Multi-model forecast quality assessment of CMIP6 decadal predictions
Journal of Climate(2022)
Abstract
Abstract Decadal climate predictions are a relatively new source of climate information for inter-annual to decadal time scales, which is of increasing interest for users. Forecast quality assessment is essential to identify windows of opportunity (e.g., variables, regions, and forecast periods) with skill that can be used to develop climate services to inform users in several sectors and define benchmarks for improvements in forecast systems. This work evaluates the quality of multi-model forecasts of near-surface air temperature, precipitation, Atlantic multi-decadal variability index (AMV) and global near-surface air temperature anomalies (GSAT) generated from all the available retrospective decadal predictions contributing to the Coupled Model Intercomparison Project Phase 6 (CMIP6). The predictions generally show high skill in predicting temperature, AMV, and GSAT, while the skill is more limited for precipitation. Different approaches for generating a multi-model forecast are compared, finding small differences between them. The multi-model ensemble is also compared to the individual forecast systems. The best system usually provides the highest skill. However, the multi-model ensemble is a reasonable choice for not having to select the best system for each particular variable, forecast period and region. Furthermore, the decadal predictions are compared to the historical simulations to estimate the impact of initialization. An added value is found for several ocean and land regions for temperature, AMV, and GSAT, while it is more reduced for precipitation. Moreover, the full ensemble is compared to a sub-ensemble to measure the impact of the ensemble size. Finally, the implications of these results in a climate services context, which requires predictions issued in near real-time, are discussed.
MoreTranslated text
Key words
Climate prediction,Ensembles,Forecast verification/skill,Hindcasts,Probability forecasts/models/,distribution,Decadal variability,Climate services
AI Read Science
Must-Reading Tree
Example
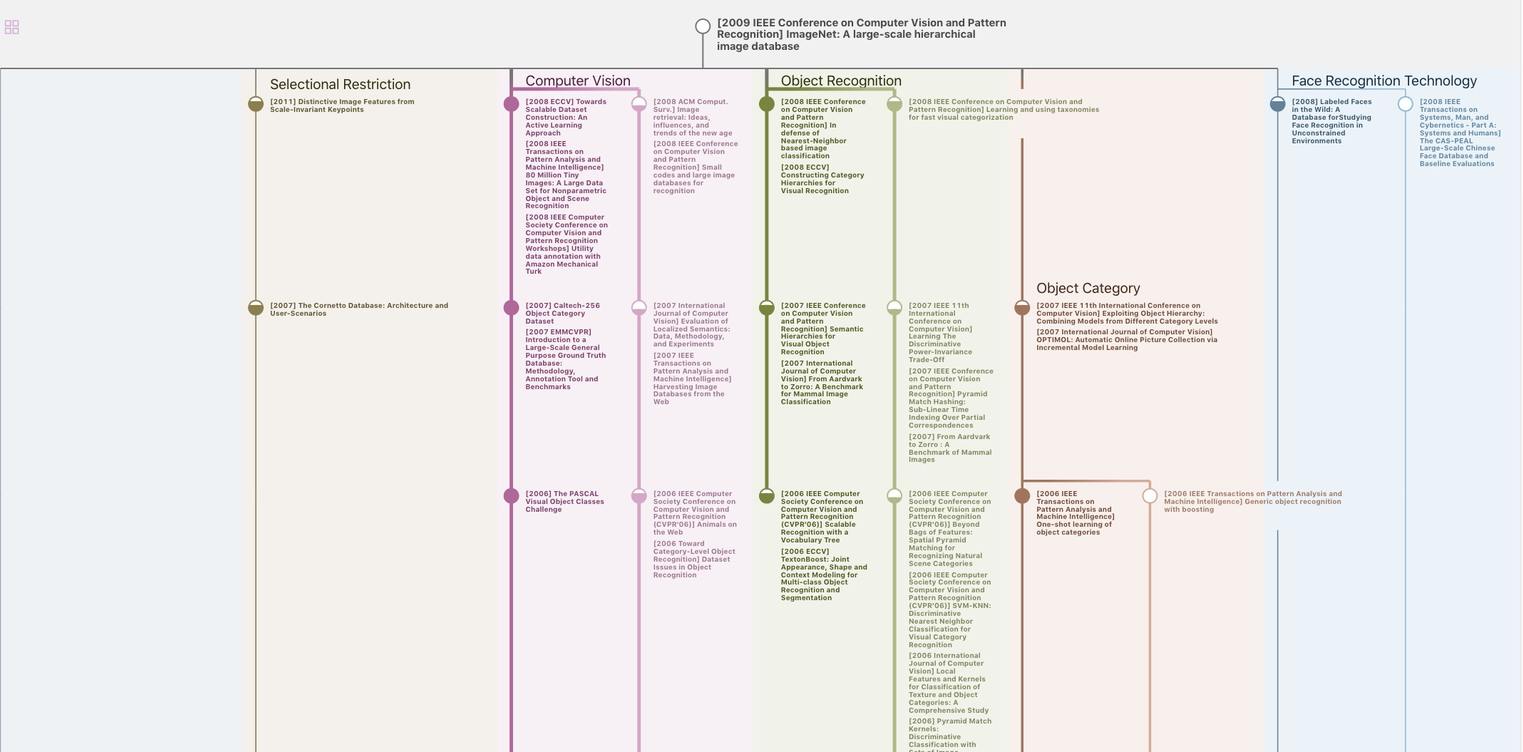
Generate MRT to find the research sequence of this paper
Chat Paper
Summary is being generated by the instructions you defined