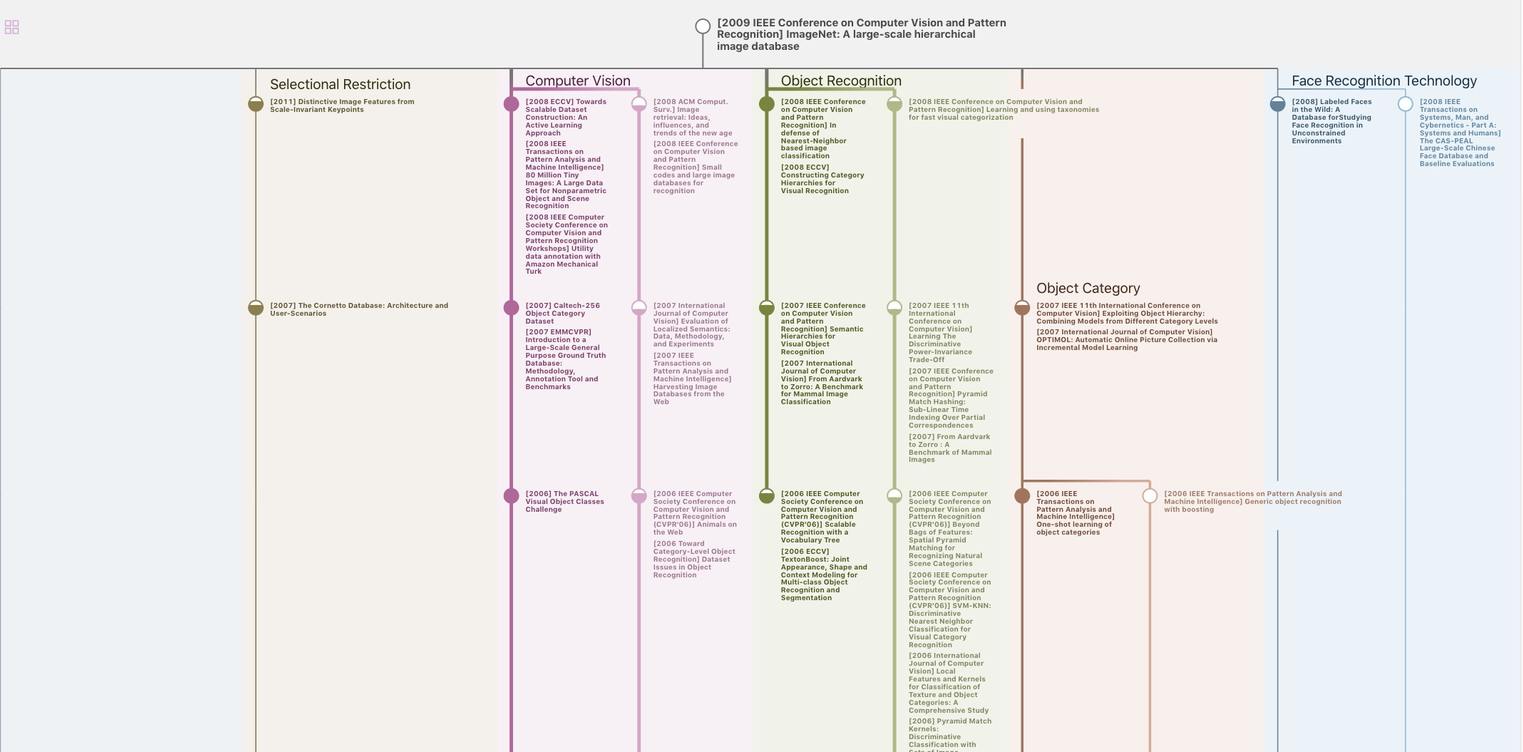
生成溯源树,研究论文发展脉络
Chat Paper
正在生成论文摘要