Multi-Label Speech Emotion Recognition via Inter-Class Difference Loss Under Response Residual Network.
IEEE Transactions on Multimedia(2023)
摘要
Speech emotion recognition has always been a challenging task due to the difference in emotion expression and perception. Currently, in the supervised speech emotion recognition systems, the soft label overcomes the disadvantage of the hard label losing annotations variability and emotion perception subjectivity, but it only considers the emotion perceptions of a few annotators and thus still brings high statistical error. For this issue, this paper redefines the target and designs a novel loss function (denoted as inter-class difference loss), which enables the network to adaptively learn an emotion distribution in all utterances. This not only restricts the negative class probability less than the positive class probability, but also limits the negative class probability close to zero. To make the speech emotion recognition system more efficient, this paper proposes an end-to-end network, called response residual network (R-ResNet), which incorporates the ResNet for features extraction, together with the emotion response module for data augmentation and variable-length data processing. Finally, the experimental results not only demonstrate the advanced performance of our work, but also confirm that the ambiguous utterances contain emotional characteristics. In addition, another interesting finding is that, on the unbalanced dataset, the batch normalization (BN) after addition performs better than BN before addition.
更多查看译文
关键词
Emotion response, inter-class difference loss, multi-label, residual network (ResNet), speech emotion recognition
AI 理解论文
溯源树
样例
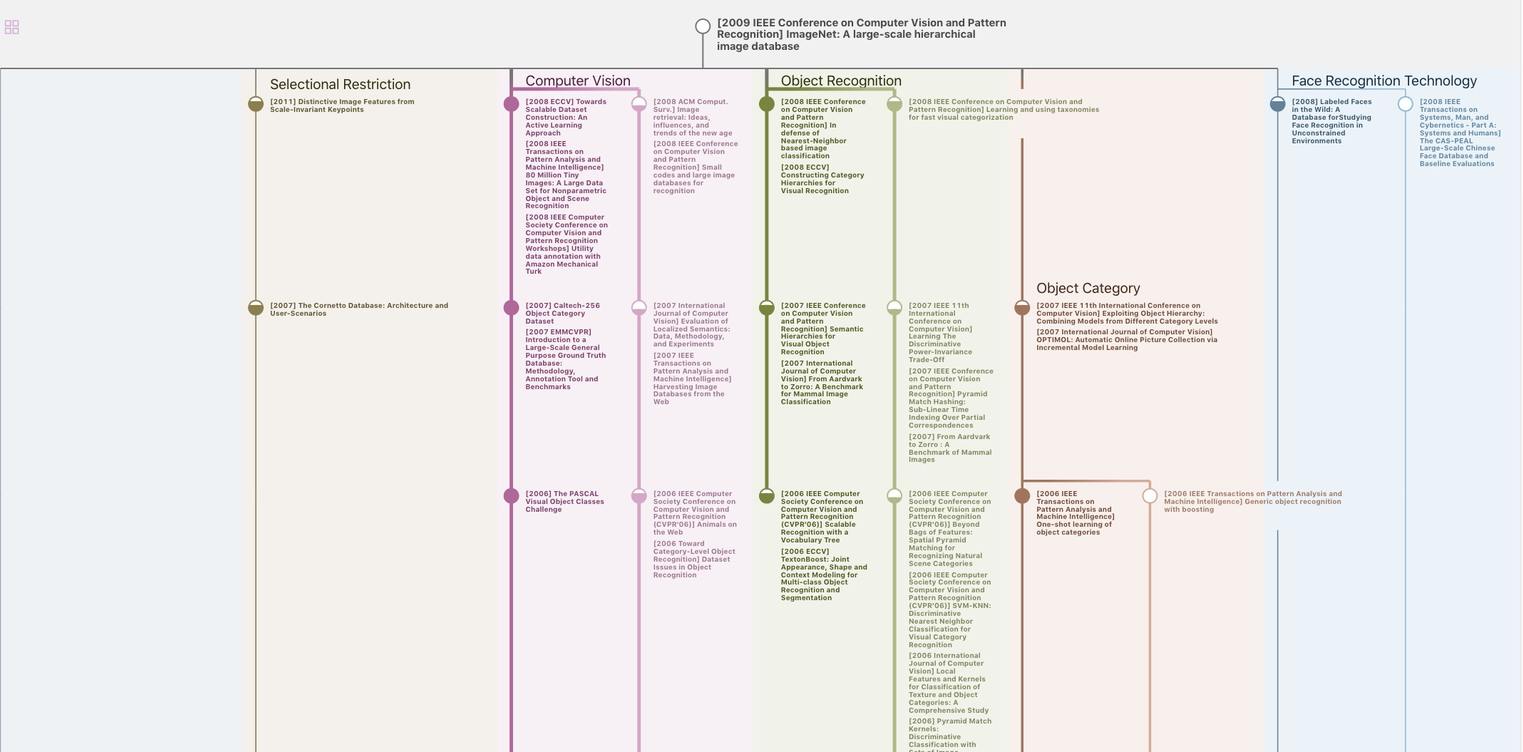
生成溯源树,研究论文发展脉络
Chat Paper
正在生成论文摘要