Wasserstein-Based Projections with Applications to Inverse Problems.
SIAM Journal on Mathematics of Data Science(2022)
摘要
Inverse problems consist of recovering a signal from a collection of noisy measurements. These are typically cast as optimization problems, with classic approaches using a data fidelity term and an analytic regularizer that stabilizes recovery. Recent plug-and-play (PnP) works propose replacing the operator for analytic regularization in optimization methods by a data-driven denoiser. These schemes obtain state-of-the-art results, but at the cost of limited theoretical guarantees. To bridge this gap, we present a new algorithm that takes samples from the manifold of true data as input and outputs an approximation of the projection operator onto this manifold. Under standard assumptions, we prove this algorithm generates a learned operator, called Wasserstein-based projection (WP), that approximates the true projection with high probability. Thus, WPs can be inserted into optimization methods in the same manner as PnP, but now with theoretical guarantees. Provided numerical examples show WPs obtain state-of-the-art results for unsupervised PnP signal recovery. All codes for this work can be found at https://github.com/swufung/WassersteinBasedProjections.
更多查看译文
关键词
inverse problems, generative adversarial network, generative modeling, deep neural network, Wasser-stein, projection, Halpern, plug-and-play, learning to optimize, computed tomography
AI 理解论文
溯源树
样例
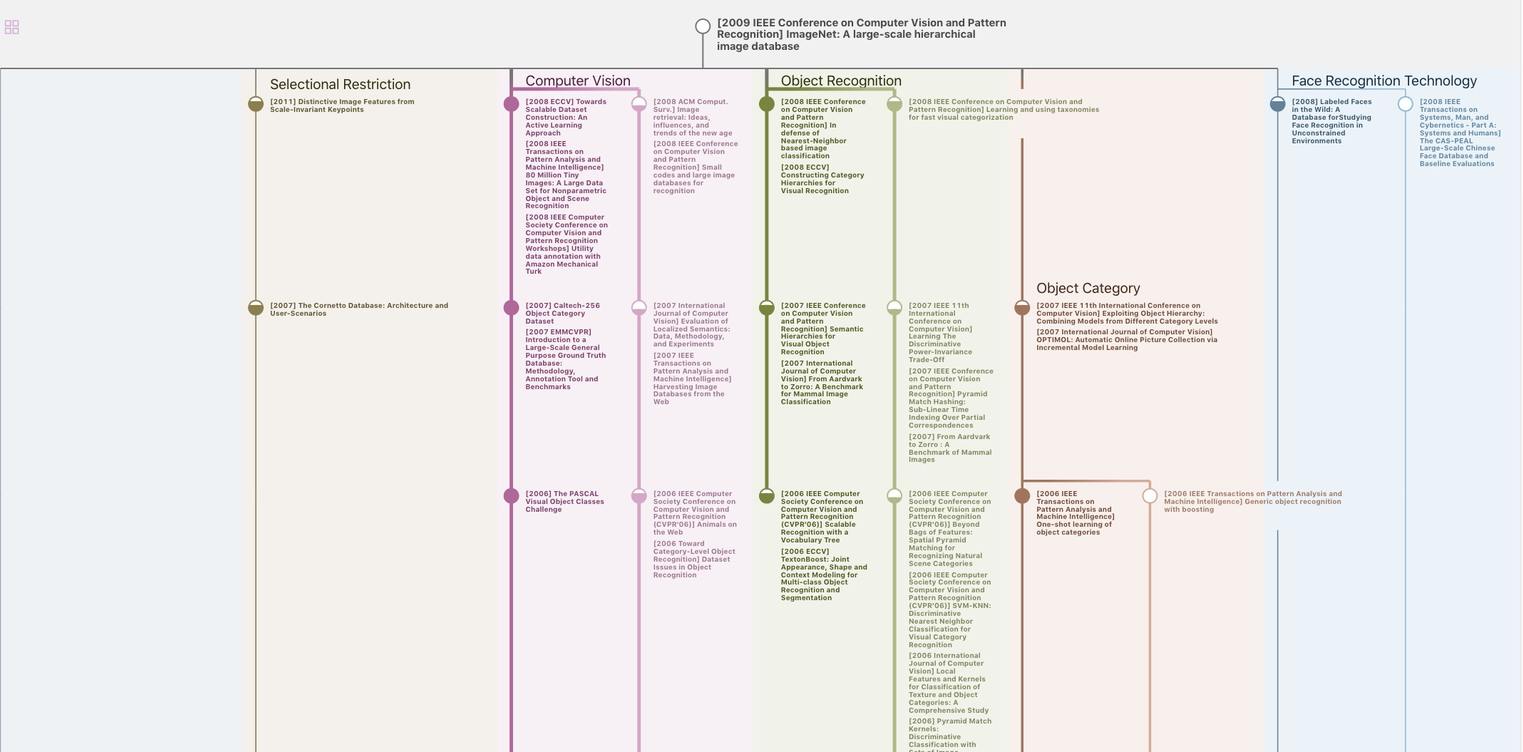
生成溯源树,研究论文发展脉络
Chat Paper
正在生成论文摘要