Time Optimal Trajectory Planning of Unmanned Surface Vessels: A Deep Learning-based Control Approach
2021 China Automation Congress (CAC)(2021)
Abstract
This paper presents a paradigm for Zermelo’s navigation problem (ZNP) of an unmanned vessel with the need of avoiding obstacles. The ZNP is first formulated as a typical optimal control problem (OCP) with continuous inequality constraints. In general, solving this typical OCP needs repeated offline optimization. On the one hand, a significant amount of time is consumed; nevertheless, once environmental influence creates a change in the system’s initial circumstances, it is required to re-optimize calculations. To overcome these drawbacks, a bi-level control paradigm based on deep neural network (DNN) is presented to achieve real-time optimal feedback control of the vessel in the presence of environmental uncertainty. Based on homotopy technology, we integrate the solution of OPC under the assumption of a limited range of current resistance as a training dataset. Due to the dataset incorporates all trajectory with different disturbance, once the DNN have been trained on a sufficient amount of dataset, the relationship between the optimal state variable and control instructs can be utilize as the optimal feedback control laws for realizing the real-time optimal control. The time-consuming re-planning process due to changes of initial conditions and the weakly robustness to control process in movement with environment disturbance can be effectively avoided in the paradigm. Numerical experimental results show the potential for real-time optimal control of more complex nonlinear models.
MoreTranslated text
Key words
Zermelo’ navigation problem,optimal control,deep learning,trajectory planning,Pseudospectral method
AI Read Science
Must-Reading Tree
Example
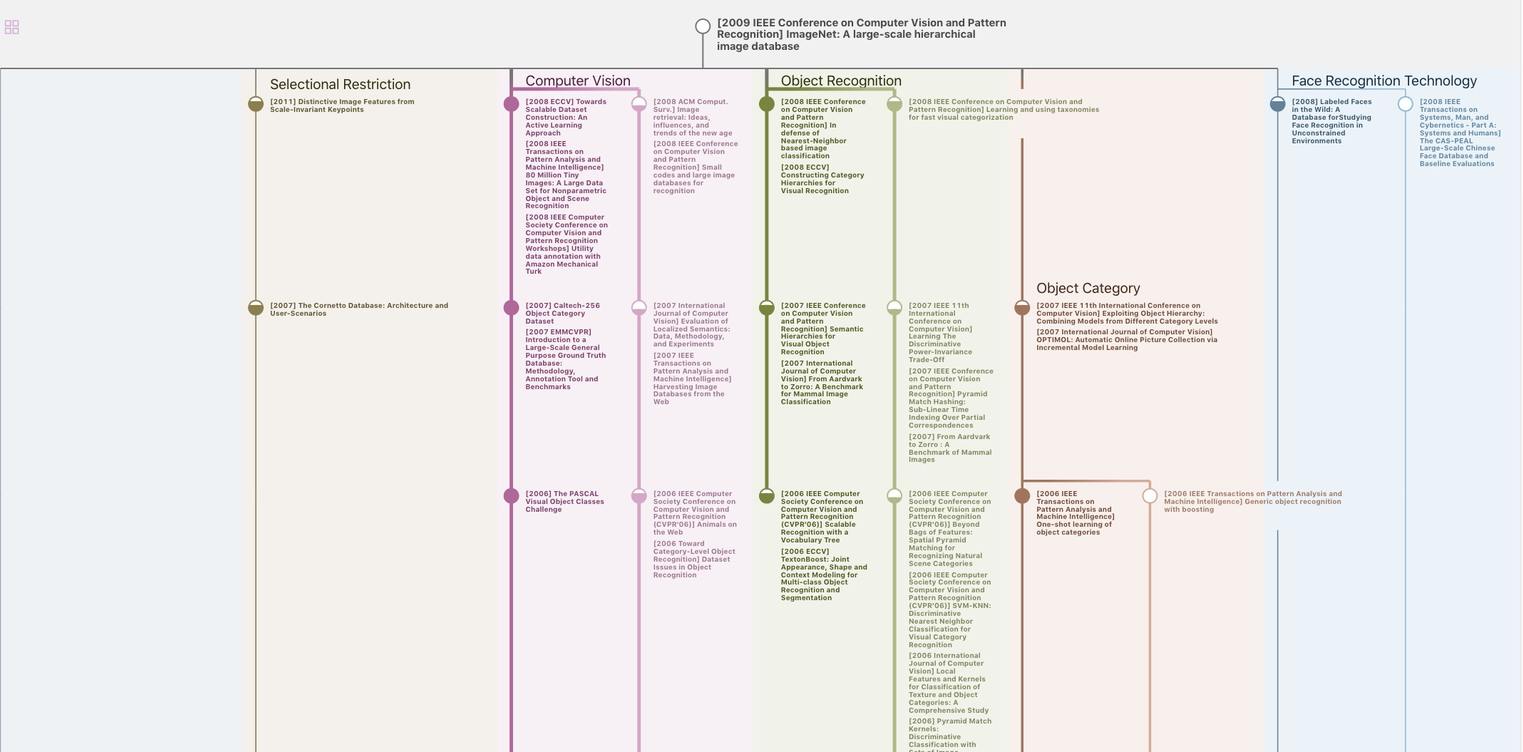
Generate MRT to find the research sequence of this paper
Chat Paper
Summary is being generated by the instructions you defined