Residual-recursive autoencoder for accelerated evolution in savonius wind turbines optimization
Neurocomputing(2022)
摘要
Recent studies verified that a genetic algorithm can discover efficient and innovative wind turbines by using image encoding and decoding techniques. To accelerate the optimization, in this work, Residual-Recursion Autoencoder (RRAE) is proposed to extract low-dimensional latent codes from rotors’ cross-section images while maintaining reconstruction accuracy as high as possible. As a kind of neural network framework, the advantages of using RRAE are threefold: 1) RRAE can wrap over different kinds of autoencoders and improve their performance; 2) RRAE is compatible with different kinds of loss functions and works well with very low-dimensional latent codes; 3) RRAE is easy to use and efficient in decoding latent codes which is important to the rapid convergence of the genetic algorithm. The experiment results has shown that the reconstruction loss has decreased by 30.56% on a recursive autoencoder, 11.40% to 29.34% on different feedforward autoencoders. Two RRAE-accelerated optimizations have been carried out in this work. One has used only 14% of the calculation required by the baseline method without any deterioration in rotor performance. The other one has used 52.33% and increased the rotor performance by 7.59%.
更多查看译文
关键词
Recursive neural network,Autoencoder,Deep learning,Geometry optimization,Savonius rotor
AI 理解论文
溯源树
样例
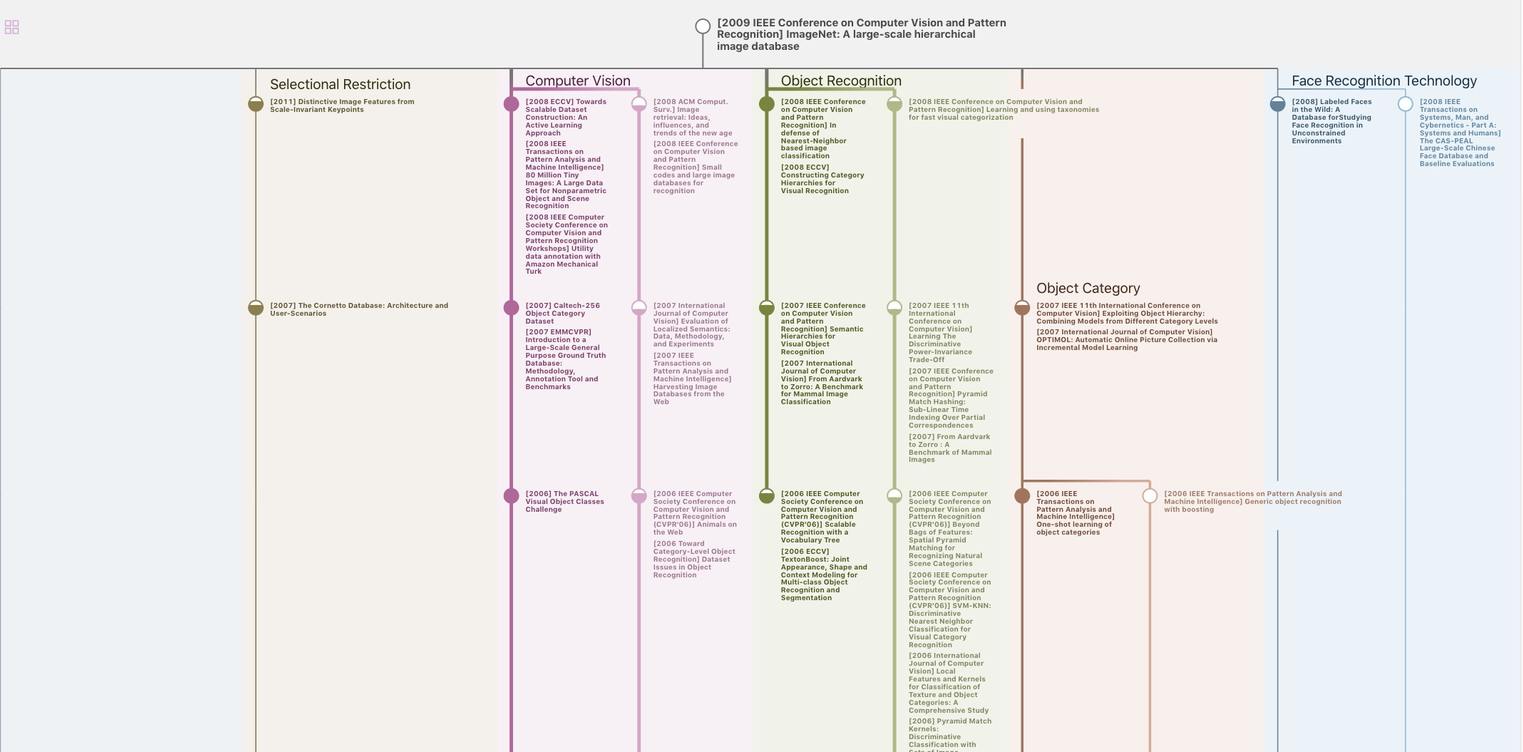
生成溯源树,研究论文发展脉络
Chat Paper
正在生成论文摘要