Jointist: Joint Learning for Multi-instrument Transcription and Its Applications
arxiv(2022)
摘要
In this paper, we introduce Jointist, an instrument-aware multi-instrument framework that is capable of transcribing, recognizing, and separating multiple musical instruments from an audio clip. Jointist consists of the instrument recognition module that conditions the other modules: the transcription module that outputs instrument-specific piano rolls, and the source separation module that utilizes instrument information and transcription results. The instrument conditioning is designed for an explicit multi-instrument functionality while the connection between the transcription and source separation modules is for better transcription performance. Our challenging problem formulation makes the model highly useful in the real world given that modern popular music typically consists of multiple instruments. However, its novelty necessitates a new perspective on how to evaluate such a model. During the experiment, we assess the model from various aspects, providing a new evaluation perspective for multi-instrument transcription. We also argue that transcription models can be utilized as a preprocessing module for other music analysis tasks. In the experiment on several downstream tasks, the symbolic representation provided by our transcription model turned out to be helpful to spectrograms in solving downbeat detection, chord recognition, and key estimation.
更多查看译文
关键词
jointist learning,multi-instrument
AI 理解论文
溯源树
样例
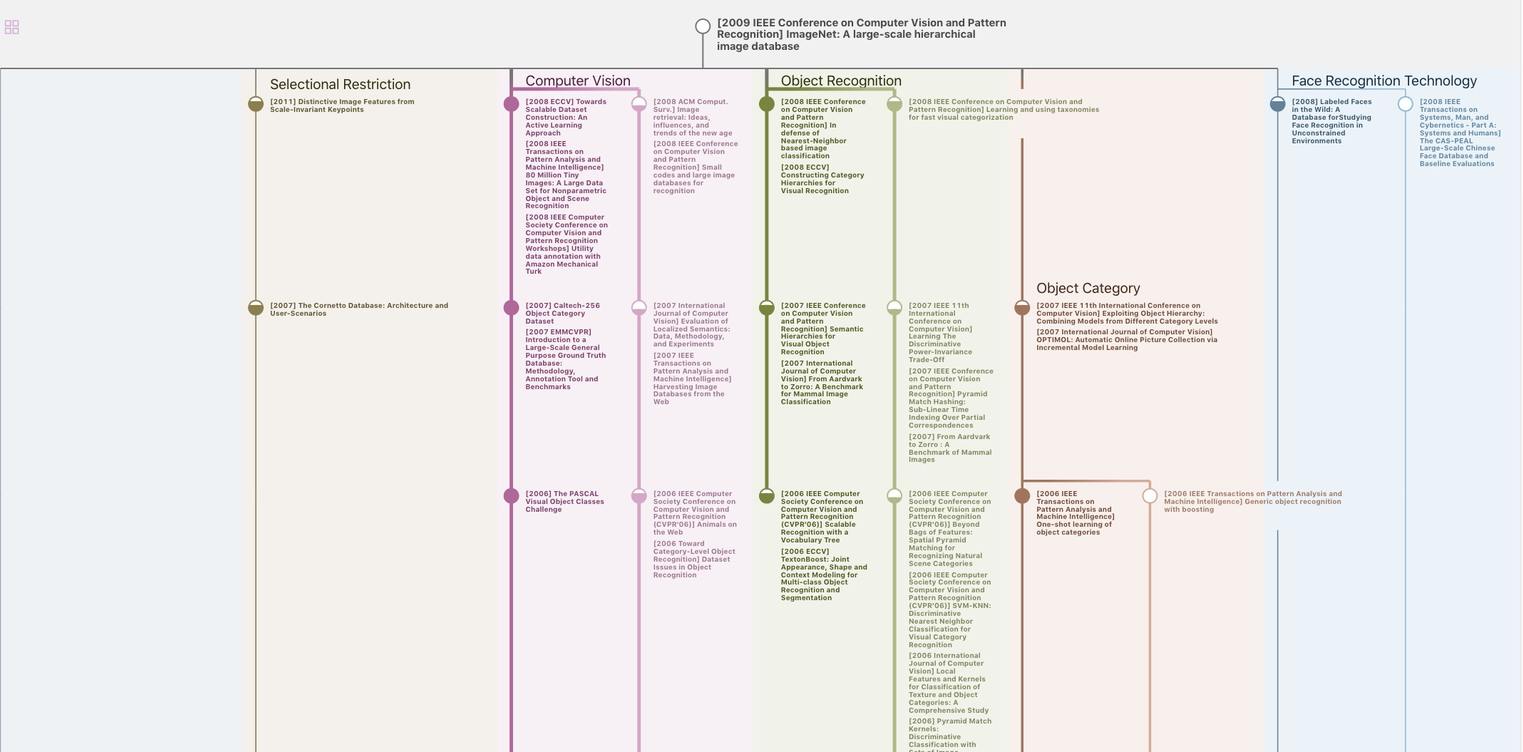
生成溯源树,研究论文发展脉络
Chat Paper
正在生成论文摘要