Cold Posteriors through PAC-Bayes
ICLR 2023(2022)
摘要
We investigate the cold posterior effect through the lens of PAC-Bayes generalization bounds. We argue that in the non-asymptotic setting, when the number of training samples is (relatively) small, discussions of the cold posterior effect should take into account that approximate Bayesian inference does not readily provide guarantees of performance on out-of-sample data. Instead, out-of-sample error is better described through a generalization bound. In this context, we explore the connections between the ELBO objective from variational inference and the PAC-Bayes objectives. We note that, while the ELBO and PAC-Bayes objectives are similar, the latter objectives naturally contain a temperature parameter $\lambda$ which is not restricted to be $\lambda=1$. For both regression and classification tasks, in the case of isotropic Laplace approximations to the posterior, we show how this PAC-Bayesian interpretation of the temperature parameter captures the cold posterior effect.
更多查看译文
关键词
cold posteriors,Bayesian,Bayesian Neural Networks,PAC-Bayes,Laplace approximation
AI 理解论文
溯源树
样例
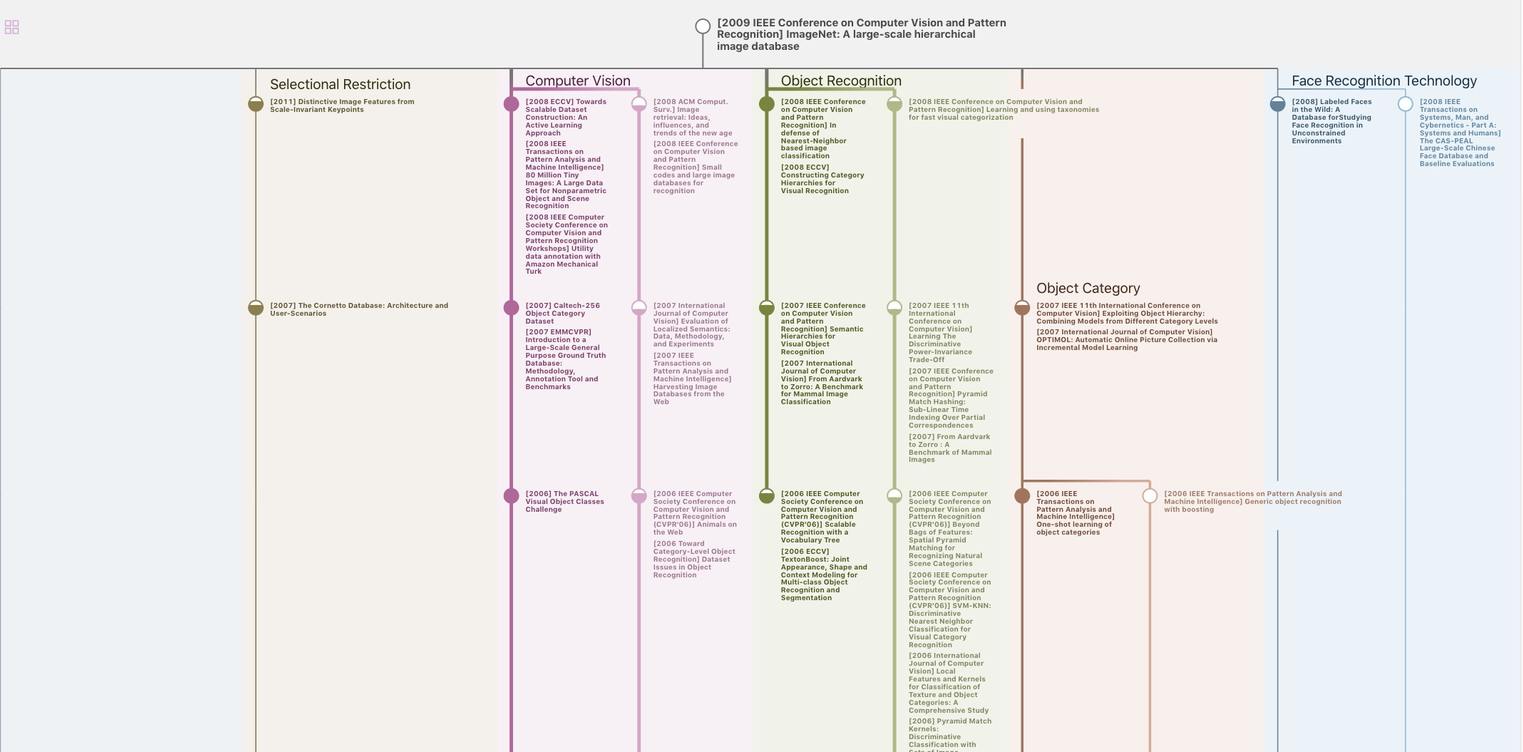
生成溯源树,研究论文发展脉络
Chat Paper
正在生成论文摘要