Neural circuit mechanisms of hierarchical sequence learning tested on large-scale recording data
PLOS COMPUTATIONAL BIOLOGY(2022)
Abstract
The brain performs various cognitive functions by learning the spatiotemporal salient features of the environment. This learning requires unsupervised segmentation of hierarchically organized spike sequences, but the underlying neural mechanism is only poorly understood. Here, we show that a recurrent gated network of neurons with dendrites can efficiently solve difficult segmentation tasks. In this model, multiplicative recurrent connections learn a context-dependent gating of dendro-somatic information transfers to minimize error in the prediction of somatic responses by the dendrites. Consequently, these connections filter the redundant input features represented by the dendrites but unnecessary in the given context. The model was tested on both synthetic and real neural data. In particular, the model was successful for segmenting multiple cell assemblies repeating in large-scale calcium imaging data containing thousands of cortical neurons. Our results suggest that recurrent gating of dendro-somatic signal transfers is crucial for cortical learning of context-dependent segmentation tasks. Author summaryThe brain learns about the environment from continuous streams of information to generate adequate behavior. This is not easy when sensory and motor sequences are hierarchically organized. Some cortical regions jointly represent multiple levels of sequence hierarchy, but how local cortical circuits learn hierarchical sequences remains largely unknown. Evidence shows that the dendrites of cortical neurons learn redundant representations of sensory information compared to the soma, suggesting a filtering process within a neuron. Our model proposes that recurrent synaptic inputs multiplicatively regulate this intracellular process by gating dendrite-to-soma information transfers depending on the context of sequence learning. Furthermore, our model provides a powerful tool to analyze the spatiotemporal patterns of neural activity in large-scale recording data.
MoreTranslated text
AI Read Science
Must-Reading Tree
Example
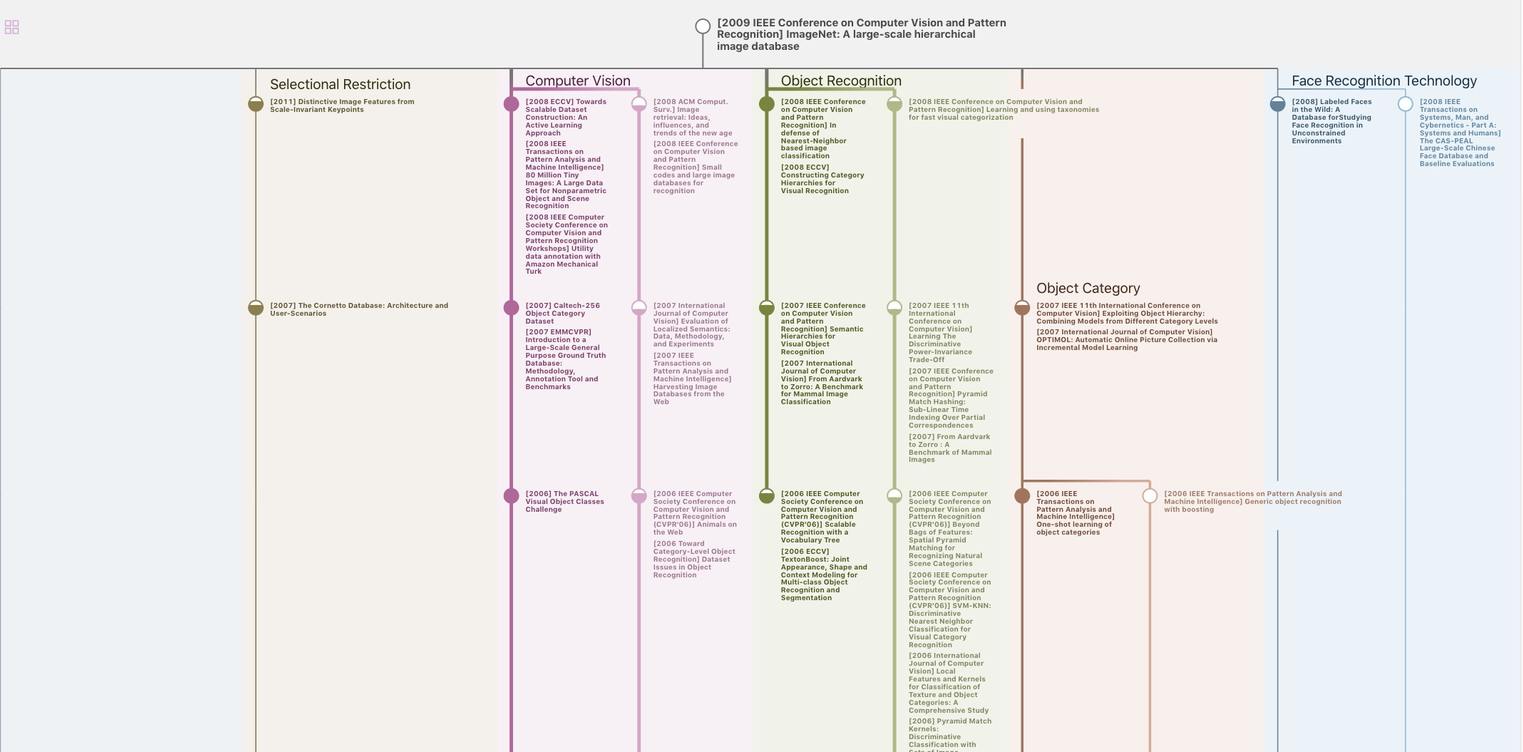
Generate MRT to find the research sequence of this paper
Chat Paper
Summary is being generated by the instructions you defined