DFIL: an Efficient Web Service Prediction Method based on Deep Feature Interactive Learning
2022 IEEE 25th International Conference on Computer Supported Cooperative Work in Design (CSCWD)(2022)
摘要
As a representative of nonfunctional features, the Quality-of-Service (QoS) plays an important role in recommending the best service to users. To obtain the QoS value of web services, researchers have proposed many web service QoS prediction methods. However, most existing methods, e.g., the collaborative filtering method, leverage the historical user service invocation information to predict the QoS value, and only consider the QoS information of similar users or services, and ignore the attributes and characteristics of users and services. In this paper, we propose a Deep Feature Interaction Learning method, called DFIL for short, for web service QoS prediction. Specifically, (1) DFIL constructs a feature extraction method to effectively obtain the features of users and services. (2) DFIL trains a novel neural network for QoS prediction, which can accurately find the potential relationship between users and services based on the feature information, and provide good web service recommendation.
更多查看译文
关键词
Web Service,QoS,Data Sparsity,Cold Start,Deep Feature Interaction Learning
AI 理解论文
溯源树
样例
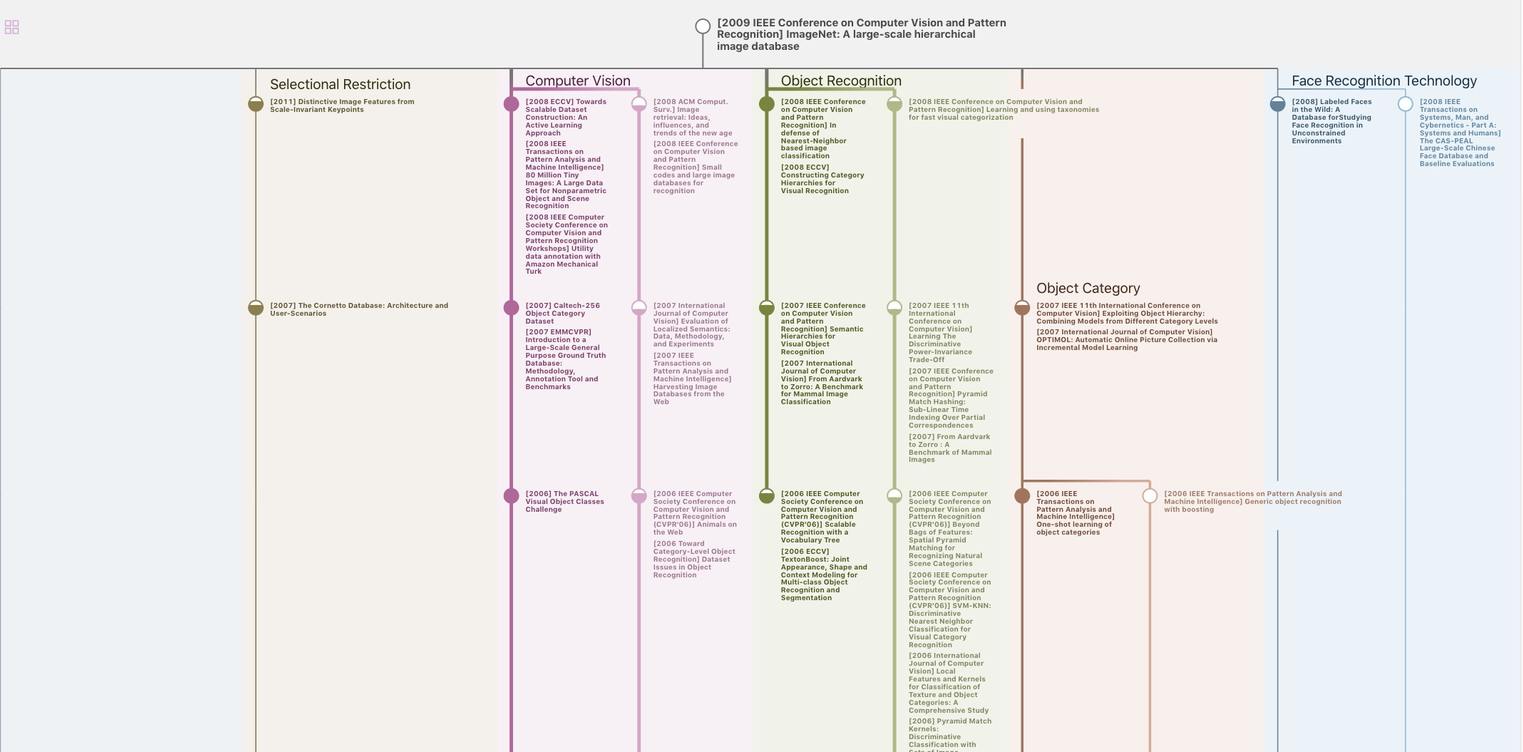
生成溯源树,研究论文发展脉络
Chat Paper
正在生成论文摘要