JointMatcher: Numerically-aware entity matching using pre-trained language models with attention concentration
Knowledge-Based Systems(2022)
摘要
Entity matching (EM) aims to identify whether two records refer to the same underlying real-world entity. Traditional entity matching methods mainly focus on structured data, where the attribute values are short and atomic. Recently, there has been an increasing demand for matching textual records, such as matching descriptions of products that correspond to long spans of text, which challenges the applications of these methods. Although a few deep learning (DL) solutions have been proposed, these solutions tend to “directly” use the DL techniques and treat the EM as NLP tasks without determining the unique demand for the EM task. Thus, the performance of these DL-based solutions is still far from satisfactory. In this paper, we present JointMatcher, a novel EM method based on the pre-trained Transformer-based language models so that the generated features of the textual records contain the context information. We realize that more attention paid to the similar segments and number-contained segments of the record pair is crucial for accurate matching. To integrate the high-contextualized features with the consideration of paying more attention to the similar segments and the number-contained segments, JointMatcher is equipped with the relevance-aware encoder and the numerically-aware encoder. Extensive experiments using structured and real-world textual datasets demonstrated that JointMatcher outperforms the previous state-of-the-art (SOTA) results without injecting any domain knowledge when small or medium size training sets are used.
更多查看译文
关键词
Entity matching,Pre-trained language model,Attention concentration
AI 理解论文
溯源树
样例
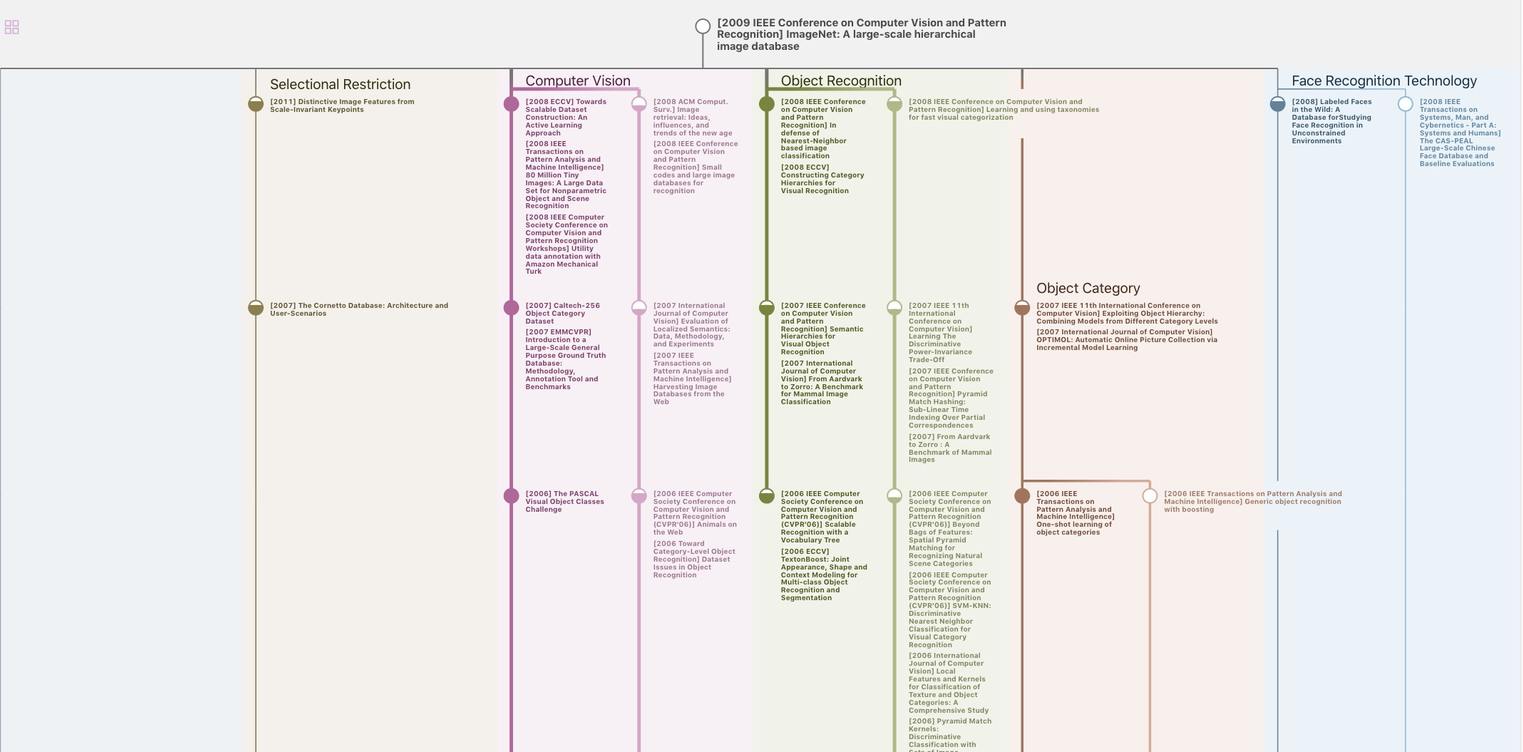
生成溯源树,研究论文发展脉络
Chat Paper
正在生成论文摘要