An Asymmetric Direct-Connected Network for Rolling Bearing Fault Diagnosis
2021 China Automation Congress (CAC)(2021)
摘要
Rolling bearings have be extensively applied in applications of intelligent manufacturing, such as rotating machinery, and the fault diagnosis of rolling bearings is critical to mitigate the production and economics losses. Deep learning, taking the advantage in signal processing, have been widely used in fault diagnosis. However, existing deep-learning-based fault diagnosis cannot be directly and easily applied to process the signal of rolling bearing. In addition, some existing scheme suffer from severe performance degradation in variable noisy environments or variable working loads. To address these issues, in this paper, an asymmetric direct-connected network, namely ADNet, is proposed to effectively diagnose faults of rolling bearing with deep learning technologies. To be specific, in our ADNet, the one-dimensional bearing signal firstly is converted into the recurrence plot (i.e., two-dimensional image), and multiple direct connection blocks (DCBs) that are nested with asymmetric convolution transformation (ACT) modules are proposed to extracted the detailed convolved feature map of faults. Finally, through the softmax classifier, the faults can be effectively identified by our ADNet based on the detailed convolved feature map of faults. Via evaluations, the data demonstrate that our ADNet can achieve great scalability and accuracy on faults diagnosis of rolling bearing in both variable noisy environments and variable working loads.
更多查看译文
关键词
Rolling bearings,deep learning,fault diagnosis,noisy environments,working loads
AI 理解论文
溯源树
样例
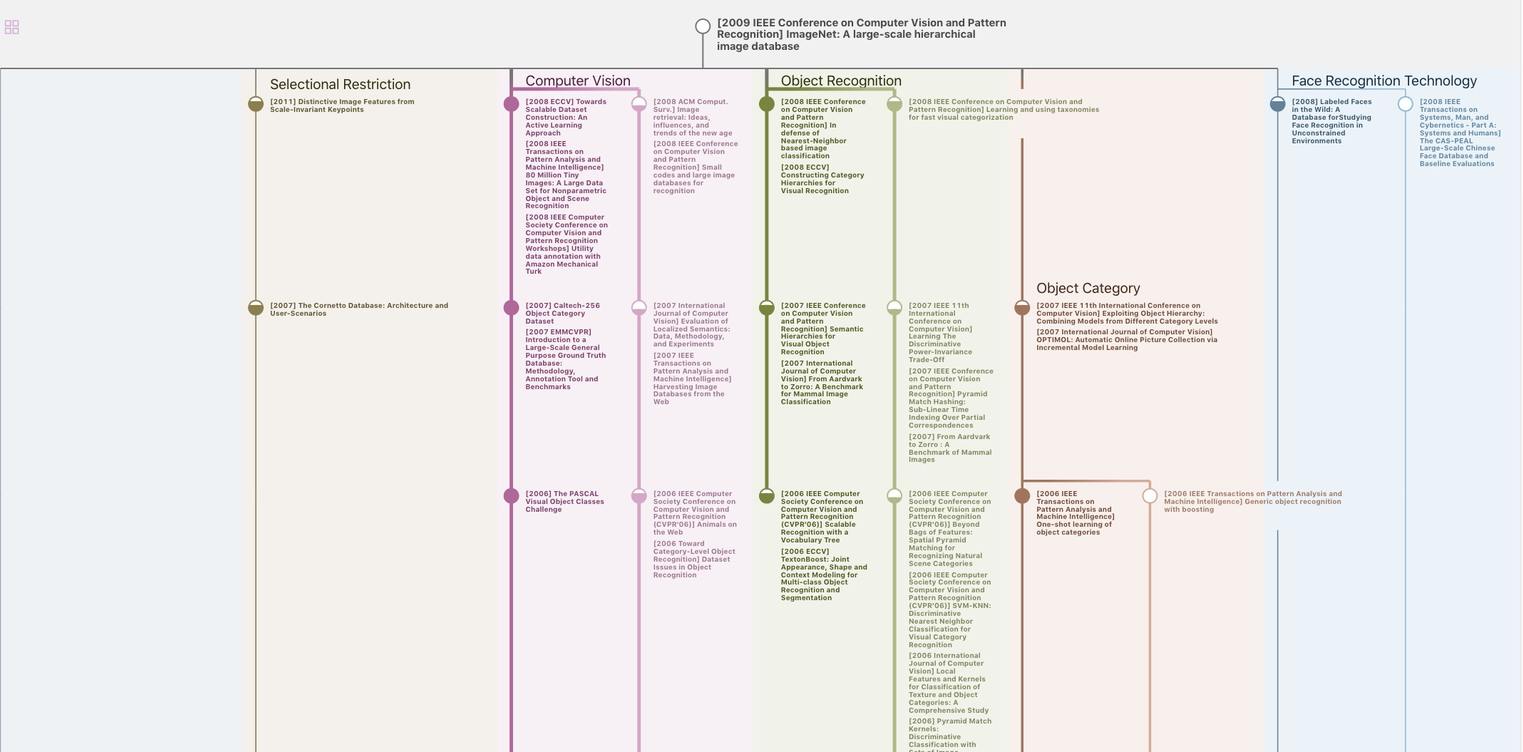
生成溯源树,研究论文发展脉络
Chat Paper
正在生成论文摘要