Optimal Energy-Centric Resource Allocation and Offloading Scheme for Green Internet of Things Using Machine Learning
ACM TRANSACTIONS ON INTERNET TECHNOLOGY(2022)
摘要
Resource allocation and offloading in green Internet of Things (IoT) relies on the multi-level heterogeneous platforms. The energy expenses of the platform determine the reliability of green IoT based services and applications. This manuscript introduces a decisive energy management scheme for optimal resource allocation and offloading along with energy constraints. This scheme handles both the allocation and energy-cost in a balanced manner through deterministic task offloading. In particular, resource allocation solution for non-delay tolerant green IoT applications is focused by confining the failures of discrete tasks through neural learning. The dropout process augmented with the learning process improves the feasible conditions for resource handling and task offloading among the active IoT service providers. Through extensive simulations the performance of the proposed scheme is analyzed and energy consumption, failure rate, processing, and completion time metrics are used for a comparative study. Further, the optimal utilization and on-demand dissipation of such stored resources help to improve the sustainability of green power and communication technologies in the smart city environment.
更多查看译文
关键词
Dropout learning,green IoT,neural network,resource allocation,task offloading
AI 理解论文
溯源树
样例
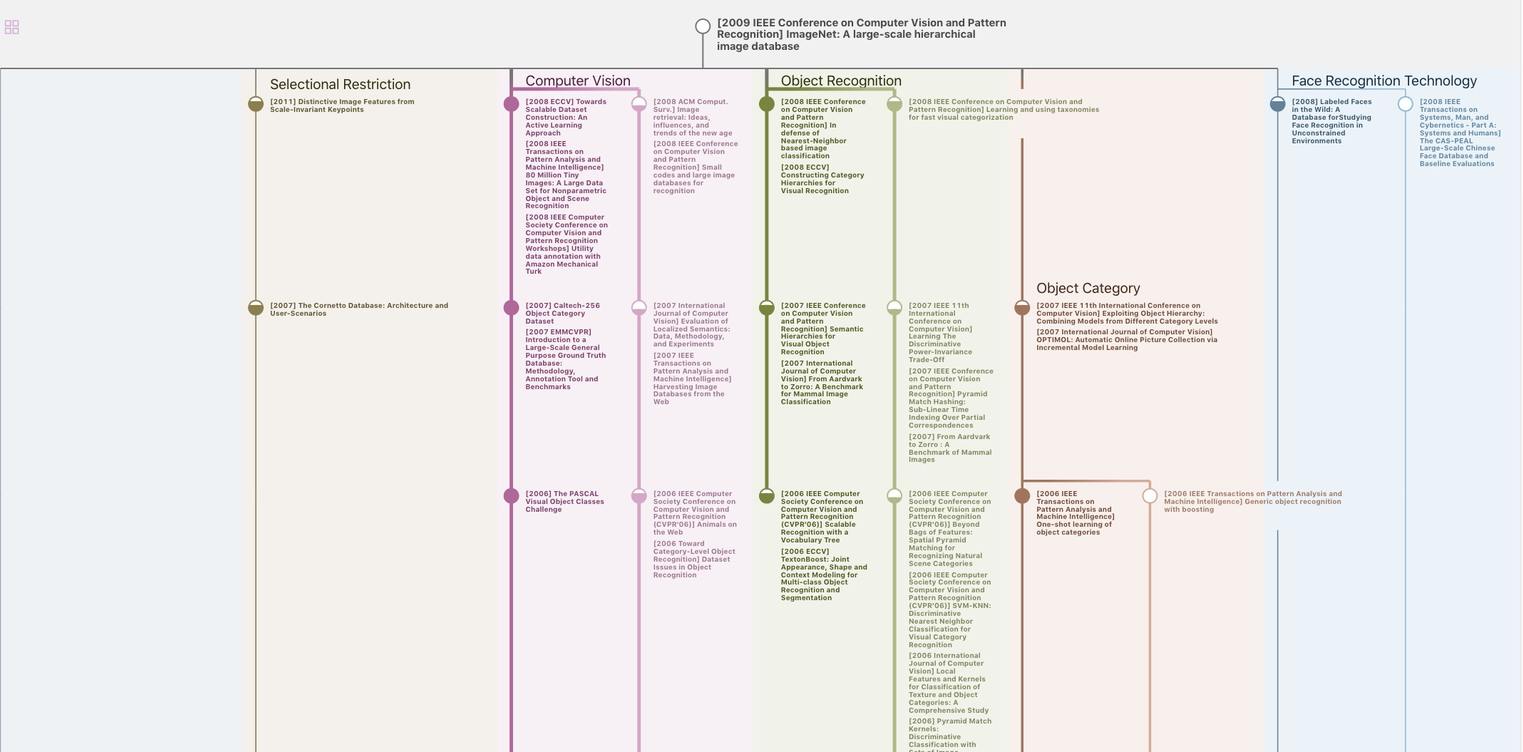
生成溯源树,研究论文发展脉络
Chat Paper
正在生成论文摘要