Regularized Label Relaxation with Negative Technique for Image Classification
Multimedia tools and applications(2022)
Abstract
Multiclass classification is a challenging task in the field of pattern recognition. Recently, linear regression (LR) and a number of its variants play a key role in classification problems. However, most of these methods manage to transform the training samples into a rigorous binary label matrix, which result in little freedom to fit the samples adequately. In addition, these variants cannot obtain a robust classification performance when dealing with noisy and contaminated data. In order to address the problems, we propose a new learning framework, which holds the following extraordinary advantages. First, a negative ε dragging technique is introduced to release the binary label matrix, which has more freedom to fit the samples and reduces the class margins between different classes for getting robust results from noisy data. Second, the manifold learning, as a regularized item, is applied to construct the class compactness graph, which can prevent the overfitting problem. In this paper, the proposed algorithm is designed based on the ℓ 2, 1 -norm loss function as it takes both superiorities of the systemic representation of ℓ 2 -norm and the discriminative nature of the ℓ 1 -norm. A large number of experiments show that our method has a good performance.
MoreTranslated text
Key words
Linear regression,Negative ε dragging,Manifold learning,Class compactness graph
AI Read Science
Must-Reading Tree
Example
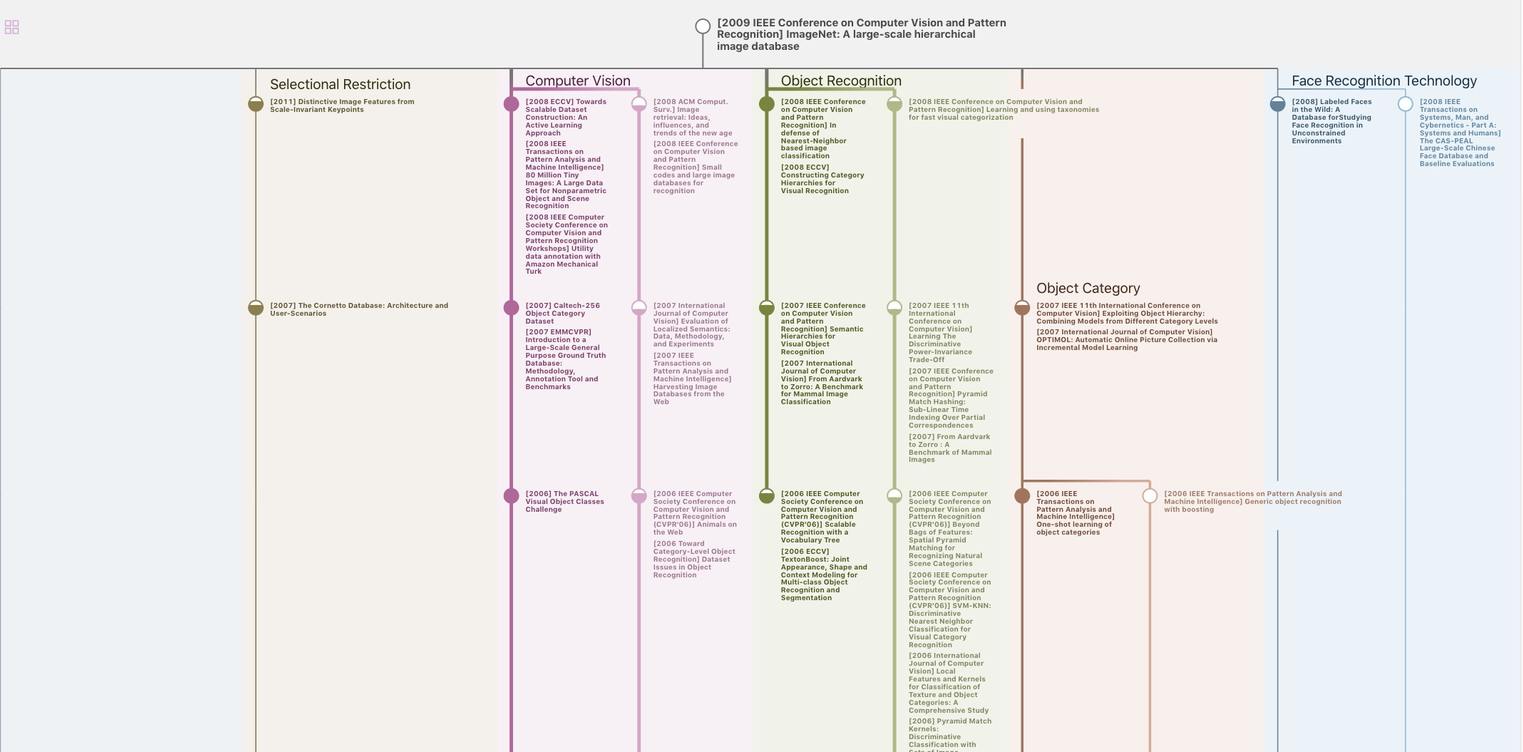
Generate MRT to find the research sequence of this paper
Chat Paper
Summary is being generated by the instructions you defined