D-LIOM: Tightly-Coupled Direct LiDAR-Inertial Odometry and Mapping
IEEE Transactions on Multimedia(2023)
摘要
Simultaneous localization and mapping via LiDARInertial fusion is a crucial technology in many automation-related applications. Recently, a number of approaches based on geometric features have evolved, yielding impressive results via tightly-coupled estimation. This sort of feature-based techniques, however, are inextricably linked to the scanning mechanism of the LiDAR, relying on stable feature detection, and thus are difficult to adapt to multi-LiDAR systems. A few "direct" solutions, on the other hand, register the raw point cloud with the built probability map, which is more computationally efficient and easy to be extended. But, the existing direct approaches are all loosely-coupled, lacking correction of the IMU biases, and thus only work well in 2D cases. To this end, we present D-LIOM, a tightly-coupled Direct LiDAR-Inertial Odometry and Mapping framework. In D-LIOM, a scan is directly registered to a probability submap, and the LiDAR odometry, the IMU pre-integration, and the gravity constraint are integrated to build a local factor graph in the submap's time window, allowing the system to perform real-time high-precision pose estimation. Furthermore, to eliminate accumulated errors in time, we detect loops and adjust the sparse pose graph based on mutual matching of projected 2D submaps, allowing D-LIOM to run stably in large-scale scenes. In addition, to improve its flexibility to varied sensor combinations, D-LIOM supports multi-LiDAR inputs and facilitates the initialization with a common 6-axis IMU. Extensive experiments demonstrate that D-LIOM largely outperforms the existing state-of-the-art counterparts in mapping effect and localization accuracy as well as with high time efficiency. Lastly, to ensure that our results are entirely reproducible, all necessary data and codes are made open-source available. One introduction video can also be found on the online website.
更多查看译文
关键词
Feature extraction,Laser radar,Simultaneous localization and mapping,Point cloud compression,Sensors,Three-dimensional displays,Gravity,LiDAR-Inertial odometry,SLAM,loop detection,data fusion
AI 理解论文
溯源树
样例
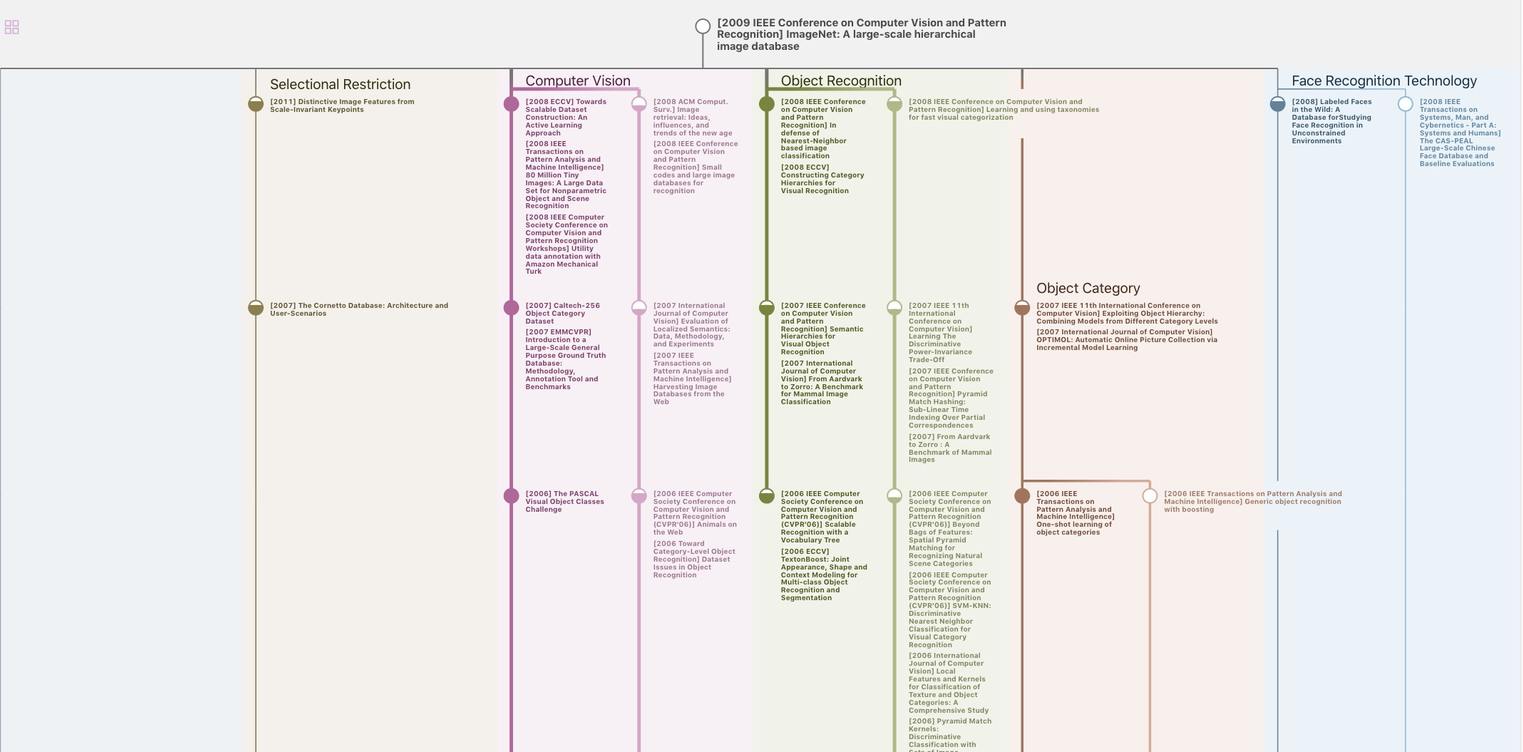
生成溯源树,研究论文发展脉络
Chat Paper
正在生成论文摘要