On the effectiveness of persistent homology
NeurIPS 2022(2022)
摘要
Persistent homology (PH) is one of the most popular methods in Topological Data Analysis. Even though PH has been used in many different types of applications, the reasons behind its success remain elusive; in particular, it is not known for which classes of problems it is most effective, or to what extent it can detect geometric or topological features. The goal of this work is to identify some types of problems where PH performs well or even better than other methods in data analysis. We consider three fundamental shape analysis tasks: the detection of the number of holes, curvature and convexity from 2D and 3D point clouds sampled from shapes. Experiments demonstrate that PH is successful in these tasks, outperforming several baselines, including PointNet, an architecture inspired precisely by the properties of point clouds. In addition, we observe that PH remains effective for limited computational resources and limited training data, as well as out-of-distribution test data, including various data transformations and noise. For convexity detection, we provide a theoretical guarantee that PH is effective for this task, and demonstrate the detection of a convexity measure on the FLAVIA dataset of plant leaf images.
更多查看译文
关键词
persistent homology,Betti numbers,curvature,convexity,topology of data,geometry of data,point clouds
AI 理解论文
溯源树
样例
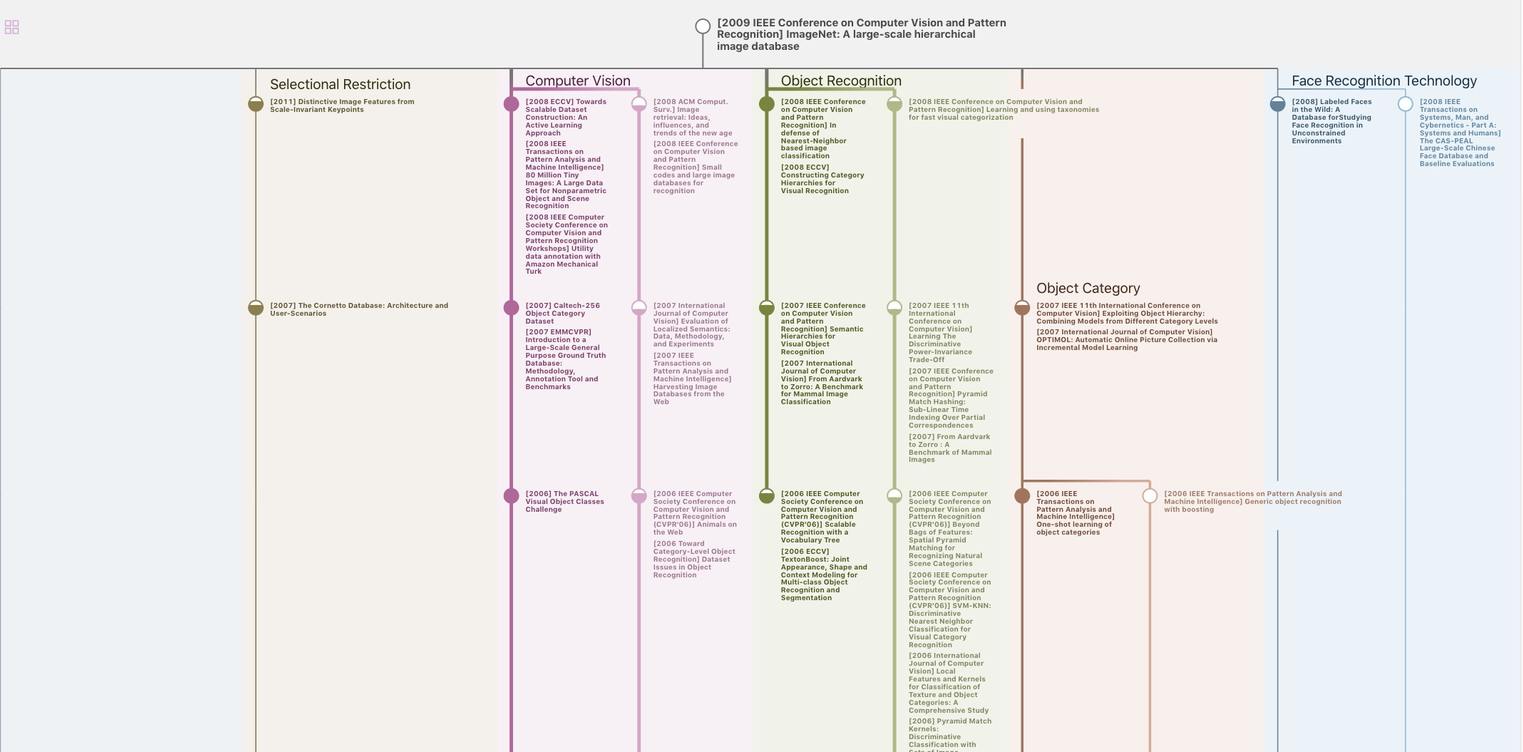
生成溯源树,研究论文发展脉络
Chat Paper
正在生成论文摘要